Learning-Based Strategy Design for Robot-Assisted Reminiscence Therapy Based on a Developed Model for People with Dementia
SOCIAL ROBOTICS, ICSR 2021(2021)
摘要
In this paper, the robot-assisted Reminiscence Therapy (RT) is studied as a psychosocial intervention to persons with dementia (PwDs). We aim at a conversation strategy for the robot by reinforcement learning to stimulate the PwD to talk. Specifically, to characterize the stochastic reactions of a PwD to the robot's actions, a simulation model of a PwD is developed which features the transition probabilities among different PwD states consisting of the response relevance, emotion levels and confusion conditions. A Q-learning (QL) algorithm is then designed to achieve the best conversation strategy for the robot. The objective is to stimulate the PwD to talk as much as possible while keeping the PwD's states as positive as possible. In certain conditions, the achieved strategy gives the PwD choices to continue or change the topic, or stop the conversation, so that the PwD has a sense of control to mitigate the conversation stress. To achieve this, the standard QL algorithm is revised to deliberately integrate the impact of PwD's choices into the Q-value updates. Finally, the simulation results demonstrate the learning convergence and validate the efficacy of the achieved strategy. Tests show that the strategy is capable to duly adjust the difficulty level of prompt according to the PwD's states, take actions (e.g., repeat or explain the prompt, or comfort) to help the PwD out of bad states, and allow the PwD to control the conversation tendency when bad states continue.
更多查看译文
关键词
Social robot, Reminiscence therapy, Reinforcement learning, Dementia care
AI 理解论文
溯源树
样例
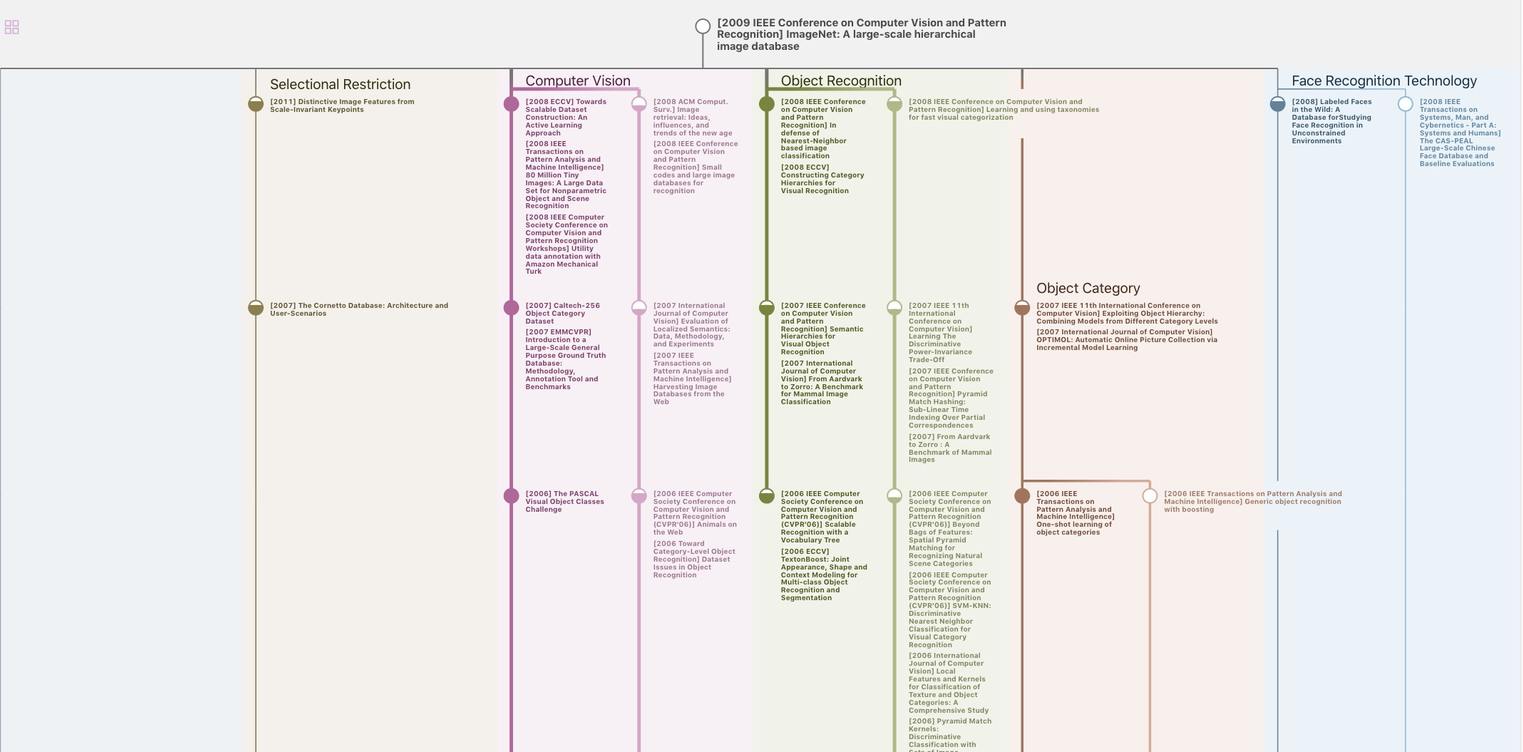
生成溯源树,研究论文发展脉络
Chat Paper
正在生成论文摘要