Accelerated Zeroth-Order Algorithm for Stochastic Distributed Non-Convex Optimization.
ACC(2022)
摘要
This paper investigates how to accelerate the convergence of distributed optimization algorithms on nonconvex problems with zeroth-order information available only. We propose a zeroth-order (ZO) distributed primal-dual stochastic coordinates algorithm equipped with "powerball" method to accelerate. We prove that the proposed algorithm has a convergence rate of $\mathcal{O}(\sqrt{p}/\sqrt{nT})$ for general nonconvex cost functions. We consider solving the generation of adversarial examples from black-box DNNs problem to compare with the existing state-of-the-art centralized and distributed ZO algorithms. The numerical results demonstrate the faster convergence rate of the proposed algorithm and match the theoretical analysis.
更多查看译文
关键词
faster convergence rate,zeroth-order algorithm,stochastic distributed nonconvex optimization,distributed optimization algorithms,nonconvex problems,zeroth-order information,primal-dual stochastic coordinate algorithm,powerball method,convergence result,considered algorithm,nonconvex cost functions,ZO algorithms
AI 理解论文
溯源树
样例
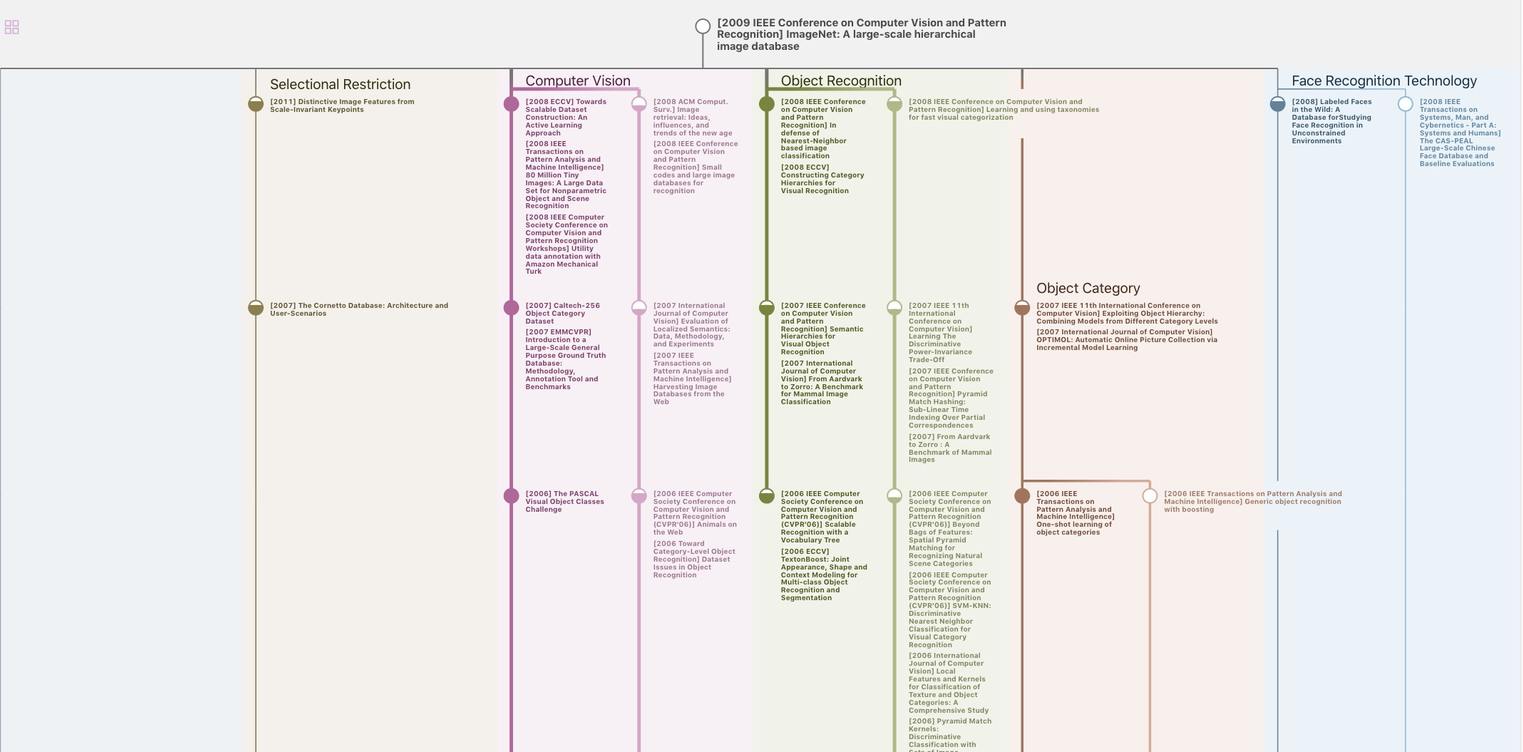
生成溯源树,研究论文发展脉络
Chat Paper
正在生成论文摘要