Unfair! Perceptions of Fairness in Human-Robot Teams
2021 30TH IEEE INTERNATIONAL CONFERENCE ON ROBOT AND HUMAN INTERACTIVE COMMUNICATION (RO-MAN)(2021)
摘要
How team members are treated influences their performance in the team and their desire to be a part of the team in the future. Prior research in human-robot teamwork proposes fairness definitions for human-robot teaming that are based on the work completed by each team member. However, metrics that properly capture people’s perception of fairness in human-robot teaming remains a research gap. We present work on assessing how well objective metrics capture people’s perception of fairness. First, we extend prior fairness metrics based on team members’ capabilities and workload to a bigger team. We also develop a new metric to quantify the amount of time that the robot spends working on the same task as each person. We conduct an online user study (n=95) and show that these metrics align with perceived fairness. Importantly, we discover that there are bleed-over effects in people’s assessment of fairness. When asked to rate fairness based on the amount of time that the robot spends working with each person, participants used two factors (fairness based on the robot’s time and teammates’ capabilities). This bleed-over effect is stronger when people are asked to assess fairness based on capability. From these insights, we propose design guidelines for algorithms to enable robotic teammates to consider fairness in its decision-making to maintain positive team social dynamics and team task performance.
更多查看译文
关键词
team member,human-robot teaming,objective metrics capture people,prior fairness metrics,team members,bigger team,perceived fairness,robotic teammates,positive team social dynamics,human-robot teams,human-robot teamwork,fairness definitions
AI 理解论文
溯源树
样例
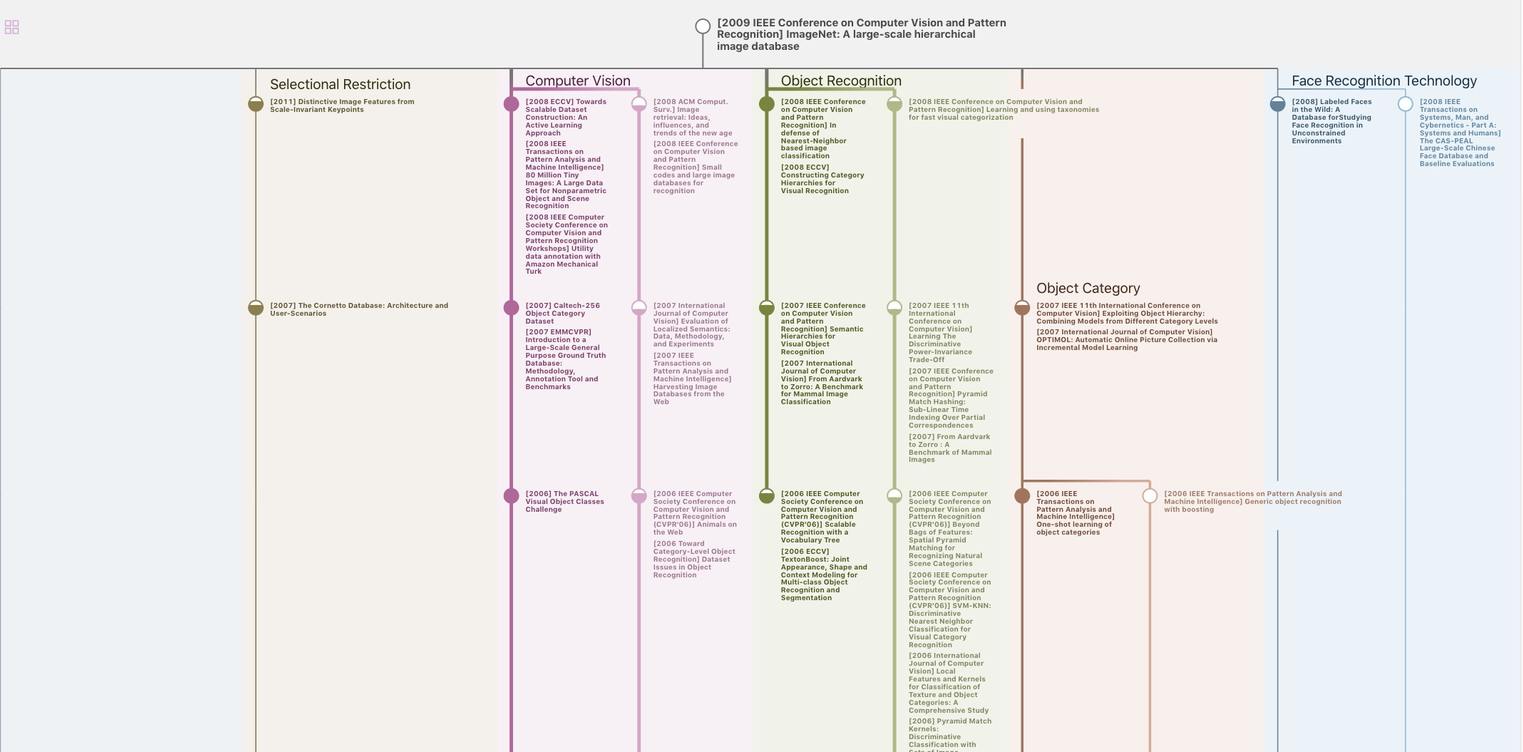
生成溯源树,研究论文发展脉络
Chat Paper
正在生成论文摘要