VARGAN: variance enforcing network enhanced GAN
arxiv(2022)
摘要
Generative adversarial networks (GANs) are one of the most widely used generative models. GANs can learn complex multi-modal distributions, and generate real-like samples. Despite the major success of GANs in generating synthetic data, they might suffer from unstable training process, and mode collapse. In this paper, we propose a new GAN architecture called variance enforcing GAN (VARGAN), which incorporates a third network to introduce diversity in the generated samples. The third network measures the diversity of the generated samples, which is used to penalize the generator’s loss for low diversity samples. The network is trained on the available training data and undesired distributions with limited modality. On a set of synthetic and real-world image data, VARGAN generates a more diverse set of samples compared to the recent state-of-the-art models. High diversity and low computational complexity, as well as fast convergence, make VARGAN a promising model to alleviate mode collapse.
更多查看译文
关键词
Generative adversarial networks, Mode collapse, Multi-modal distribution, Variance enforcing network
AI 理解论文
溯源树
样例
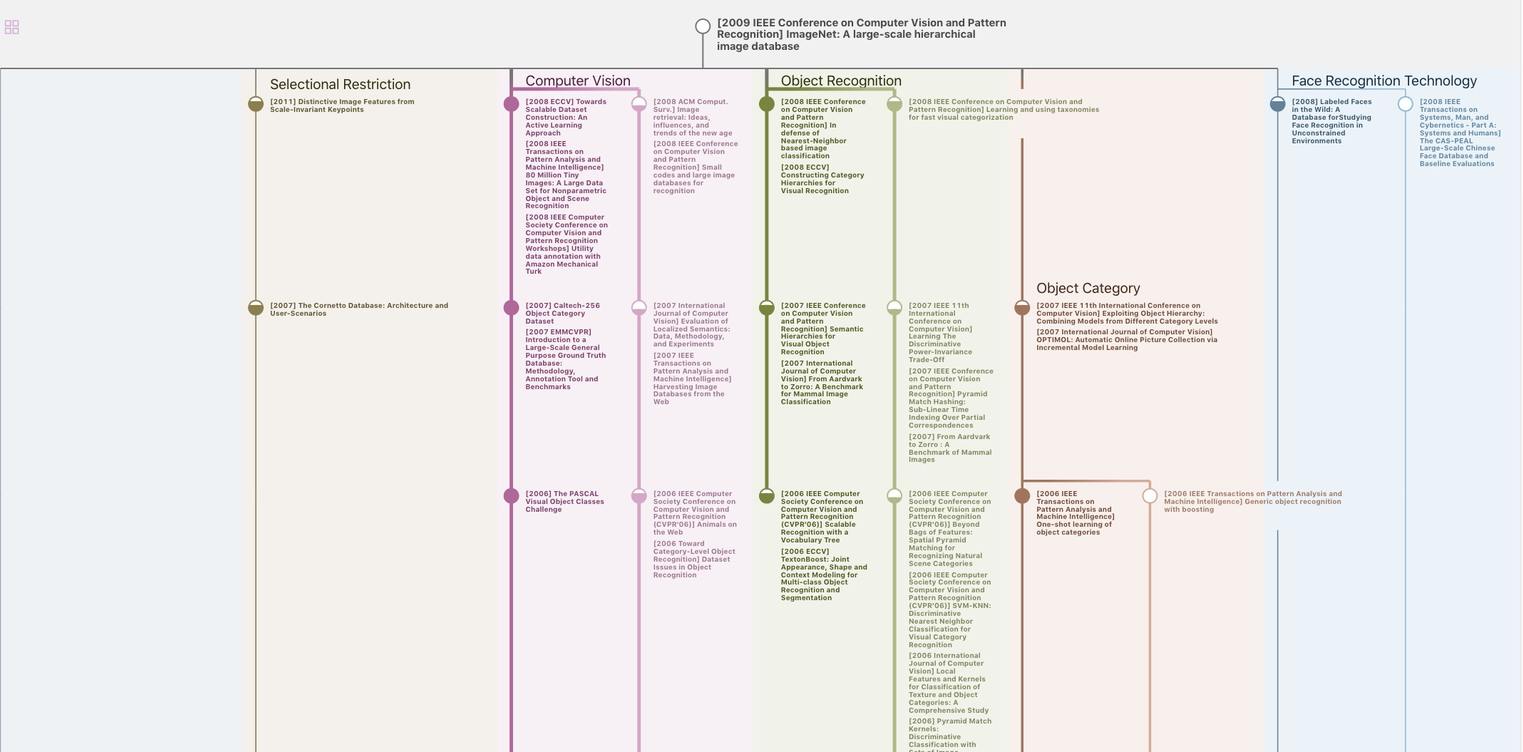
生成溯源树,研究论文发展脉络
Chat Paper
正在生成论文摘要