Street-level Air Quality Inference Based on Geographically Context-aware Random Forest Using Opportunistic Mobile Sensor Network
2021 5TH INTERNATIONAL CONFERENCE ON INNOVATION IN ARTIFICIAL INTELLIGENCE (ICIAI 2021)(2021)
摘要
The spatial heterogeneity and temporal variability of air pollution in urban environments make air quality inference for fine-grained air pollution monitoring extremely challenging. Most of the existing work estimates the air quality using sparse measurements collected from a limited number of fixed monitoring stations or make use of computationally demanding physicochemical models simulating the source and fate of pollutants across multiple spatial scales. In this work, we propose a geographically context-aware random forest model for street-level air quality inference using high spatial resolution data collected by an opportunistic mobile sensor network. Compared with a traditional random forest model, the proposed method builds a local model for each location by considering the neighbors in both geographical and feature space. The model is evaluated on our real air quality dataset collected from mobile sensors in Antwerp, Belgium. The experimental results show that the proposed method outperforms a series of commonly used methods including Ordinary Kriging (OK), Inverse Distance Weighting (IDW) and Random forest (RF).
更多查看译文
关键词
Air quality inference, Internet of Things (IoT), Smart city, Machine learning
AI 理解论文
溯源树
样例
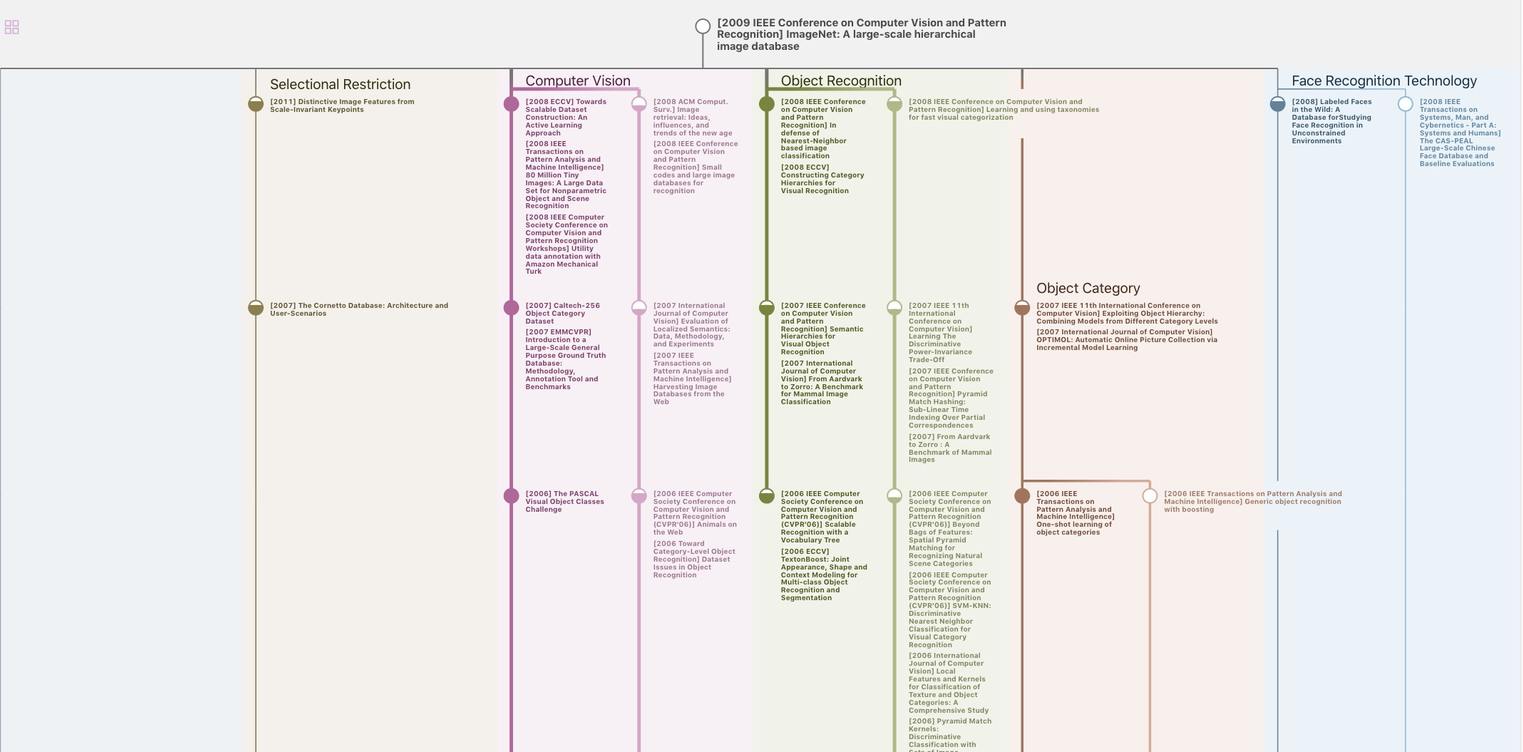
生成溯源树,研究论文发展脉络
Chat Paper
正在生成论文摘要