CEGAR-Tableaux: Improved Modal Satisfiability via Modal Clause-Learning and SAT
AUTOMATED REASONING WITH ANALYTIC TABLEAUX AND RELATED METHODS, TABLEAUX 2021(2021)
摘要
We present CEGAR-Tableaux, a tableaux-like method for propositional modal logics utilising SAT-solvers, modal clause-learning and multiple optimisations from modal and description logic tableaux calculi. We use the standard Counter-example Guided Abstract Refinement (CEGAR) strategy for SAT-solvers to mimic a tableau-like search strategy that explores a rooted tree-model with the classical propositional logic part of each Kripke world evaluated using a SAT-solver. Unlike modal SAT-solvers and modal resolution methods, we do not explicitly represent the accessibility relation but track it implicitly via recursion. By using "satisfiability under unit assumptions", we can iterate rather than "backtrack" over the satisfiable diamonds at the same modal level (context) of the tree model with one SAT-solver. By keeping modal contexts separate from one another, we add further refinements for reflexivity and transitivity which manipulate modal contexts once only. Our solver CEGARBox is, overall, the best for modal logics K, KT and S4 over the standard benchmarks, sometimes by orders of magnitude.
更多查看译文
关键词
improved modal satisfiability,cegar-tableaux,clause-learning
AI 理解论文
溯源树
样例
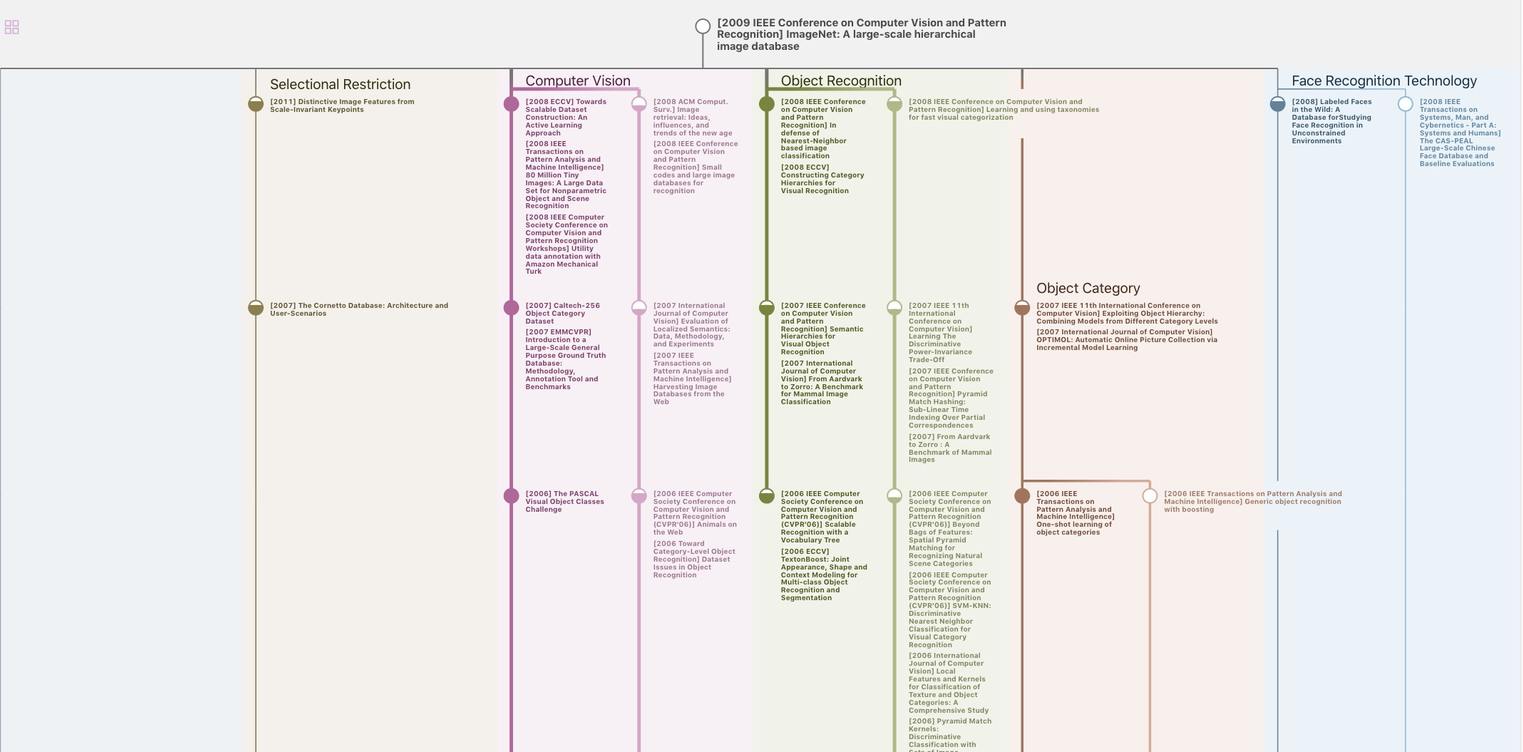
生成溯源树,研究论文发展脉络
Chat Paper
正在生成论文摘要