A gradient sampling algorithm for stratified maps with applications to topological data analysis
arxiv(2023)
摘要
We introduce a novel gradient descent algorithm refining the well-known Gradient Sampling algorithm on the class of stratifiably smooth objective functions, which are defined as locally Lipschitz functions that are smooth on some regular pieces—called the strata—of the ambient Euclidean space. On this class of functions, our algorithm achieves a sub-linear convergence rate. We then apply our method to objective functions based on the (extended) persistent homology map computed over lower-star filters, which is a central tool of Topological Data Analysis. For this, we propose an efficient exploration of the corresponding stratification by using the Cayley graph of the permutation group. Finally, we provide benchmarks and novel topological optimization problems that demonstrate the utility and applicability of our framework.
更多查看译文
关键词
Optimization,Nonsmooth analysis,Persistent homology
AI 理解论文
溯源树
样例
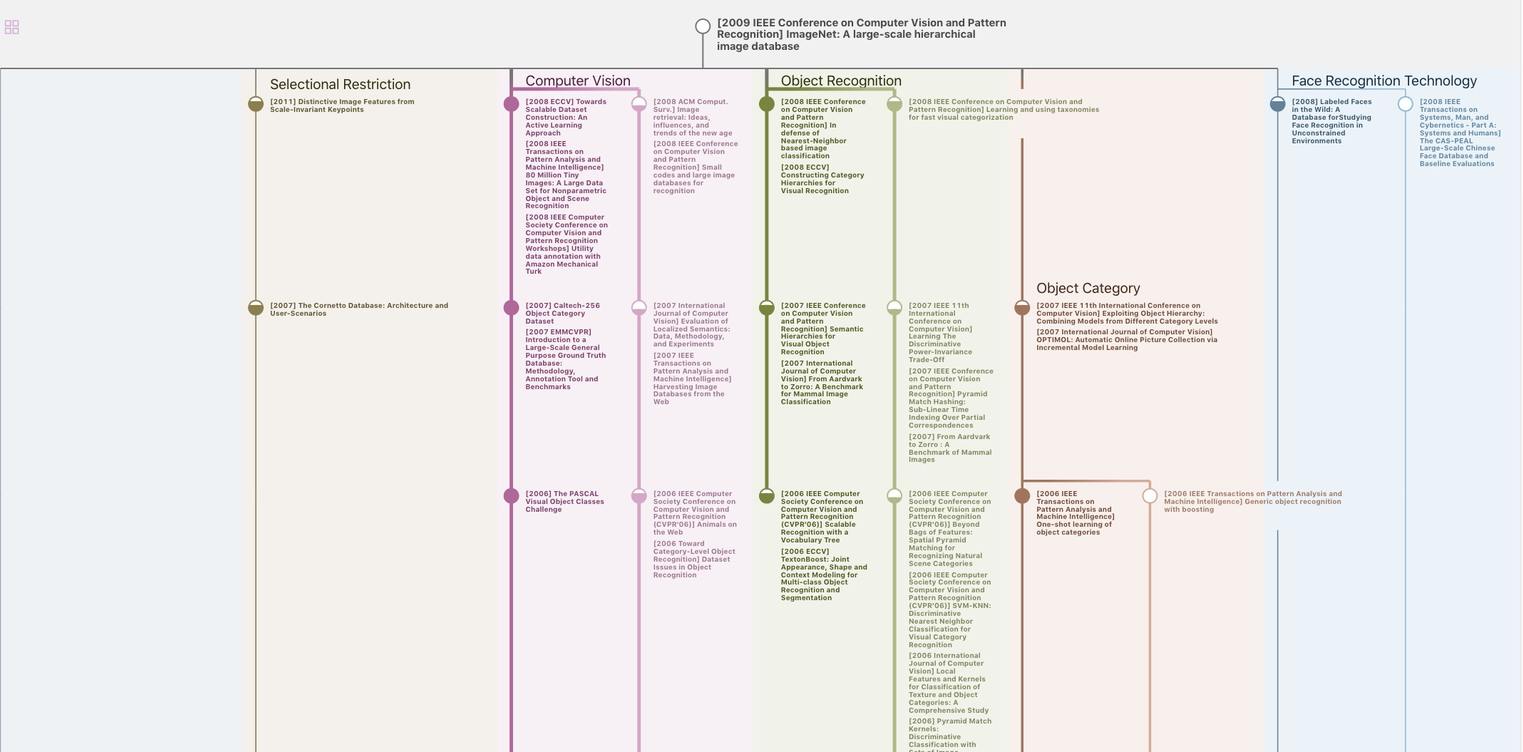
生成溯源树,研究论文发展脉络
Chat Paper
正在生成论文摘要