Rethinking Data Augmentation for Low-Resource Neural Machine Translation - A Multi-Task Learning Approach.
EMNLP(2021)
摘要
In the context of neural machine translation, data augmentation (DA) techniques may be used for generating additional training samples when the available parallel data are scarce. Many DA approaches aim at expanding the support of the empirical data distribution by generating new sentence pairs that contain infrequent words, thus making it closer to the true data distribution of parallel sentences. In this paper, we propose to follow a completely different approach and present a multi-task DA approach in which we generate new sentence pairs with transformations, such as reversing the order of the target sentence, which produce unfluent target sentences. During training, these augmented sentences are used as auxiliary tasks in a multi-task framework with the aim of providing new contexts where the target prefix is not informative enough to predict the next word. This strengthens the encoder and forces the decoder to pay more attention to the source representations of the encoder. Experiments carried out on six low-resource translation tasks show consistent improvements over the baseline and over DA methods aiming at extending the support of the empirical data distribution. The systems trained with our approach rely more on the source tokens, are more robust against domain shift and suffer less hallucinations.
更多查看译文
关键词
neural machine
AI 理解论文
溯源树
样例
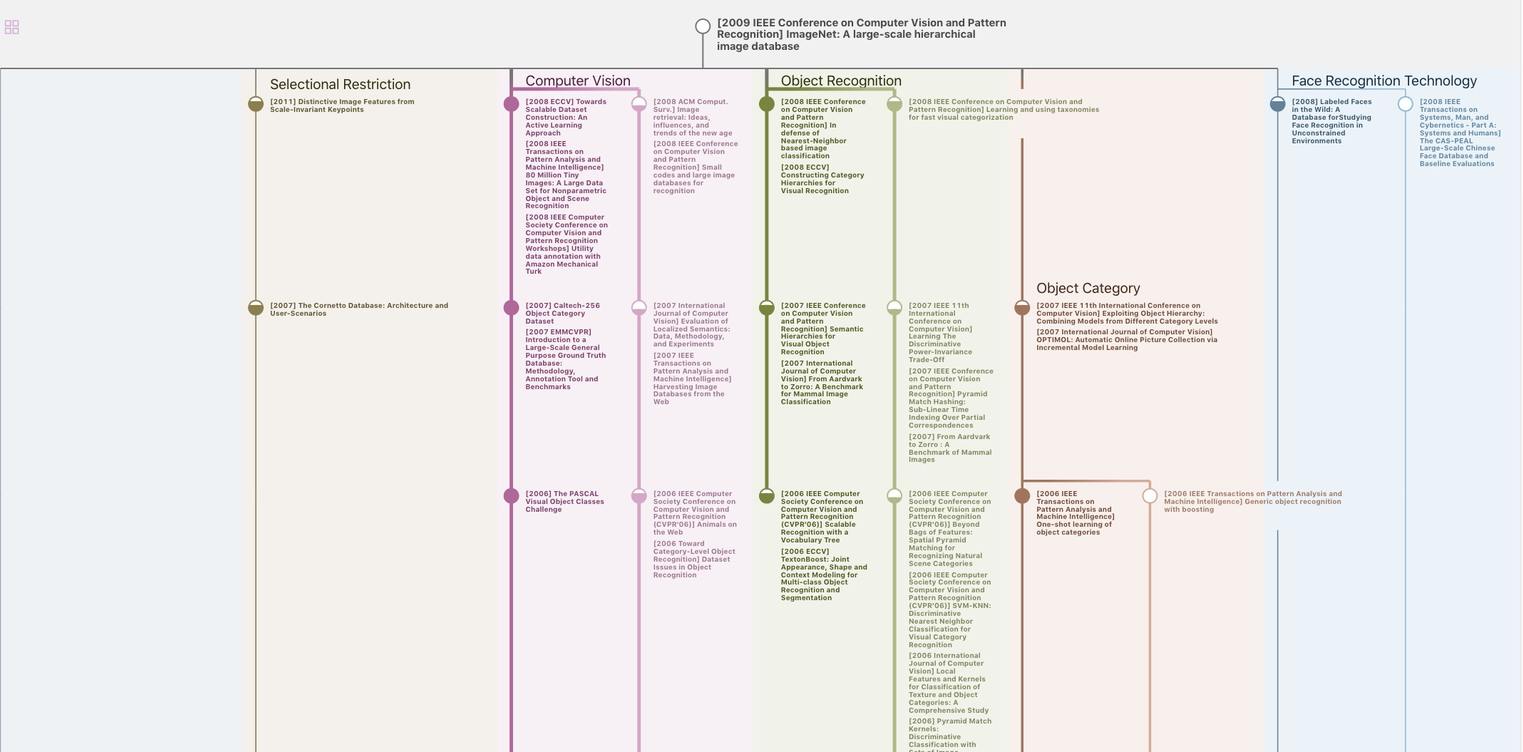
生成溯源树,研究论文发展脉络
Chat Paper
正在生成论文摘要