Sparse-MLP: A Fully-MLP Architecture with Conditional Computation
arxiv(2021)
摘要
Mixture of Experts (MoE) with sparse conditional computation has been proved an effective architecture for scaling attention-based models to more parameters with comparable computation cost. In this paper, we propose Sparse-MLP, scaling the recent MLP-Mixer model with sparse MoE layers, to achieve a more computation-efficient architecture. We replace a subset of dense MLP blocks in the MLP-Mixer model with Sparse blocks. In each Sparse block, we apply two stages of MoE layers: one with MLP experts mixing information within channels along image patch dimension, one with MLP experts mixing information within patches along the channel dimension. Besides, to reduce computational cost in routing and improve experts capacity, we design Re-represent layers in each Sparse block. These layers are to re-scale image representations by two simple but effective linear transformations. By pre-training on ImageNet-1k with MoCo v3 algorithm, our models can outperform dense MLP models with comparable parameters and less computational cost on several downstream image classification tasks.
更多查看译文
关键词
conditional computation,architecture,sparse-mlp,fully-mlp
AI 理解论文
溯源树
样例
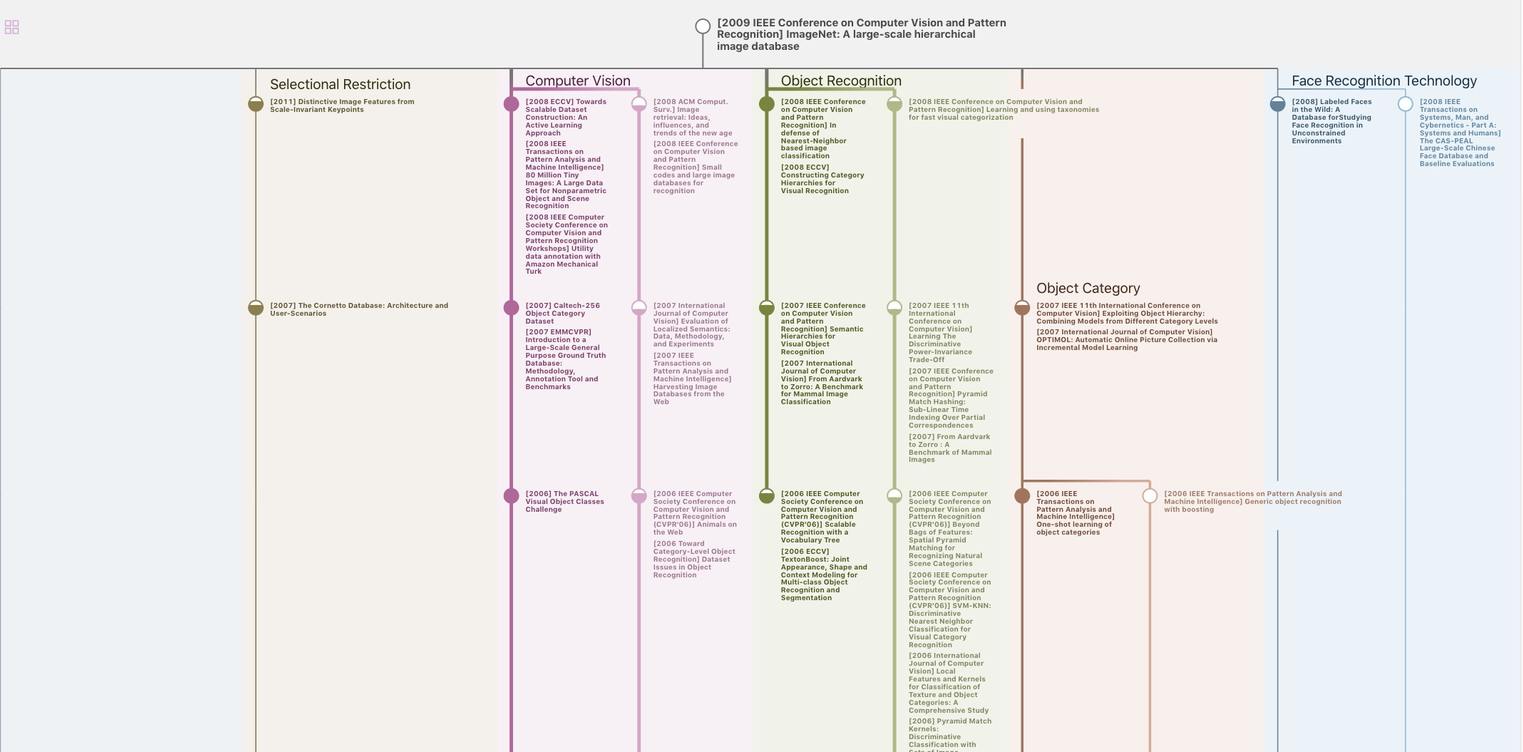
生成溯源树,研究论文发展脉络
Chat Paper
正在生成论文摘要