Learning Density Distribution of Reachable States for Autonomous Systems.
CoRL(2021)
摘要
State density distribution, in contrast to worst-case reachability, can be leveraged for safety-related problems to better quantify the likelihood of the risk for potentially hazardous situations. In this work, we propose a data-driven method to compute the density distribution of reachable states for nonlinear and even black-box systems. Our semi-supervised approach learns system dynamics and the state density jointly from trajectory data, guided by the fact that the state density evolution follows the Liouville partial differential equation. With the help of neural network reachability tools, our approach can estimate the set of all possible future states as well as their density. Moreover, we could perform online safety verification with probability ranges for unsafe behaviors to occur. We use an extensive set of experiments to show that our learned solution can produce a much more accurate estimate on density distribution, and can quantify risks less conservatively and flexibly comparing with worst-case analysis.
更多查看译文
关键词
reachable states,density distribution,learning
AI 理解论文
溯源树
样例
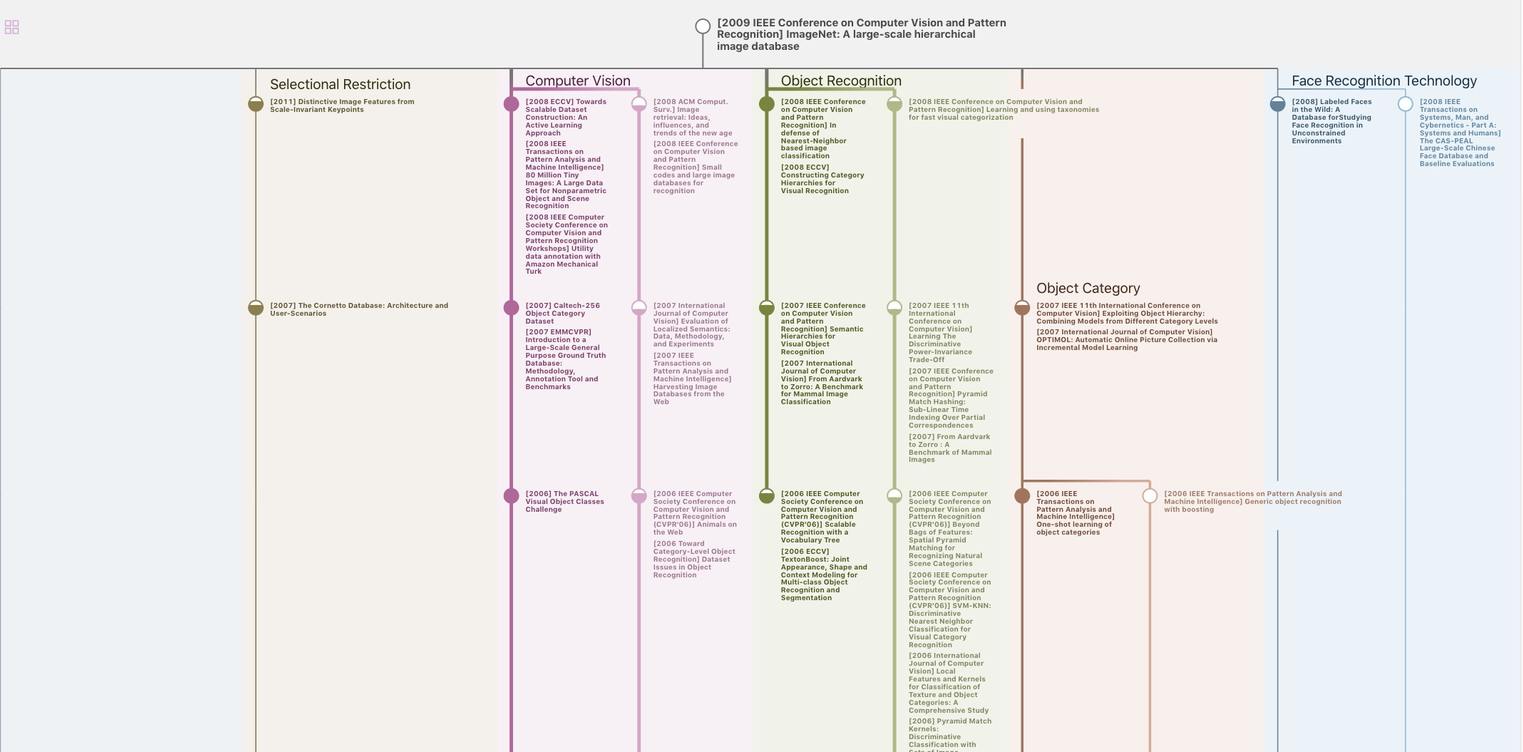
生成溯源树,研究论文发展脉络
Chat Paper
正在生成论文摘要