RFDoc: Memory Efficient Local Descriptors for ID Documents Localization and Classification
DOCUMENT ANALYSIS AND RECOGNITION - ICDAR 2021, PT II(2021)
摘要
Majority of recent papers in the field of image matching introduces universal descriptors for arbitrary keypoints matching. In this paper we propose a data-driven approach to building a descriptor for matching local keypoints from identity documents in the context of simultaneous ID document localization and classification on mobile devices. In the first stage, we train features robust to lighting changes. In the second stage, we select the most best-performing and discriminant features and forms a set of classifiers which we called RFDoc descriptor. To address the problem of limited computing resources the proposed descriptor is binary rather than a real-valued one. To perform experiments we prepared a dataset of aligned patches from a subset of identity document types presented in MIDV datasets and made it public. RFDoc descriptor showed similar performance in complex document detection and classification on the test part of the MIDV-500 dataset to the state-of-the-art BEBLID-512 descriptor, which is more than 2.6 times less memory efficient than RFDoc. On a more complex MIDV-2019 dataset RFDoc showed 21% fewer classification errors.
更多查看译文
关键词
Local features learning, Document classification, Identity documents recognition, Keypoint descriptors
AI 理解论文
溯源树
样例
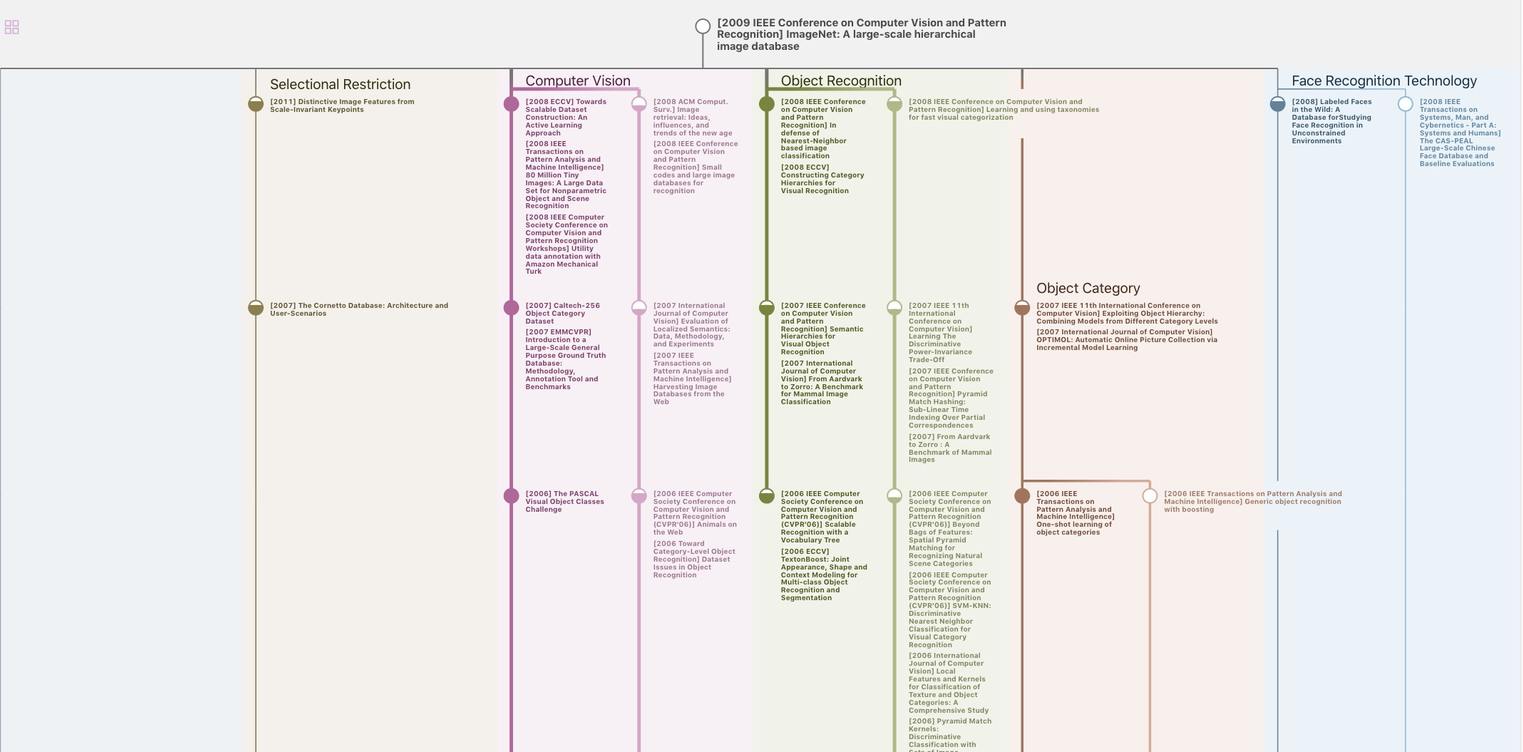
生成溯源树,研究论文发展脉络
Chat Paper
正在生成论文摘要