Detecting and Mitigating Test-time Failure Risks via Model-agnostic Uncertainty Learning
2021 21ST IEEE INTERNATIONAL CONFERENCE ON DATA MINING (ICDM 2021)(2021)
摘要
Reliably predicting potential failure risks of machine learning (ML) systems when deployed with production data is a crucial aspect of trustworthy AI. This paper introduces Risk Advisor, a novel post-hoc meta-learner for estimating failure risks and predictive uncertainties of any already-trained black-box classification model. In addition to providing a risk score, the Risk Advisor decomposes the uncertainty estimates into aleatoric and epistemic uncertainty components, thus giving informative insights into the sources of uncertainty inducing the failures. Consequently, Risk Advisor can distinguish between failures caused by data variability, data shifts and model limitations and advise on mitigation actions (e.g., collecting more data to counter data shift). Extensive experiments on real-world datasets covering a variety of ML failure scenarios show that the Risk Advisor reliably predicts deployment-time failure risks in all the scenarios, and outperforms strong baselines.
更多查看译文
关键词
Responsible AI,Trustworthy AI,Model Uncertainty,Error Analysis
AI 理解论文
溯源树
样例
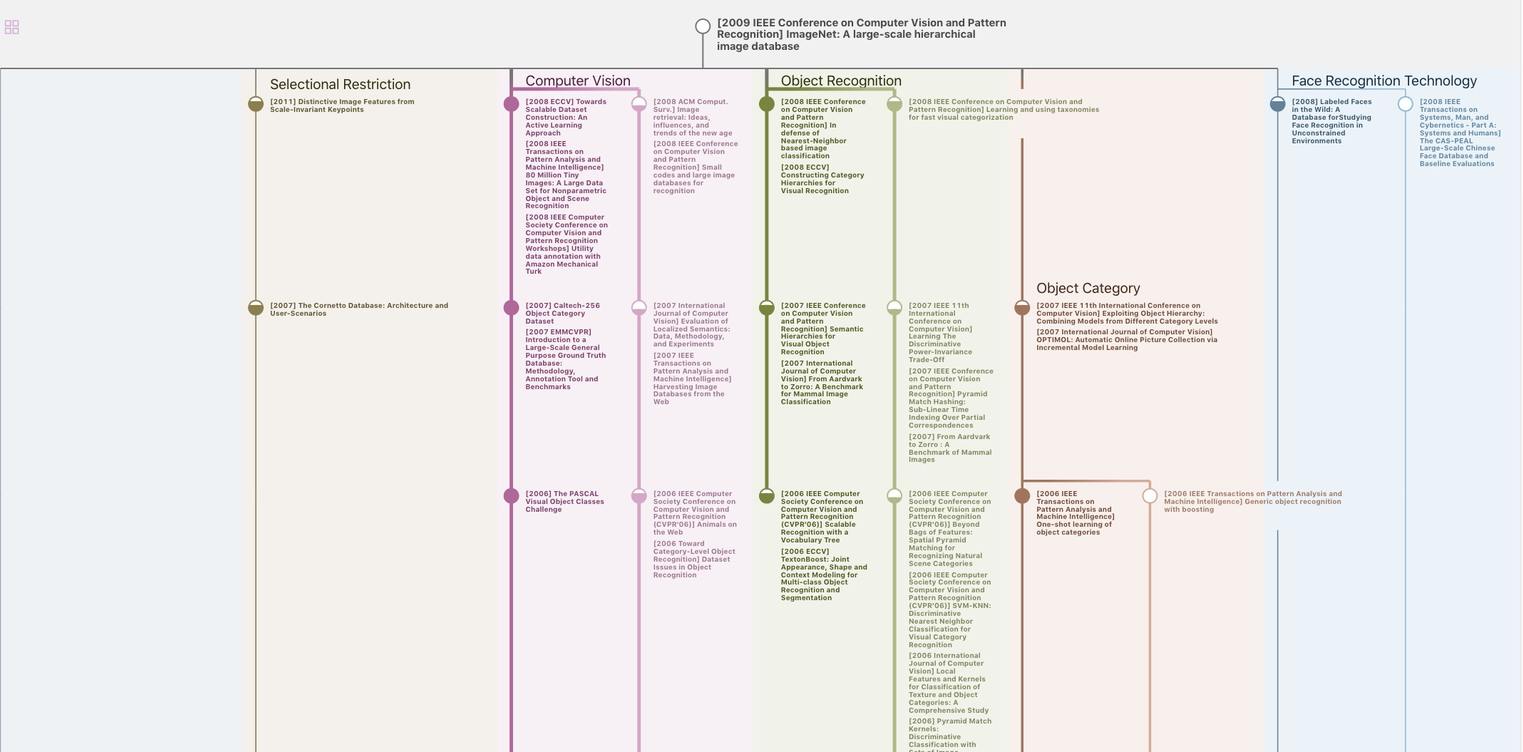
生成溯源树,研究论文发展脉络
Chat Paper
正在生成论文摘要