SanitAIs: Unsupervised Data Augmentation to Sanitize Trojaned Neural Networks
2022 IEEE Intl Conf on Dependable, Autonomic and Secure Computing, Intl Conf on Pervasive Intelligence and Computing, Intl Conf on Cloud and Big Data Computing, Intl Conf on Cyber Science and Technology Congress (DASC/PiCom/CBDCom/CyberSciTech)(2022)
摘要
Self-supervised learning (SSL) methods have resulted in broad improvements to neural network performance by leveraging large, untapped collections of unlabeled data to learn generalized underlying structure. In this work, we harness unsupervised data augmentation (UDA), an SSL technique, to mitigate backdoor or Trojan attacks on deep neural networks. We show that UDA is more effective at removing trojans than current state-of-the-art methods for both feature space and point triggers, over a range of model architectures, trojans, and data quantities provided for trojan removal. These results demonstrate that UDA is both an effective and practical approach to mitigating the effects of backdoors on neural networks.
更多查看译文
关键词
deep learning,trojans,backdoor attack,defense,mitigation
AI 理解论文
溯源树
样例
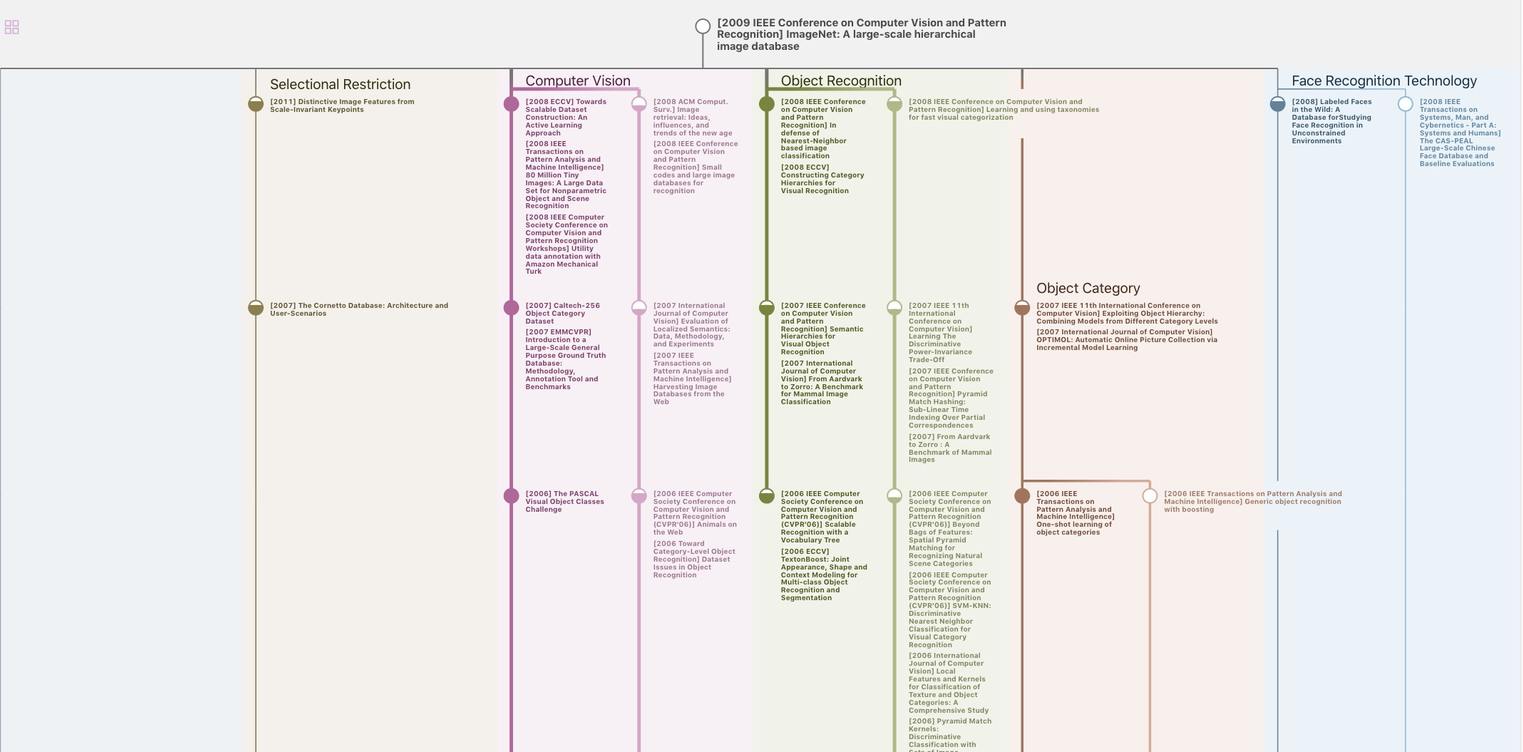
生成溯源树,研究论文发展脉络
Chat Paper
正在生成论文摘要