Translate & Fill - Improving Zero-Shot Multilingual Semantic Parsing with Synthetic Data.
EMNLP(2021)
摘要
While multilingual pretrained language models (LMs) fine-tuned on a single language have shown substantial cross-lingual task transfer capabilities, there is still a wide performance gap in semantic parsing tasks when target language supervision is available. In this paper, we propose a novel Translate-and-Fill (TaF) method to produce silver training data for a multilingual semantic parser. This method simplifies the popular Translate-Align-Project (TAP) pipeline and consists of a sequence-to-sequence filler model that constructs a full parse conditioned on an utterance and a view of the same parse. Our filler is trained on English data only but can accurately complete instances in other languages (i.e., translations of the English training utterances), in a zero-shot fashion. Experimental results on three multilingual semantic parsing datasets show that data augmentation with TaF reaches accuracies competitive with similar systems which rely on traditional alignment techniques.
更多查看译文
关键词
translate,zero-shot
AI 理解论文
溯源树
样例
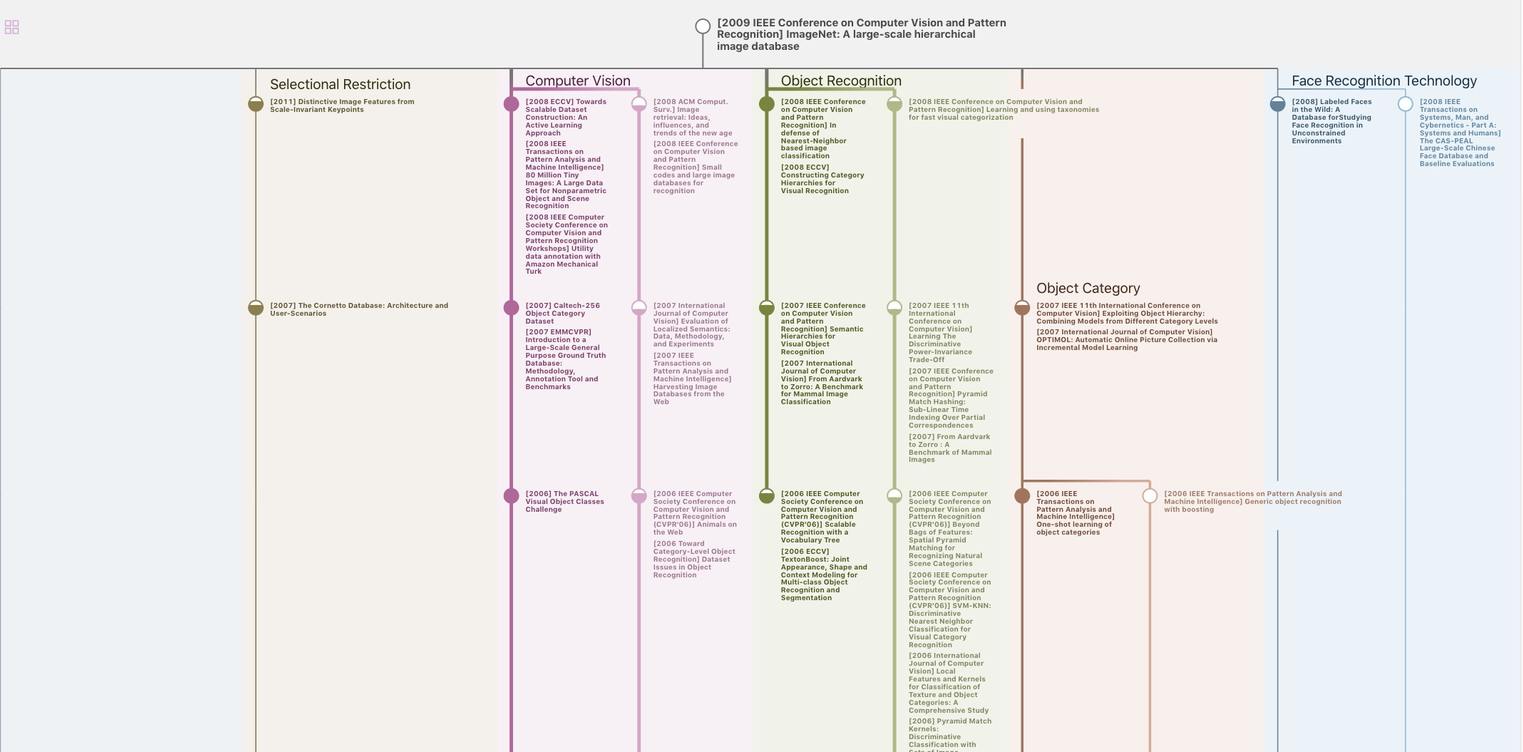
生成溯源树,研究论文发展脉络
Chat Paper
正在生成论文摘要