Generalised Partial Association in Causal Rules Discovery
PROGRESS IN ARTIFICIAL INTELLIGENCE (EPIA 2021)(2021)
摘要
One of the most significant challenges for machine learning nowadays is the discovery of causal relationships from data. This causal discovery is commonly performed using Bayesian like algorithms. However, more recently, more and more causal discovery algorithms have appeared that do not fall into this category. In this paper, we present a new algorithm that explores global causal association rules with Uncertainty Coefficient. Our algorithm, CRPA-UC, is a global structure discovery approach that combines the advantages of association mining with causal discovery and can be applied to binary and non-binary discrete data. This approach was compared to the PC algorithm using several well-known data sets, using several metrics.
更多查看译文
关键词
Causality, Causal association rules, Generalised Cochran-Mantel-Haenszel, Uncertainty coefficient
AI 理解论文
溯源树
样例
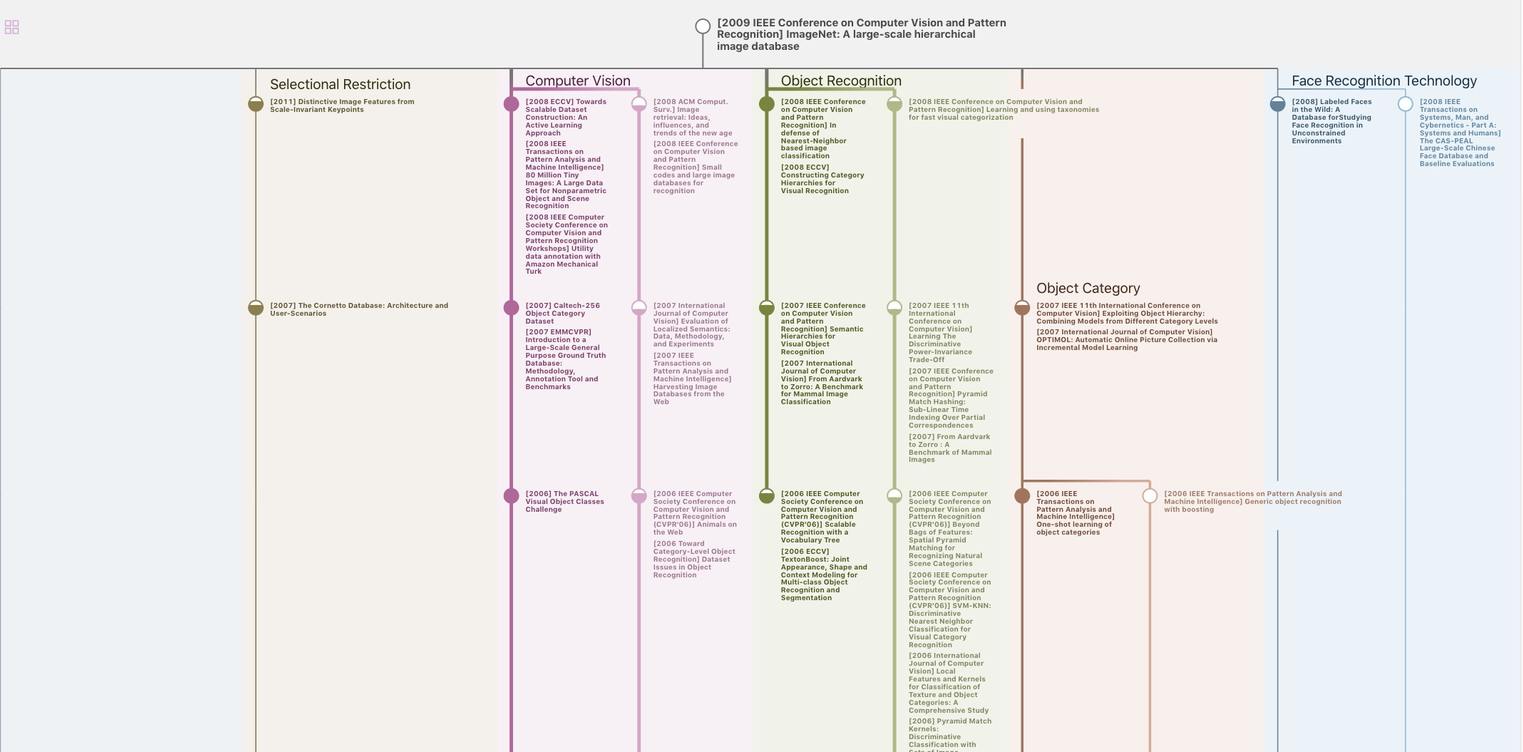
生成溯源树,研究论文发展脉络
Chat Paper
正在生成论文摘要