Phase-Property Diagrams for Multicomponent Oxide Systems toward Materials Libraries
ADVANCED MATERIALS(2021)
摘要
Exploring the vast compositional space offered by multicomponent systems or high entropy materials using the traditional route of materials discovery, one experiment at a time, is prohibitive in terms of cost and required time. Consequently, the development of high-throughput experimental methods, aided by machine learning and theoretical predictions will facilitate the search for multicomponent materials in their compositional variety. In this study, high entropy oxides are fabricated and characterized using automated high-throughput techniques. For intuitive visualization, a graphical phase-property diagram correlating the crystal structure, the chemical composition, and the band gap are introduced. Interpretable machine learning models are trained for automated data analysis and to speed up data comprehension. The establishment of materials libraries of multicomponent systems correlated with their properties (as in the present work), together with machine learning-based data analysis and theoretical approaches are opening pathways toward virtual development of novel materials for both functional and structural applications.
更多查看译文
关键词
high entropy materials, high-throughput techniques, machine learning, materials libraries, phase diagram, virtual materials
AI 理解论文
溯源树
样例
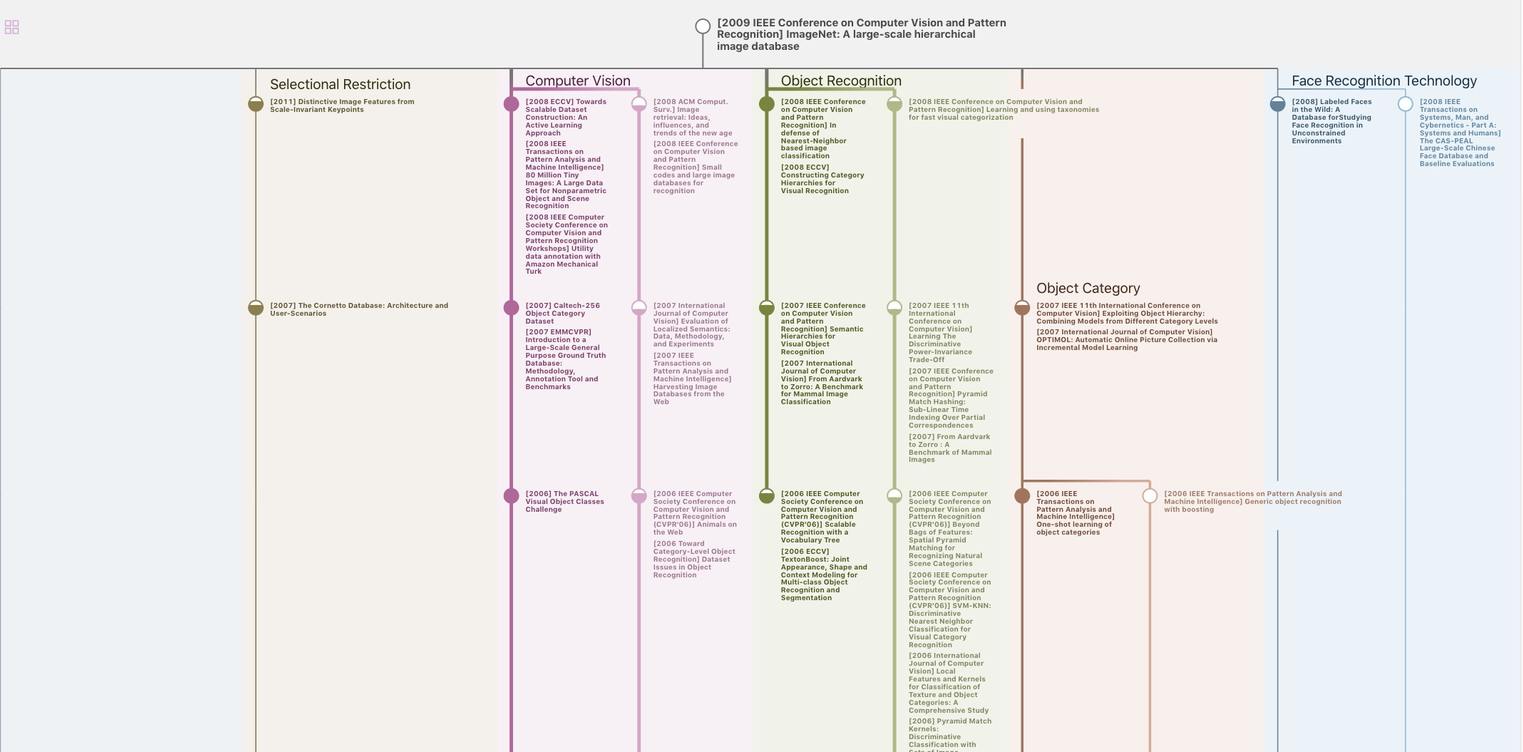
生成溯源树,研究论文发展脉络
Chat Paper
正在生成论文摘要