Modular Neural Ordinary Differential Equations
arxiv(2021)
摘要
The laws of physics have been written in the language of dif-ferential equations for centuries. Neural Ordinary Differen-tial Equations (NODEs) are a new machine learning architecture which allows these differential equations to be learned from a dataset. These have been applied to classical dynamics simulations in the form of Lagrangian Neural Net-works (LNNs) and Second Order Neural Differential Equations (SONODEs). However, they either cannot represent the most general equations of motion or lack interpretability. In this paper, we propose Modular Neural ODEs, where each force component is learned with separate modules. We show how physical priors can be easily incorporated into these models. Through a number of experiments, we demonstrate these result in better performance, are more interpretable, and add flexibility due to their modularity.
更多查看译文
关键词
ordinary differential equations
AI 理解论文
溯源树
样例
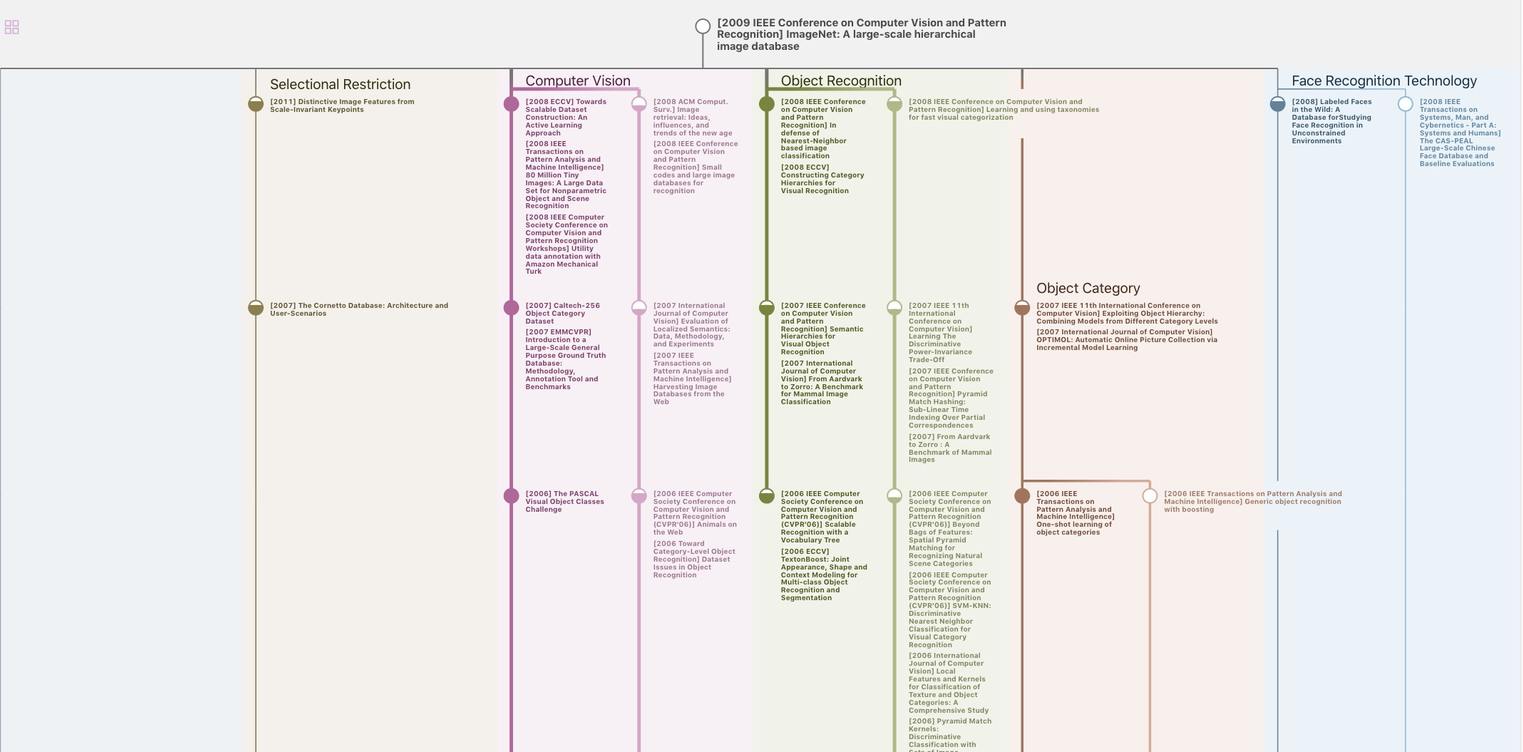
生成溯源树,研究论文发展脉络
Chat Paper
正在生成论文摘要