Neural-IMLS: Self-supervised Implicit Moving Least-Squares Network for Surface Reconstruction
arxiv(2022)
摘要
Surface reconstruction is very challenging when the input point clouds, particularly real scans, are noisy and lack normals. Observing that the Multilayer Perceptron (MLP) and the implicit moving least-square function (IMLS) provide a dual representation of the underlying surface, we introduce Neural-IMLS, a novel approach that directly learns the noise-resistant signed distance function (SDF) from unoriented raw point clouds in a self-supervised fashion. We use the IMLS to regularize the distance values reported by the MLP while using the MLP to regularize the normals of the data points for running the IMLS. We also prove that at the convergence, our neural network, benefiting from the mutual learning mechanism between the MLP and the IMLS, produces a faithful SDF whose zero-level set approximates the underlying surface. We conducted extensive experiments on various benchmarks, including synthetic scans and real scans. The experimental results show that {\em Neural-IMLS} can reconstruct faithful shapes on various benchmarks with noise and missing parts. The source code can be found at~\url{https://github.com/bearprin/Neural-IMLS}.
更多查看译文
关键词
surface reconstruction,self-supervised neural network,implicit neural representations,implicit moving least squares
AI 理解论文
溯源树
样例
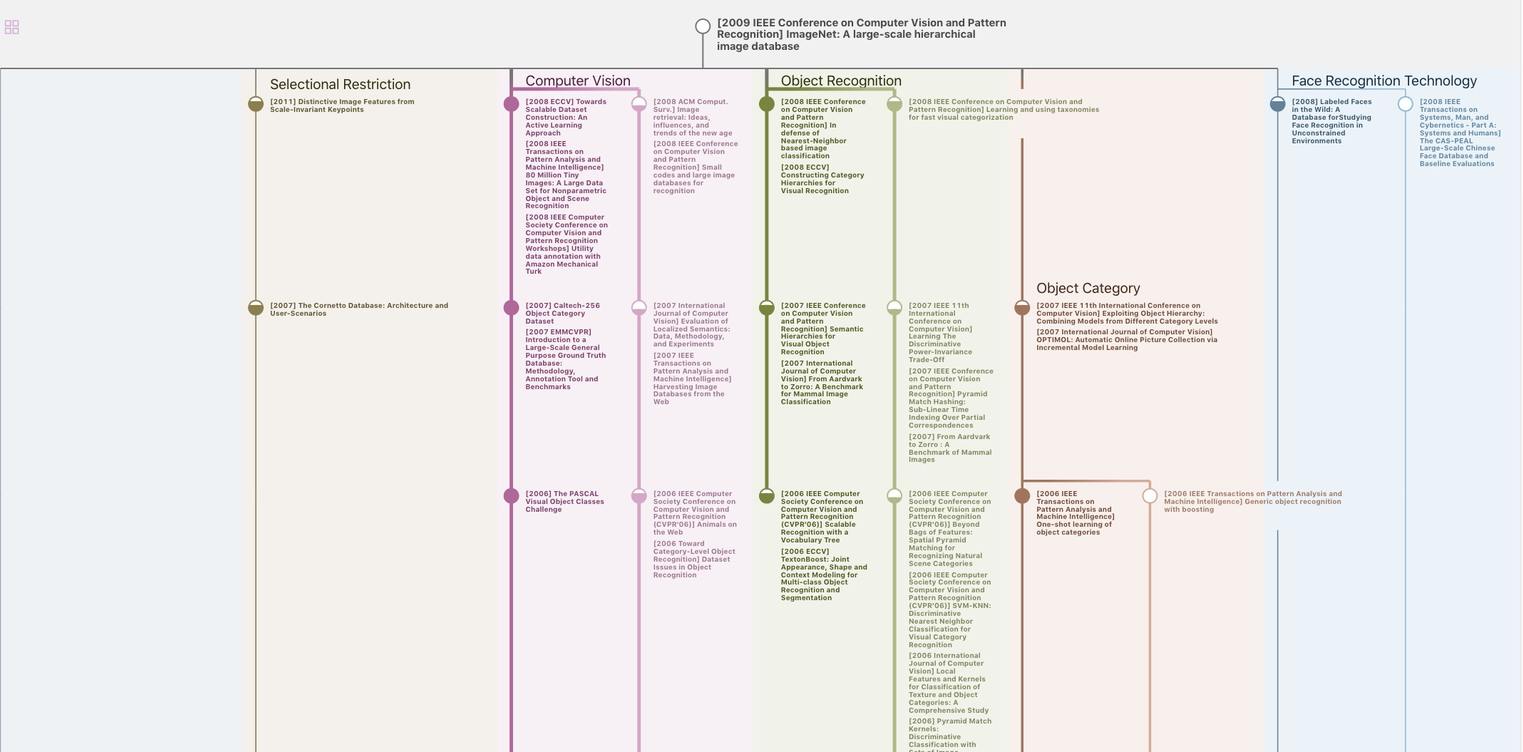
生成溯源树,研究论文发展脉络
Chat Paper
正在生成论文摘要