FBSNet: A Fast Bilateral Symmetrical Network for Real-Time Semantic Segmentation.
arxiv(2023)
摘要
Real-time semantic segmentation, which can be visually understood as the pixel-level classification task on the input image, currently has broad application prospects, especially in the fast-developing fields of autonomous driving and drone navigation. However, the huge burden of calculation together with redundant parameters are still the obstacles to its technological development. In this article, we propose a Fast Bilateral Symmetrical Network (FBSNet) to alleviate the above challenges. Specifically, FBSNet employs a symmetrical encoder-decoder structure with two branches, semantic information branch and spatial detail branch. The Semantic Information Branch (SIB) is the main branch with semantic architecture to acquire the contextual information of the input image and meanwhile acquire sufficient receptive field. While the Spatial Detail Branch (SDB) is a shallow and simple network used to establish local dependencies of each pixel for preserving details, which is essential for restoring the original resolution during the decoding phase. Meanwhile, a Feature Aggregation Module (FAM) is designed to effectively combine the output of these two branches. Experimental results of Cityscapes and CamVid show that the proposed FBSNet can strike a good balance between accuracy and efficiency. Specifically, it obtains 70.9% and 68.9% mIoU along with the inference speed of 90 fps and 120 fps on these two test datasets, respectively, with only 0.62 million parameters on a single RTX 2080Ti GPU. The code is available at https://github.com/IVIPLab/FBSNet.
更多查看译文
关键词
Feature aggregation,local dependencies,real-time,semantic segmentation
AI 理解论文
溯源树
样例
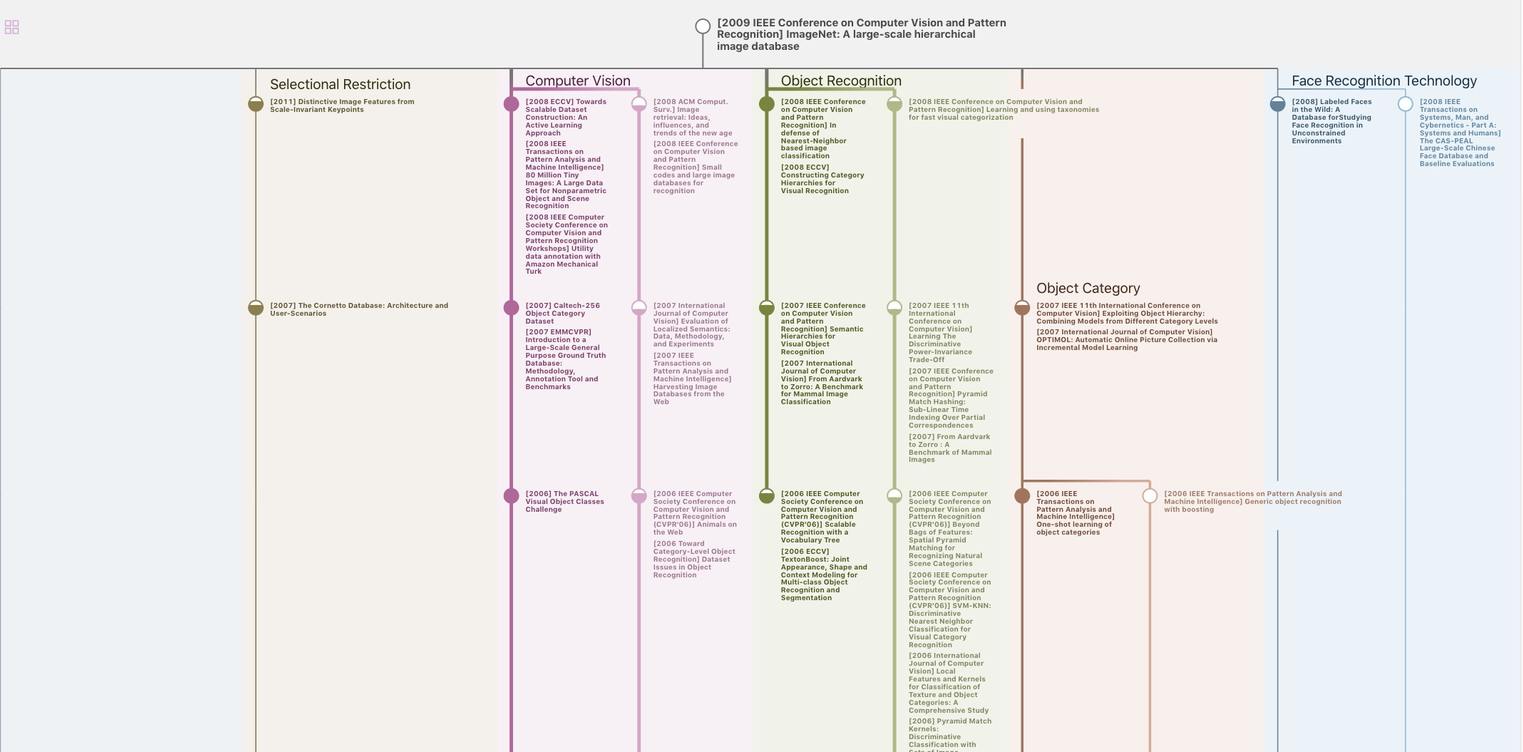
生成溯源树,研究论文发展脉络
Chat Paper
正在生成论文摘要