SphereFace Revived: Unifying Hyperspherical Face Recognition
IEEE Transactions on Pattern Analysis and Machine Intelligence(2023)
摘要
This paper addresses the deep face recognition problem under an open-set protocol, where ideal face features are expected to have smaller maximal intra-class distance than minimal inter-class distance under a suitably chosen metric space. To this end, hyperspherical face recognition, as a promising line of research, has attracted increasing attention and gradually become a major focus in face recognition research. As one of the earliest works in hyperspherical face recognition, SphereFace explicitly proposed to learn face embeddings with large inter-class angular margin. However, SphereFace still suffers from severe training instability which limits its application in practice. In order to address this problem, we introduce a unified framework to understand large angular margin in hyperspherical face recognition. Under this framework, we extend the study of SphereFace and propose an improved variant with substantially better training stability – SphereFace-R. Specifically, we propose two novel ways to implement the multiplicative margin, and study SphereFace-R under three different feature normalization schemes (no feature normalization, hard feature normalization and soft feature normalization). We also propose an implementation strategy – “characteristic gradient detachment” – to stabilize training. Extensive experiments on SphereFace-R show that it is consistently better than or competitive with state-of-the-art methods.
更多查看译文
关键词
Hypersphere,face recognition,angular margin,loss function
AI 理解论文
溯源树
样例
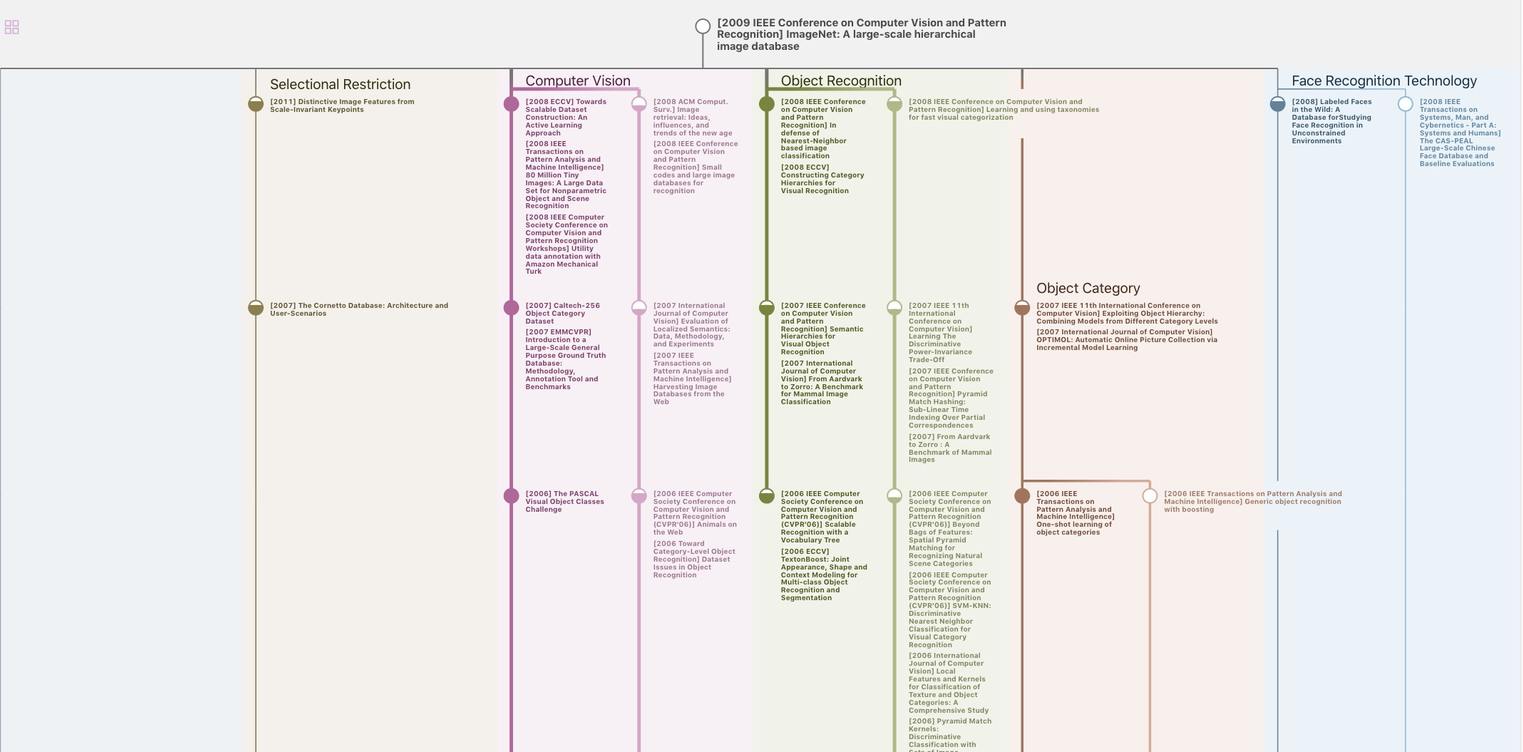
生成溯源树,研究论文发展脉络
Chat Paper
正在生成论文摘要