Stochastic Codebook Regeneration For Sequential Compression Of Continuous Alphabet Sources
2021 IEEE INTERNATIONAL SYMPOSIUM ON INFORMATION THEORY (ISIT)(2021)
摘要
This paper proposes an effective and asymptotically optimal framework for stochastic, adaptive codebook regeneration for sequential ("on the fly") lossy coding of continuous alphabet sources. Earlier work has shown that the rate-distortion bound can be asymptotically achieved for discrete alphabet sources, by a "natural type selection" (NTS) algorithm. At each iteration n, a maximum-likelihood framework is used to estimate the reproduction distribution most likely to generate the empirical types of a sequence of K length-l codewords that respectively "d-match" (i.e., are within distortion d from) a sequence of K length-l source words. The reproduction distribution estimated at iteration n is used to regenerate the codebook for iteration n + 1. The sequence of reproduction distributions was shown to converge, asymptotically in K, n, and l, to the optimal distribution that achieves the rate-distortion bound for discrete alphabet sources. This work generalizes the NTS framework to handle sources over more general (e.g., continuous) alphabet spaces, which often preclude a natural interpretation of the concept of "type". We show, for continuous alphabet sources and fixed block length l, that as K -> infinity and n -> infinity, the sequence of estimated reproduction distributions converges, in the weak convergence sense, to a distribution that achieves the rate-distortion bound, albeit for an auxiliary distortion measure introduced as subterfuge to effectively impose a maximum distortion constraint over K blocks. Leveraging this result, we establish that the sequence of reproduction distributions converges, asymptotically in l, to the optimal codebook reproduction distribution Q* that achieves the rate-distortion bound, with respect to the original distortion measure.
更多查看译文
关键词
optimal distribution,rate-distortion,discrete alphabet sources,NTS framework,continuous alphabet sources,estimated reproduction distributions converges,maximum distortion constraint,stochastic codebook regeneration,effective framework,asymptotically optimal framework,adaptive codebook regeneration,natural type selection algorithm,maximum-likelihood framework,source words,optimal codebook reproduction distribution
AI 理解论文
溯源树
样例
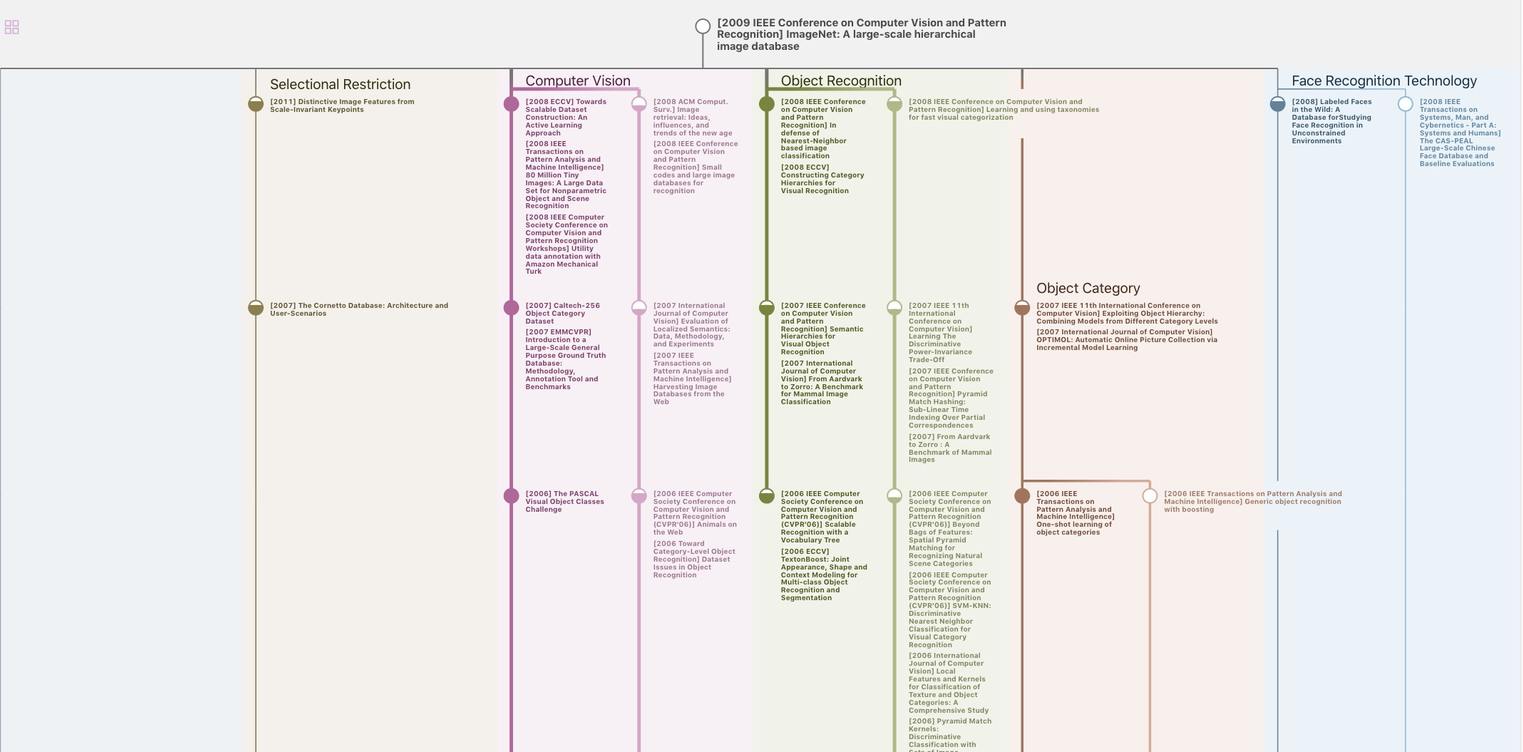
生成溯源树,研究论文发展脉络
Chat Paper
正在生成论文摘要