Levenshtein Training for Word-level Quality Estimation.
EMNLP(2021)
摘要
We propose a novel scheme to use the Levenshtein Transformer to perform the task of word-level quality estimation. A Levenshtein Transformer is a natural fit for this task: trained to perform decoding in an iterative manner, a Levenshtein Transformer can learn to post-edit without explicit supervision. To further minimize the mismatch between the translation task and the word-level QE task, we propose a two-stage transfer learning procedure on both augmented data and human post-editing data. We also propose heuristics to construct reference labels that are compatible with subword-level finetuning and inference. Results on WMT 2020 QE shared task dataset show that our proposed method has superior data efficiency under the data-constrained setting and competitive performance under the unconstrained setting.
更多查看译文
关键词
quality,word-level
AI 理解论文
溯源树
样例
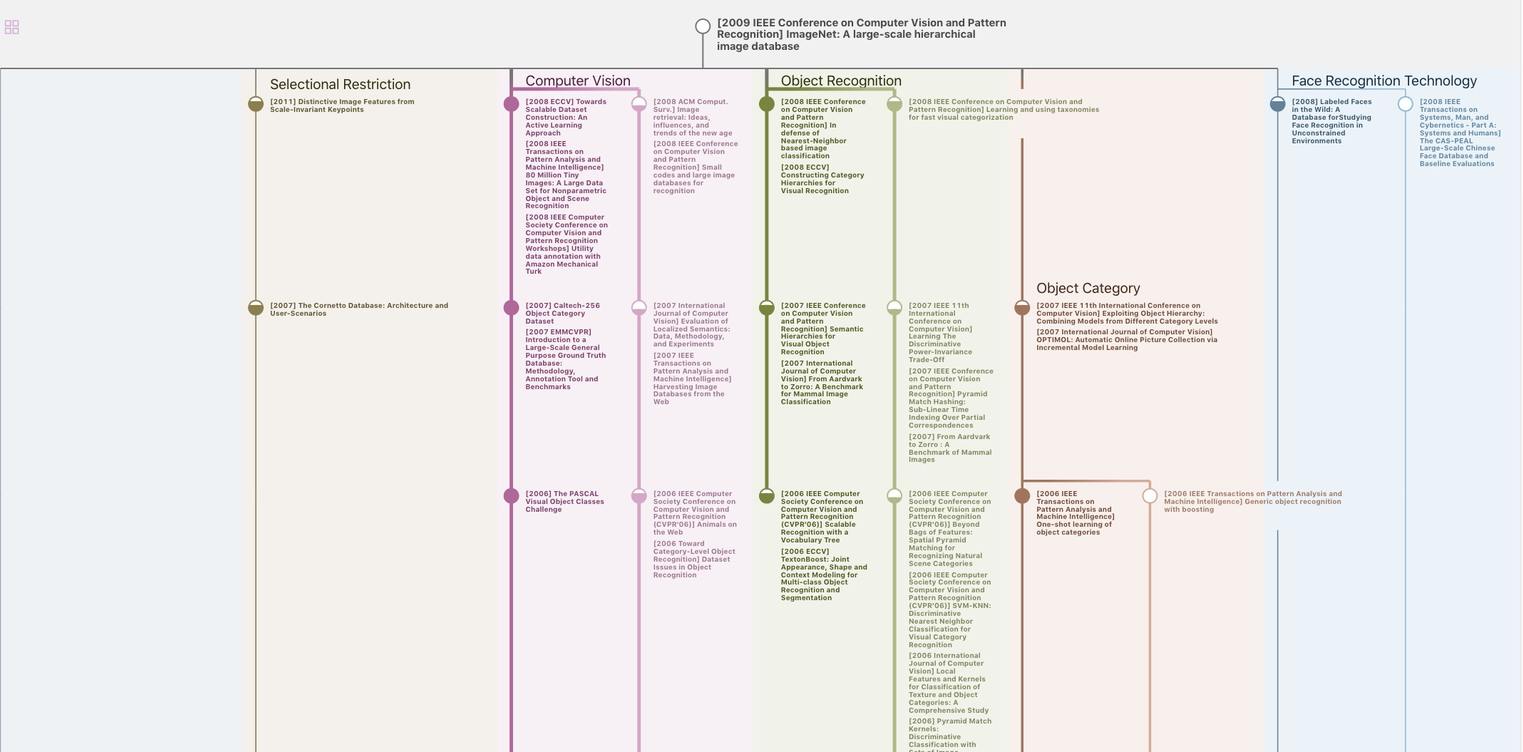
生成溯源树,研究论文发展脉络
Chat Paper
正在生成论文摘要