Multinomial naïve Bayesian classifier with generalized Dirichlet priors for high-dimensional imbalanced data
Periodicals(2021)
摘要
AbstractAbstractThe data-level approach can be used to balance the class distribution in a data set, while generating synthetic instances in a high-dimensional space is an awful task. Naïve Bayesian classifier with multinomial model, called multinomial naïve Bayesian classifier, is a popular classification algorithm for high-dimensional data because of its computational efficiency. However, this algorithm generally cannot have a better performance than the other algorithms on high-dimensional imbalanced data. Generalized Dirichlet distribution that is defined on unit simplex can be priors for multinomial naïve Bayesian classifier. This study proposes methods to find noninformative generalized Dirichlet priors for multinomial naïve Bayesian classifier so that its performance on high-dimensional imbalanced data can be largely improved. The methods are tested on seven high-dimensional imbalanced data sets to demonstrate that the multinomial naïve Bayesian classifier with generalized Dirichlet priors can significantly outperform not only the original multinomial naïve Bayesian classifier, but also random forest and Ripper algorithm.
更多查看译文
关键词
Generalized Dirichlet prior, High-dimensional imbalanced data, Multinomial naive Bayesian classifier
AI 理解论文
溯源树
样例
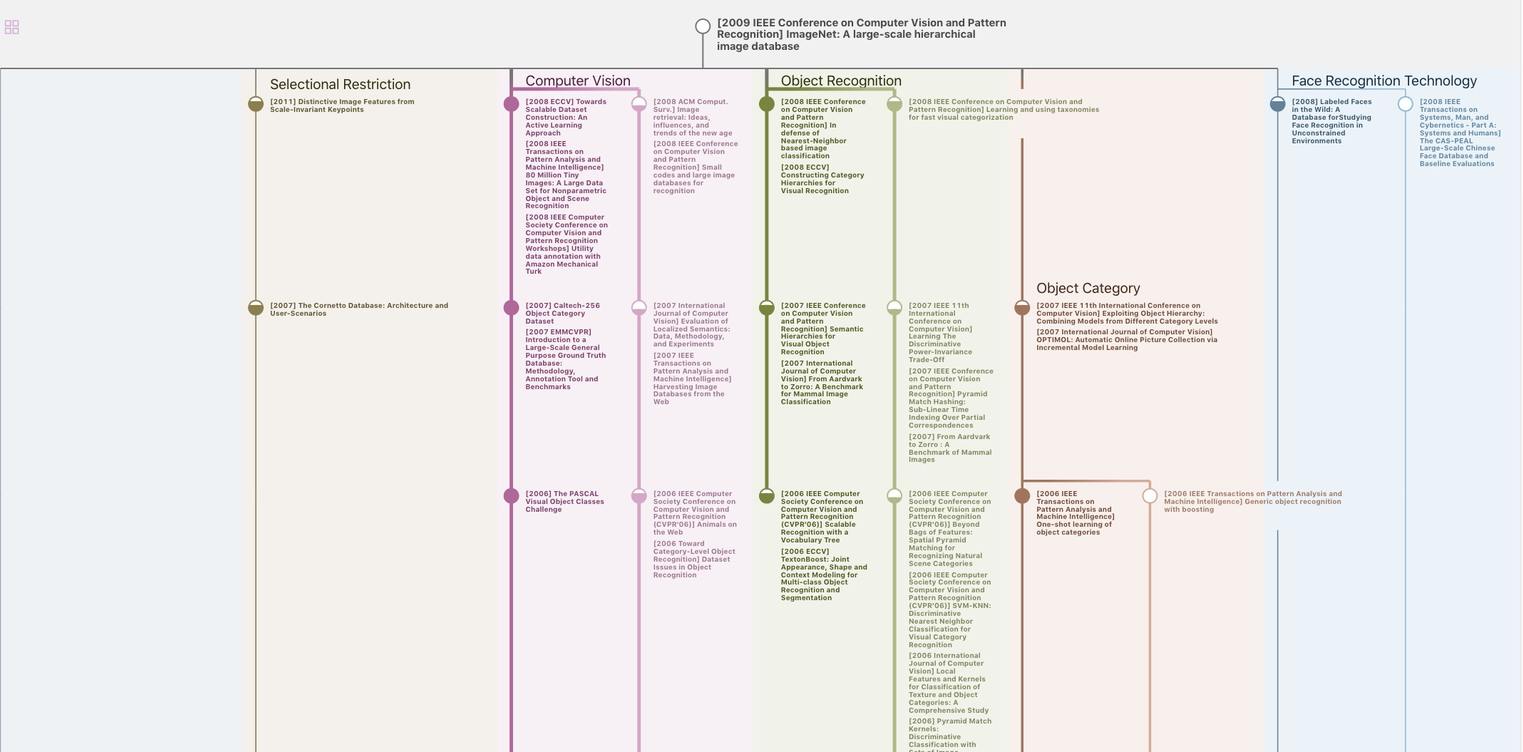
生成溯源树,研究论文发展脉络
Chat Paper
正在生成论文摘要