Scalable Analysis Of Multi-Modal Biomedical Data
GIGASCIENCE(2021)
摘要
Background: Targeted diagnosis and treatment options are dependent on insights drawn from multi-modal analysis of large-scale biomedical datasets. Advances in genomics sequencing, image processing, and medical data management have supported data collection and management within medical institutions. These efforts have produced large-scale datasets and have enabled integrative analyses that provide a more thorough look of the impact of a disease on the underlying system. The integration of large-scale biomedical data commonly involves several complex data transformation steps, such as combining datasets to build feature vectors for learning analysis. Thus, scalable data integration solutions play a key role in the future of targeted medicine. Though large-scale data processing frameworks have shown promising performance for many domains, they fail to support scalable processing of complex datatypes.Solution: To address these issues and achieve scalable processing of multi-modal biomedical data, we present TraNCE, a framework that automates the difficulties of designing distributed analyses with complex biomedical data types. Performance: We outline research and clinical applications for the platform, including data integration support for building feature sets for classification. We show that the system is capable of outperforming the common alternative, based on "flattening" complex data structures, and runs efficiently when alternative approaches are unable to perform at all.
更多查看译文
关键词
nested data, distributed processing, Spark, query compilation, multi-omics analysis, multi-modal data integration
AI 理解论文
溯源树
样例
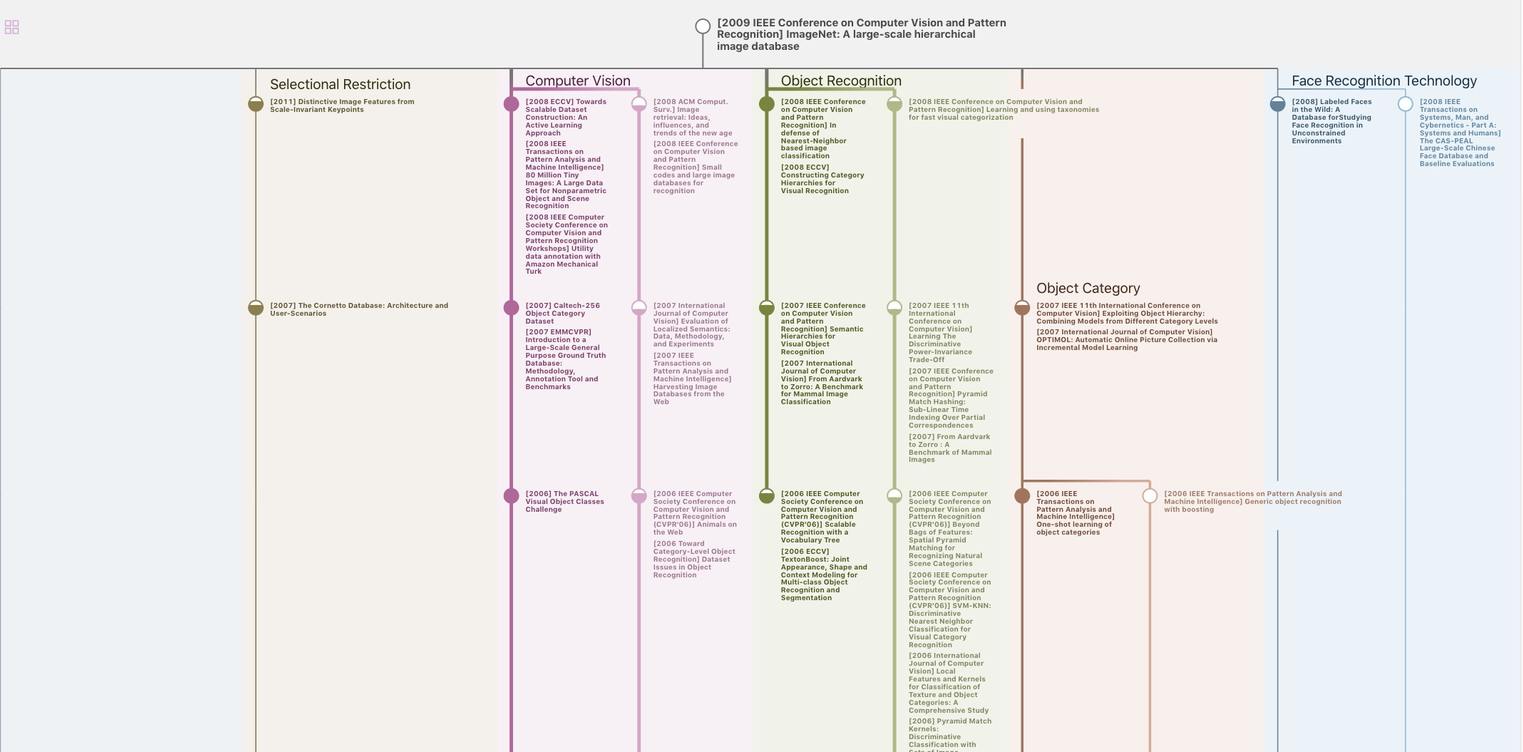
生成溯源树,研究论文发展脉络
Chat Paper
正在生成论文摘要