Inequality Constrained Trajectory Optimization with A Hybrid Multiple-shooting iLQR
arxiv(2023)
摘要
Trajectory optimization has been used extensively in robotic systems. In particular, iterative Linear Quadratic Regulator (iLQR) has performed well as an off-line planner and online nonlinear model predictive control solver, with a lower computational cost. However, standard iLQR cannot handle any constraints or perform reasonable initialization of a state trajectory. In this paper, we propose a hybrid constrained iLQR variant with a multiple-shooting framework to incorporate general inequality constraints and infeasible states initialization. The main technical contributions are twofold: 1) In addition to inheriting the simplicity of the initialization in multiple-shooting settings, a two-stage framework is developed to deal with state and/or control constraints robustly without loss of the linear feedback term of iLQR. Such a hybrid strategy offers fast convergence of constraint satisfaction. 2) An improved globalization strategy is proposed to exploit the coupled effects between line-searching and regularization, which is able to enhance the numerical robustness of the constrained iLQR approaches. Our approach is tested on various constrained trajectory optimization problems and outperforms the commonly-used collocation and shooting methods.
更多查看译文
关键词
optimization,multiple-shooting
AI 理解论文
溯源树
样例
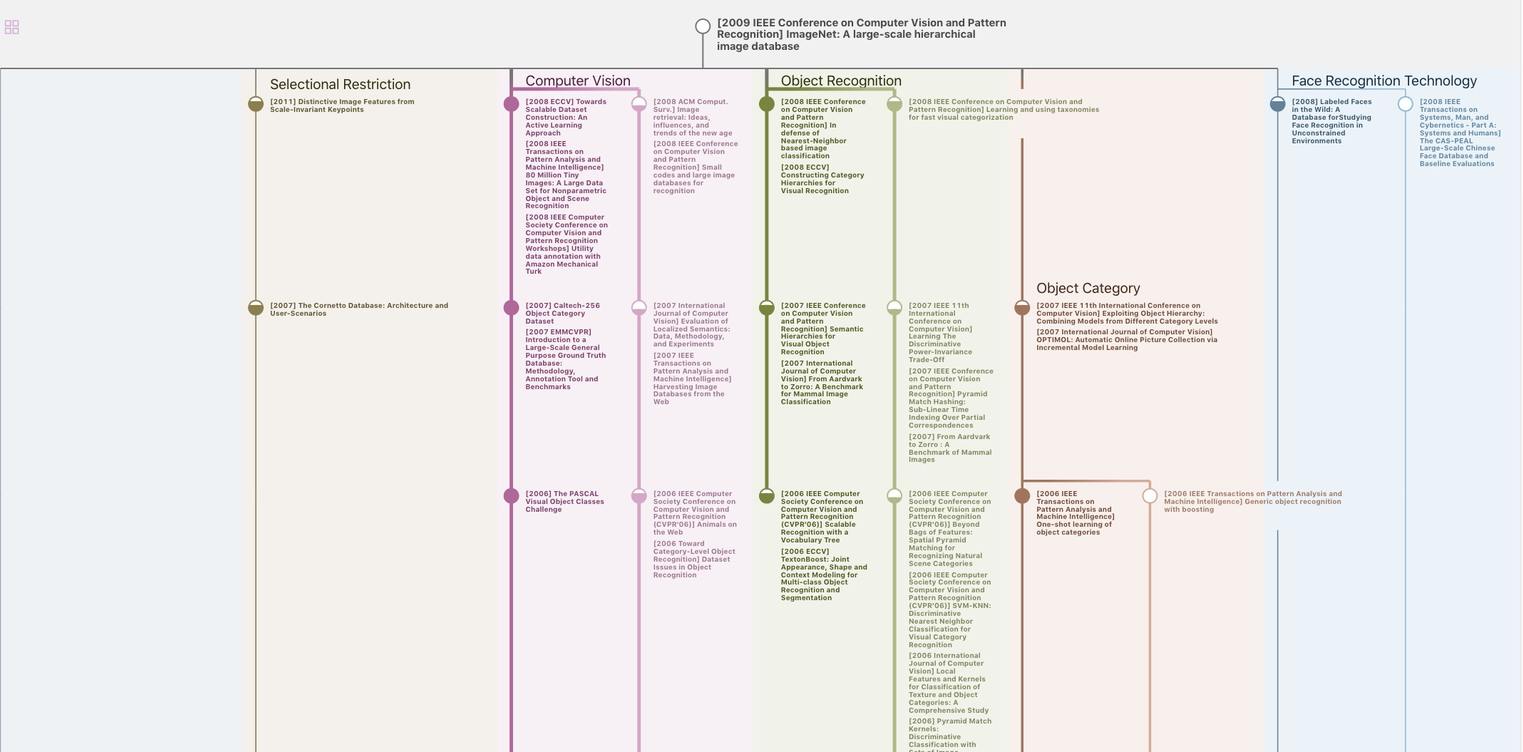
生成溯源树,研究论文发展脉络
Chat Paper
正在生成论文摘要