Infusing Future Information into Monotonic Attention Through Language Models
arxiv(2021)
摘要
Simultaneous neural machine translation(SNMT) models start emitting the target sequence before they have processed the source sequence. The recent adaptive policies for SNMT use monotonic attention to perform read/write decisions based on the partial source and target sequences. The lack of sufficient information might cause the monotonic attention to take poor read/write decisions, which in turn negatively affects the performance of the SNMT model. On the other hand, human translators make better read/write decisions since they can anticipate the immediate future words using linguistic information and domain knowledge.Motivated by human translators, in this work, we propose a framework to aid monotonic attention with an external language model to improve its decisions.We conduct experiments on the MuST-C English-German and English-French speech-to-text translation tasks to show the effectiveness of the proposed framework.The proposed SNMT method improves the quality-latency trade-off over the state-of-the-art monotonic multihead attention.
更多查看译文
关键词
monotonic attention,future information
AI 理解论文
溯源树
样例
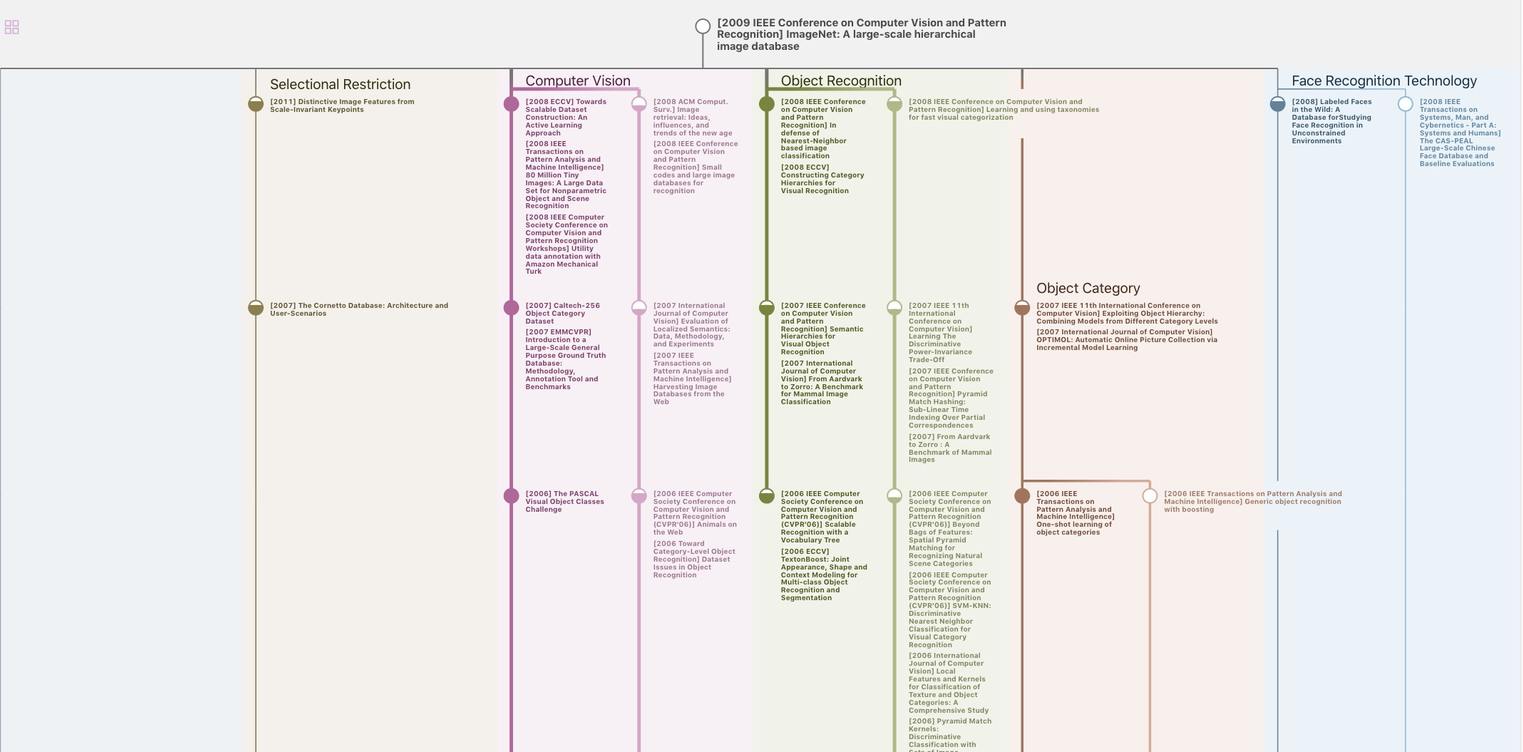
生成溯源树,研究论文发展脉络
Chat Paper
正在生成论文摘要