Ensemble Learning Accurately Predicts The Potential Benefits Of Thrombolytic Therapy In Acute Ischemic Stroke
QUANTITATIVE IMAGING IN MEDICINE AND SURGERY(2021)
摘要
Background: Finding methods to accurately predict the final infarct volumes for acute ischemic stroke patients with full or no recanalization would significantly help to evaluate the potential benefits of thrombolytic therapy. We proposed such a method by constructing a model of ensemble deep learning and machine learning using diffusion-weighted imaging (DWI) only.Methods: The proposed prediction model (named AUNet) combines an adaptive linear ensemble model (ALEM) of machine learning and a deep U-Net network with an accelerated non-local module (U-NL-Net) to learn voxel-wise and spatial features, respectively. Of 40 patients with acute ischemic stroke who received thrombolytic therapy, 17 were fully recanalized, 14 were not recanalized, and nine were partially recanalized. The AUNet was separately trained for full recanalization conditions (AUNetR) and no recanalization (AUNetN) as the best and worst outcomes of thrombolysis, respectively.Results: AUNet performed significantly better in predicting the final infarct volumes in both the recanalization and non-recanalization conditions [area under the receiver operating characteristic curve (AUC) =0.898 +/- 0.022, recanalization; AUC = 0.875 +/- 0.036, non-recanalization: Matthew's correlation coefficient (MCC) =0.863 +/- 0.033, recanalization; MCC =0.851 +/- 0.025, non-recanalization] than the fixedthresholding method (AUC =0.776 +/- 0.021, P<0.0001, recanalization; AUC =0.692 +/- 0.023, P<0.0001, nonrecanalization: MCC =0.742 +/- 0.035, recanalization; MCC =0.671 +/- 0.024, non-recanalization), the logistic regression method (AUC =0.797 +/- 0.023, P<0.003, recanalization; AUC =0.751 +/- 0.030, P<0.003, nonrecanalization: MCC =0.762 +/- 0.035, recanalization; MCC =0.730 +/- 0.031, non-recanalization), and a recently developed convolutional neural network (AUC =0.814 +/- 0.013, P<0.003, recanalization; AUC =0.781 +/- 0.027, P<0.003, non-recanalization: MCC =792 +/- 0.022, recanalization; MCC =0.758 +/- 0.016, non-recanalization). The potential benefit of thrombolysis calculated from AUNetR and AUNetN showed large individual differences (from 12.81% to 239.73%)Conclusions: AUNet improved predictive accuracy over current state-of-the-art methods. More importantly, the accurate prediction of infarct volumes under different recanalization conditions may provide benefitial information for physicians in selecting suitable patients for thrombolytic therapy.
更多查看译文
关键词
Infarct volume prediction, thrombolytic therapy, recanalization, ensemble learning, convolutional neural networks, computer-aided diagnosis
AI 理解论文
溯源树
样例
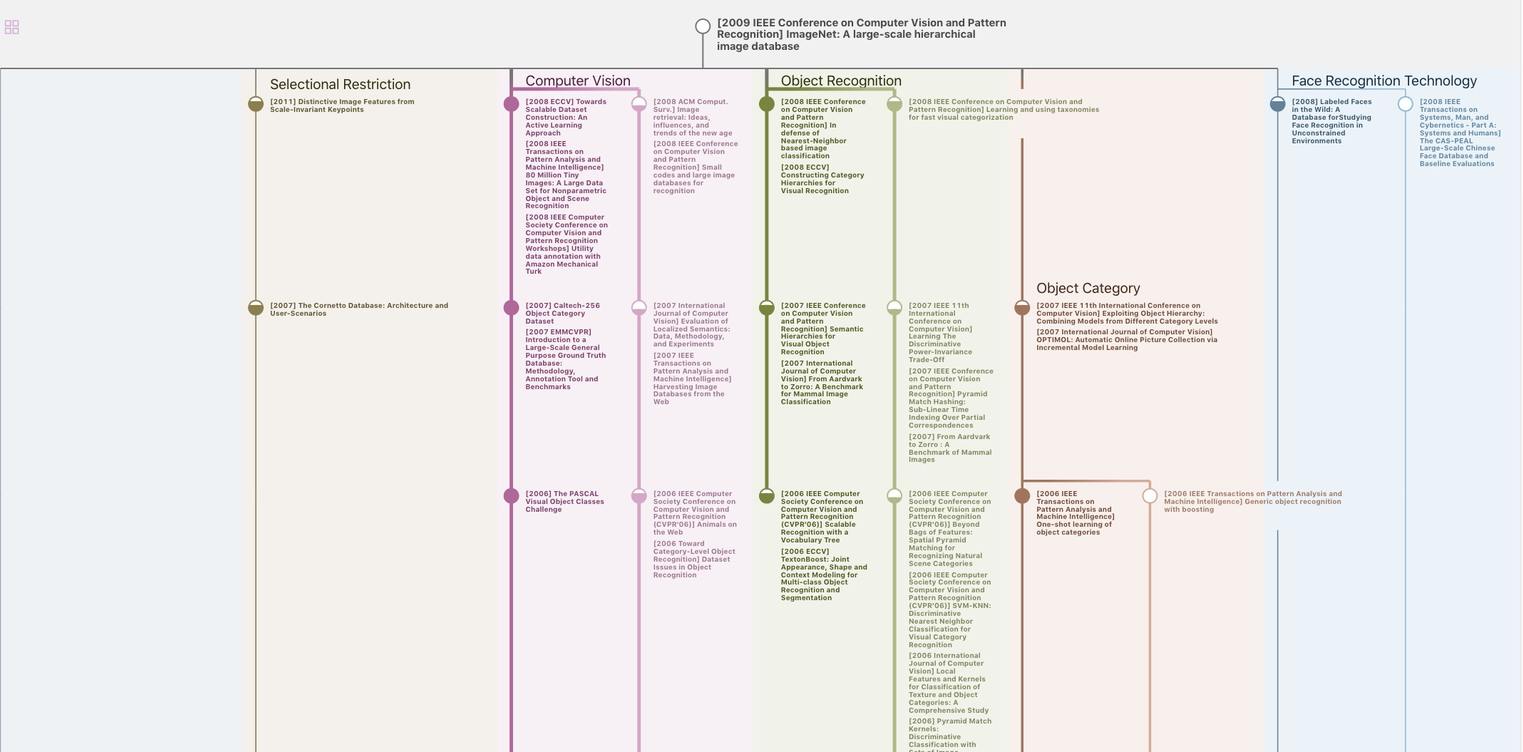
生成溯源树,研究论文发展脉络
Chat Paper
正在生成论文摘要