Practically Solving Lpn
2021 IEEE INTERNATIONAL SYMPOSIUM ON INFORMATION THEORY (ISIT)(2021)
摘要
The best algorithms for the Learning Parity with Noise (LPN) problem require sub-exponential time and memory. This often makes memory, and not time, the limiting factor for practical attacks, which seem to be out of reach even for relatively small parameters. In this paper, we try to bring the state-of-the-art in solving LPN closer to the practical realm. We improve upon the existing algorithms by modifying the Coded-BKW algorithm to work under various memory constrains. We correct and expand previous analysis and experimentally verify our findings. As a result we were able to mount practical attacks on the largest parameters reported to date using only 2(39) bits of memory.
更多查看译文
关键词
solving LPN,Learning Parity,Noise problem,sub-exponential time,limiting factor,practical attacks,relatively small parameters,LPN closer,practical realm,Coded-BKW algorithm,memory constrains,largest parameters,word length 239.0 bit
AI 理解论文
溯源树
样例
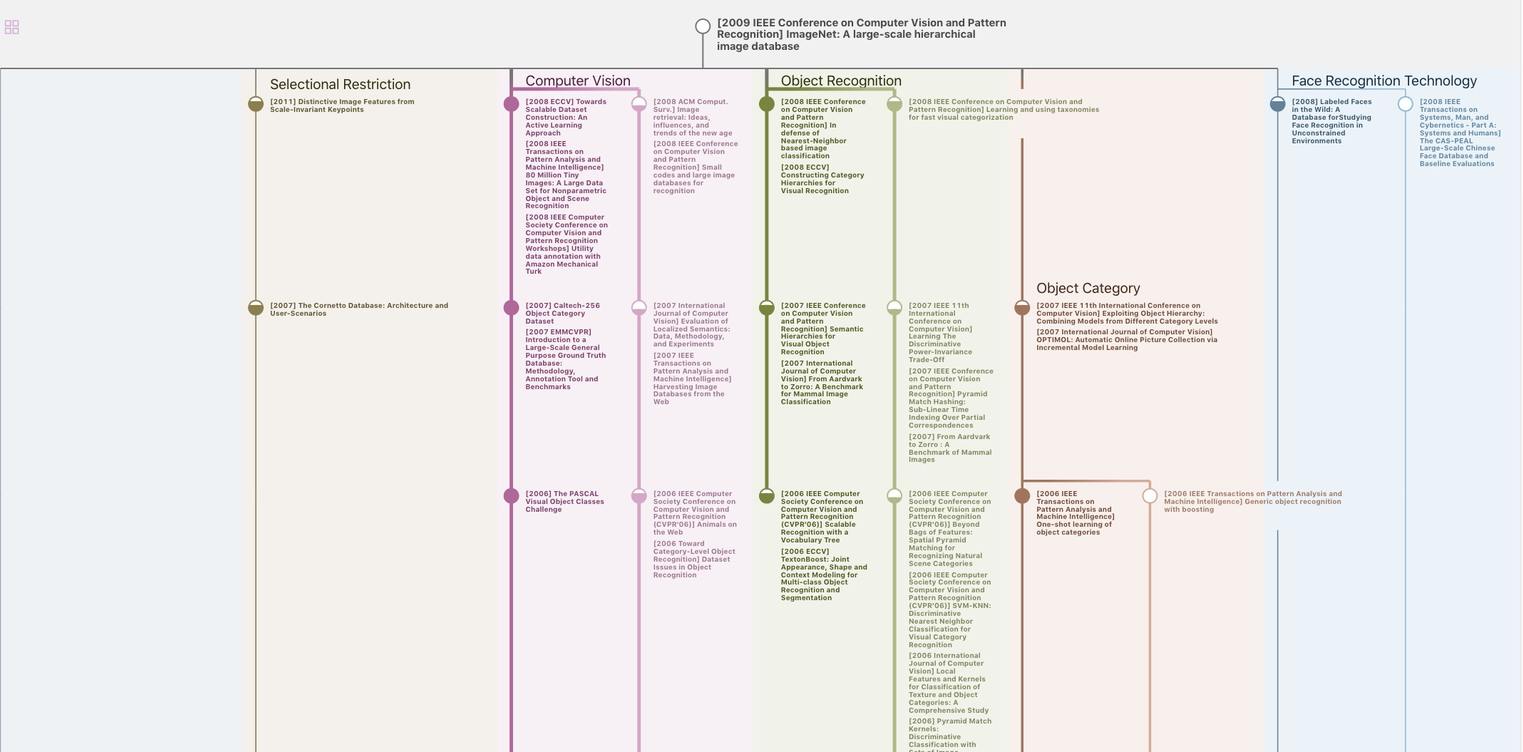
生成溯源树,研究论文发展脉络
Chat Paper
正在生成论文摘要