GPGM-SLAM: a Robust SLAM System for Unstructured Planetary Environments with Gaussian Process Gradient Maps
arxiv(2021)
摘要
Simultaneous Localization and Mapping (SLAM) techniques play a key role towards long-term autonomy of mobile robots due to the ability to correct localization errors and produce consistent maps of an environment over time. Contrarily to urban or man-made environments, where the presence of unique objects and structures offer unique cues for localization, the appearance of unstructured natural environments is often ambiguous and self-similar, hindering the performances of loop closure detection. In this paper, we present an approach to improve the robustness of place recognition in the context of a submap-based stereo SLAM based on Gaussian Process Gradient Maps (GPGMaps). GPGMaps embed a continuous representation of the gradients of the local terrain elevation by means of Gaussian Process regression and Structured Kernel Interpolation, given solely noisy elevation measurements. We leverage the image-like structure of GPGMaps to detect loop closures using traditional visual features and Bag of Words. GPGMap matching is performed as an SE(2) alignment to establish loop closure constraints within a pose graph. We evaluate the proposed pipeline on a variety of datasets recorded on Mt. Etna, Sicily and in the Morocco desert, respectively Moon- and Mars-like environments, and we compare the localization performances with state-of-the-art approaches for visual SLAM and visual loop closure detection.
更多查看译文
关键词
robust gpgm-slam system,unstructured planetary environments,maps
AI 理解论文
溯源树
样例
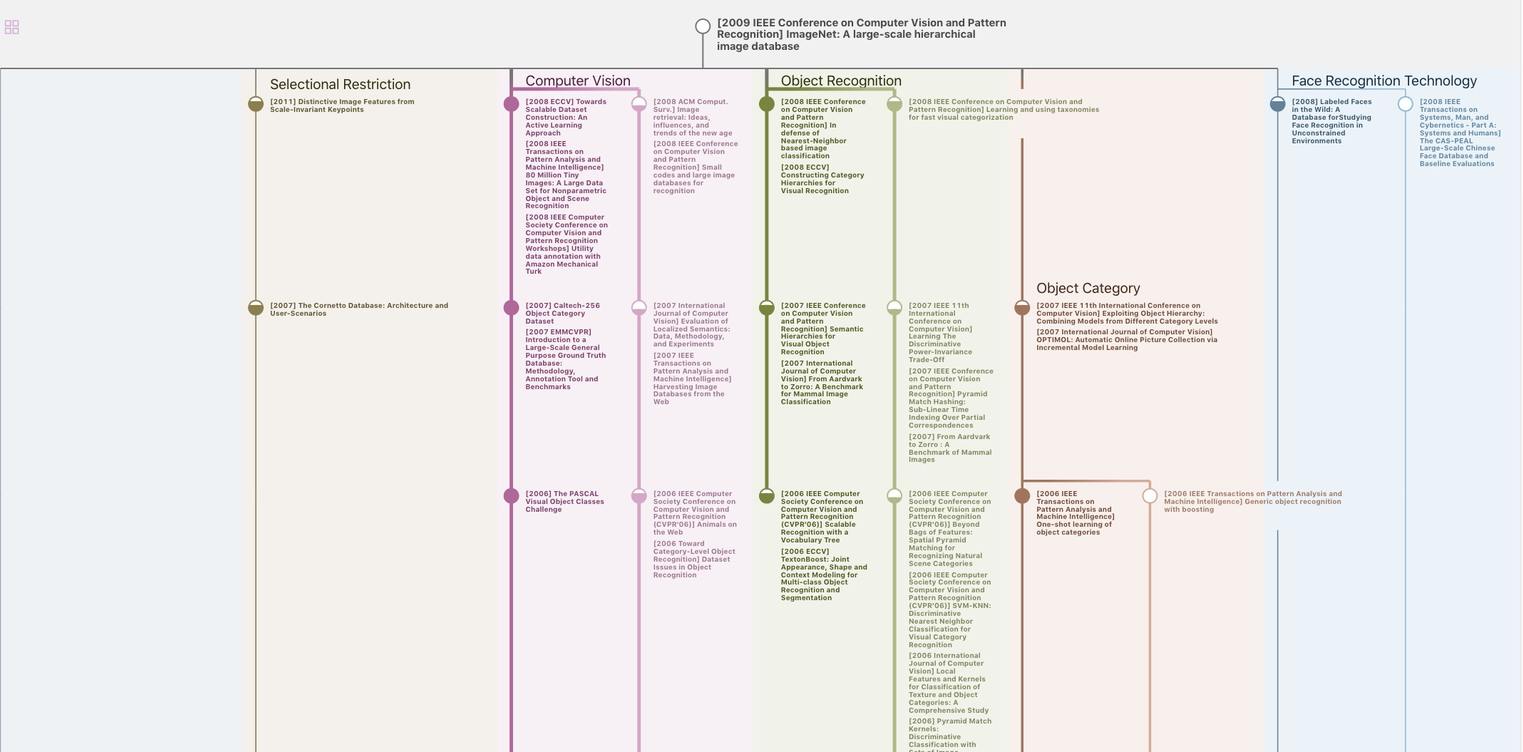
生成溯源树,研究论文发展脉络
Chat Paper
正在生成论文摘要