Robust Event Detection based on Spatio-Temporal Latent Action Unit using Skeletal Information
2021 IEEE/RSJ INTERNATIONAL CONFERENCE ON INTELLIGENT ROBOTS AND SYSTEMS (IROS)(2021)
摘要
This paper proposes a novel dictionary learning approach to detect event anomalities using skeletal information extracted from RGBD video. The event action is represented as several latent action atoms and composed of latent spatial and temporal attributes. We aim to construct a network able to learn from few examples and also rules defined by the user. The skeleton frames are clustered by an initial K-means method. Each skeleton frame is assigned with a varying weight parameter and fed into our Gradual Online Dictionary Learning (GODL) algorithm. During the training process, outlier frames will be gradually filtered by reducing the weight that is inversely proportional to a cost. To strictly distinguish the event action from similar actions and robustly acquire its action units, we build a latent unit temporal structure for each sub-action. We validate the method at the example of fall event detection on NTU RGB+D dataset, because it provides a benchmark available for comparison. We present the experimental validation of the achieved accuracy, recall, and precision. Our approach achieves the best performance in precision and accuracy of human fall event detection, compared with other existing dictionary learning methods. Our method remains the highest accuracy and the lowest variance, with increasing noise ratio.
更多查看译文
关键词
robust event detection,skeletal information,action,spatio-temporal
AI 理解论文
溯源树
样例
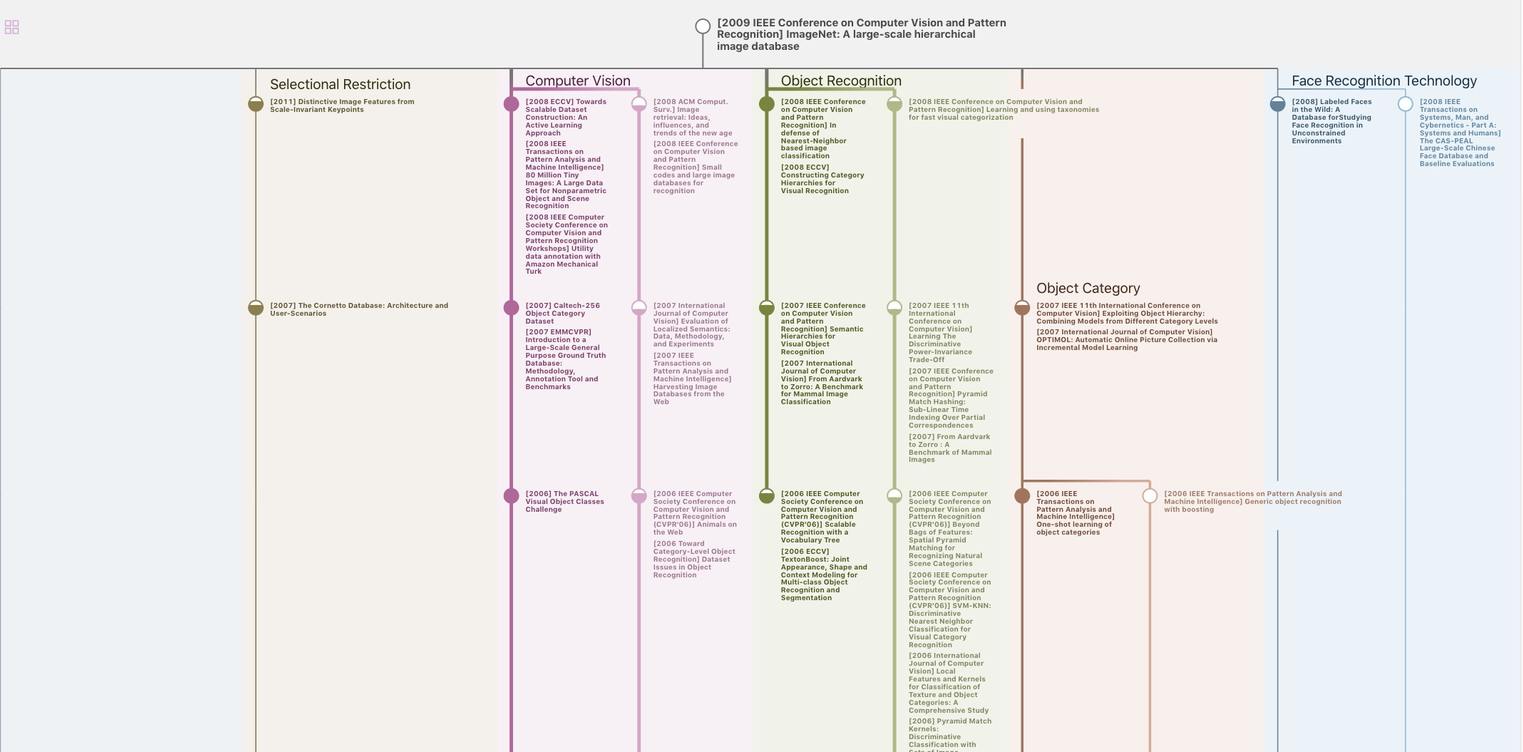
生成溯源树,研究论文发展脉络
Chat Paper
正在生成论文摘要