Data Augmentation for End-to-End Optical Music Recognition
DOCUMENT ANALYSIS AND RECOGNITION, ICDAR 2021 WORKSHOPS, PT I(2021)
摘要
Optical Music Recognition (OMR) is the research area that studies how to transcribe the content from music documents into a structured digital format. Within this field, techniques based on Deep Learning represent the current state of the art. Nevertheless, their use is constrained by the large amount of labeled data required, which constitutes a relevant issue when dealing with historical manuscripts. This drawback can be palliated by means of Data Augmentation (DA), which encompasses a series of strategies to increase data without the need of manual labeling new images. This work studies the applicability of specific DA techniques in the context of end-to-end staff-level OMR methods. More precisely, considering two corpora of historical music manuscripts, we applied different types of distortions to the music scores and assessed their contribution in an end-to-end system. Our results show that some transformations are much more appropriate than others, leading up to a 34.5% of relative improvement with respect to scenario without DA.
更多查看译文
关键词
Optical music recognition, Data augmentation, Deep learning
AI 理解论文
溯源树
样例
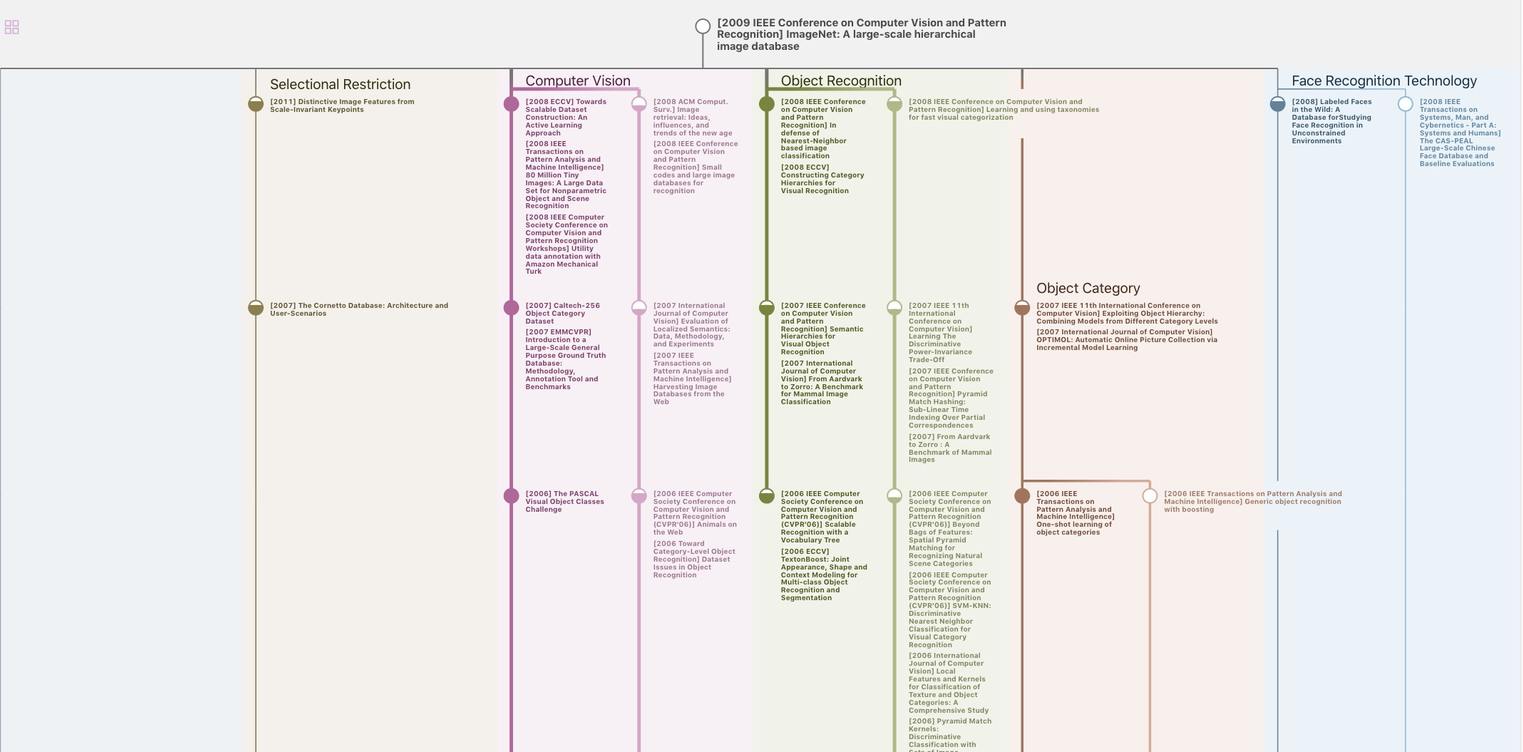
生成溯源树,研究论文发展脉络
Chat Paper
正在生成论文摘要