Fast Extraction of Word Embedding from Q-contexts
Conference on Information and Knowledge Management(2021)
摘要
ABSTRACTThe notion of word embedding plays a fundamental role in natural language processing (NLP). However, pre-training word embedding for very large-scale vocabulary is computationally challenging for most existing methods. In this work, we show that with merely a small fraction of contexts (Q-contexts) which are typical in the whole corpus (and their mutual information with words), one can construct high-quality word embedding with negligible errors. Mutual information between contexts and words can be encoded canonically as a sampling state, thus, Q-contexts can be fast constructed. Furthermore, we present an efficient and effective WEQ method, which is capable of extracting word embedding directly from these typical contexts. In practical scenarios, our algorithm runs 11 ~ 13 times faster than well-established methods. By comparing with well-known methods such as matrix factorization, word2vec, GloVe and fasttext, we demonstrate that our method achieves comparable performance on a variety of downstream NLP tasks, and in the meanwhile maintains run-time and resource advantages over all these baselines.
更多查看译文
AI 理解论文
溯源树
样例
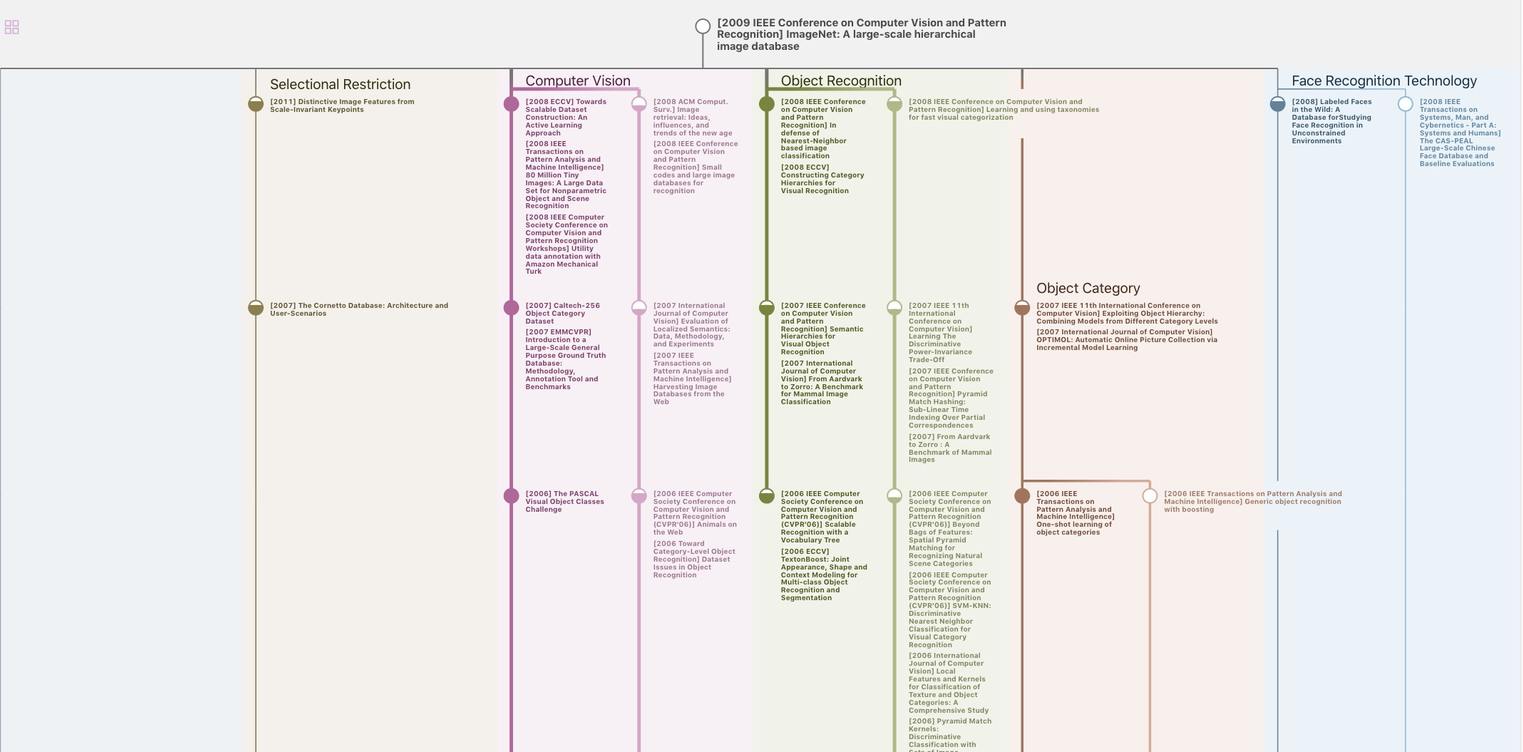
生成溯源树,研究论文发展脉络
Chat Paper
正在生成论文摘要