A two-stage safe screening method for non-convex support vector machine with ramp loss
Periodicals(2021)
摘要
AbstractAbstractSupport vector machine (SVM) is one of the extremely effective classification tools, which has been widely employed in real-world applications. However, it is time-consuming and sensitive to outliers. To address the above two limitations, on the one hand, a variety of safe screening strategies have recently emerged, whose major aim is to accelerate training without sacrificing accuracy. On the other hand, ramp support vector machine (RSVM) remains the sparsity and enhances robustness for outliers by introducing the ramp loss. Unfortunately, due to the fact that ramp loss is non-convex, the existing commonly applied method for solving RSVM is to convert it to a series of convex problems by concave–convex procedure (CCCP), which undoubtedly leads to the increased computational cost. Inspired by the aforementioned researches, we propose a two-stage safe screening method (TSS) for RSVM under the framework of CCCP, which contains the dual screening method with variational inequalities (DVI) and the dynamic screening rule (DSR) based on duality gap. In the first stage, by discarding those redundant samples, DVI reduces the scale of the optimization problem before training and initializes a more accurate feasible solution for the next stage. In the second stage, DSR is embedded into the solver for further acceleration. Finally, a post-checking procedure is conducted to guarantee the safety after the solution is obtained. Numerical experiments on five synthetic datasets and twelve real-world datasets demonstrate the feasibility and efficiency of TSS. Moreover, we extend TSS to other non-convex models and conduct experiments to further verify the validity and safety of our proposed method.
更多查看译文
关键词
Support vector machine, Safe screening, Ramp loss, Variational inequality, Duality gap
AI 理解论文
溯源树
样例
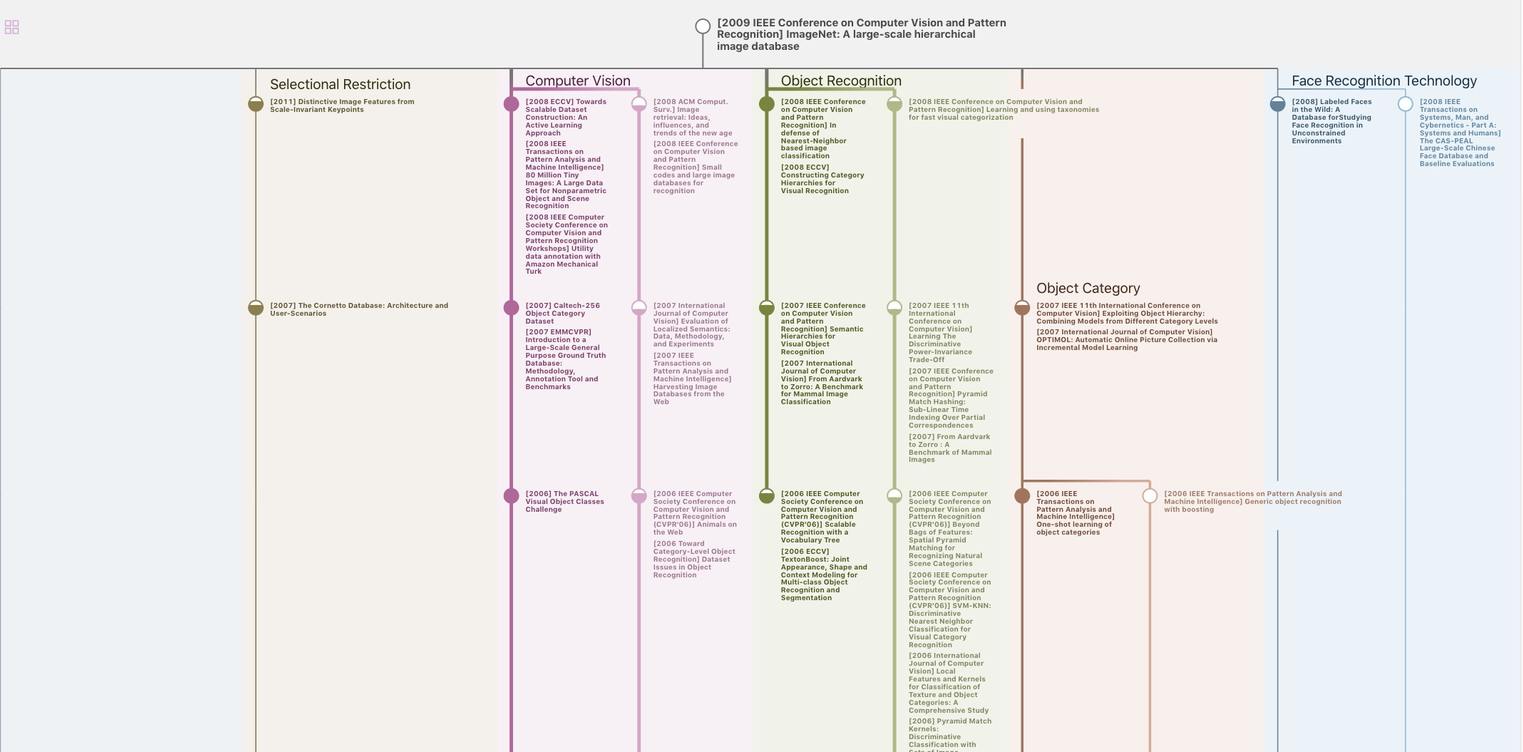
生成溯源树,研究论文发展脉络
Chat Paper
正在生成论文摘要