Prediction of lung emphysema in COPD by spirometry and clinical symptoms: results from COSYCONET
RESPIRATORY RESEARCH(2021)
摘要
Background Lung emphysema is an important phenotype of chronic obstructive pulmonary disease (COPD), and CT scanning is strongly recommended to establish the diagnosis. This study aimed to identify criteria by which physicians with limited technical resources can improve the diagnosis of emphysema. Methods We studied 436 COPD patients with prospective CT scans from the COSYCONET cohort. All items of the COPD Assessment Test (CAT) and the St George’s Respiratory Questionnaire (SGRQ), the modified Medical Research Council (mMRC) scale, as well as data from spirometry and CO diffusing capacity, were used to construct binary decision trees. The importance of parameters was checked by the Random Forest and AdaBoost machine learning algorithms. Results When relying on questionnaires only, items CAT 1 & 7 and SGRQ 8 & 12 sub-item 3 were most important for the emphysema- versus airway-dominated phenotype, and among the spirometric measures FEV 1 /FVC. The combination of CAT item 1 (≤ 2) with mMRC (> 1) and FEV 1 /FVC, could raise the odds for emphysema by factor 7.7. About 50% of patients showed combinations of values that did not markedly alter the likelihood for the phenotypes, and these could be easily identified in the trees. Inclusion of CO diffusing capacity revealed the transfer coefficient as dominant measure. The results of machine learning were consistent with those of the single trees. Conclusions Selected items (cough, sleep, breathlessness, chest condition, slow walking) from comprehensive COPD questionnaires in combination with FEV 1 /FVC could raise or lower the likelihood for lung emphysema in patients with COPD. The simple, parsimonious approach proposed by us might help if diagnostic resources regarding respiratory diseases are limited. Trial registration ClinicalTrials.gov, Identifier: NCT01245933, registered 18 November 2010, https://clinicaltrials.gov/ct2/show/record/NCT01245933 .
更多查看译文
关键词
Emphysema, CT scan, Decision trees, Random forest, Adaboost, COPD phenotypes
AI 理解论文
溯源树
样例
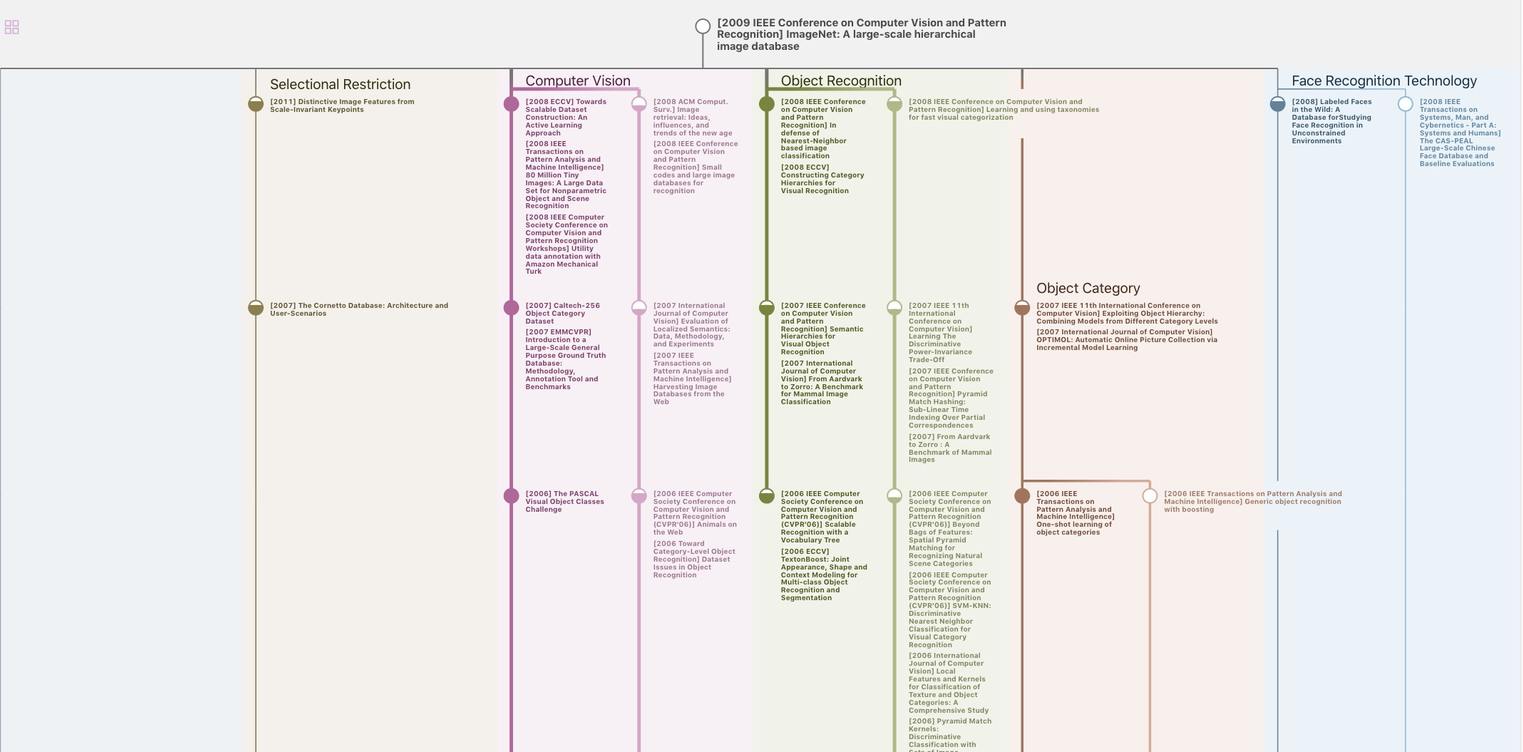
生成溯源树,研究论文发展脉络
Chat Paper
正在生成论文摘要