M-DFNet: Multi-phase Discriminative Feature Network for Retrieval of Focal Liver Lesions
International Multimedia Conference(2021)
摘要
ABSTRACTContent based medical image retrieval (CBMIR) plays a great role in computer aided diagnosis for assisting radiologists to detect and characterize focal liver lesions (FLLs). Deep learning has gained exciting performance on CBMIR. While the features generated by deep learning models trained using softmax loss are always separable but not discriminative enough, which is insufficient for retrieval task. In this paper, we propose a multi-phase discriminative feature network (M-DFNet) with a DeepExtracter and a feature refine module (FRModule) to learn discriminative and separable features under a joint supervision of center loss and softmax loss. The hybrid loss enables to minimize intra-class variations and enlarge inter-class differences as much as possible. The FRModule is proposed to recalibrate the deep features based on the learned class centers to tackle the complex imaging manifestations of FLLs and further enhance both the feature discrimination and generalization. Multi-phase computed tomography (CT) images contain pivotal information for diagnosis of FLLs. Thus the M-DFNet is designed to cope with multi-phase information and we explore an appropriate and effective method for multi-phase feature integration on limited data. Experimental results clearly demonstrate strong performance superiority by our proposed method.
更多查看译文
关键词
CBMIR, Discriminative Feature Network, Multi-phase CT, Focal liver lesions
AI 理解论文
溯源树
样例
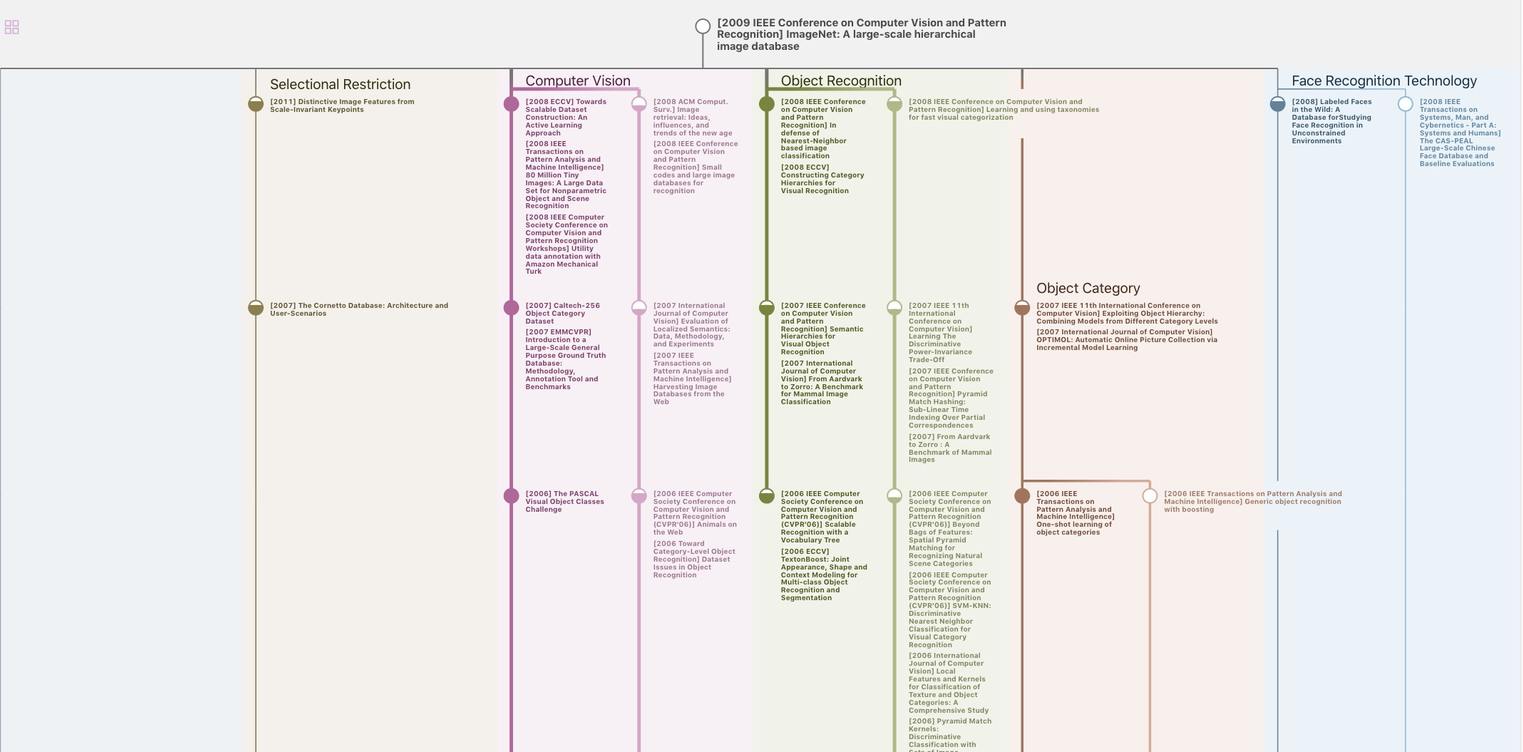
生成溯源树,研究论文发展脉络
Chat Paper
正在生成论文摘要