k-Center Clustering with Outliers in the Sliding-Window Model.
ESA(2021)
摘要
The $k$-center problem for a point set~$P$ asks for a collection of $k$ congruent balls (that is, balls of equal radius) that together cover all the points in $P$ and whose radius is minimized. The $k$-center problem with outliers is defined similarly, except that $z$ of the points in $P$ do need not to be covered, for a given parameter $z$. We study the $k$-center problem with outliers in data streams in the sliding-window model. In this model we are given a possibly infinite stream $P=\langle p_1,p_2,p_3,\ldots\rangle$ of points and a time window of length $W$, and we want to maintain a small sketch of the set $P(t)$ of points currently in the window such that using the sketch we can approximately solve the problem on $P(t)$. We present the first algorithm for the $k$-center problem with outliers in the sliding-window model. The algorithm works for the case where the points come from a space of bounded doubling dimension and it maintains a set $S(t)$ such that an optimal solution on $S(t)$ gives a $(1+\varepsilon)$-approximate solution on $P(t)$. The algorithm is deterministic and uses $O((kz/\varepsilon^d)\log \sigma)$ storage, where $d$ is the doubling dimension of the underlying space and $\sigma$ is the spread of the points in the stream. Algorithms providing a $(1+\varepsilon)$-approximation were not even known in the setting without outliers or in the insertion-only setting with outliers. We also present a lower bound showing that any algorithm that provides a $(1+\varepsilon)$-approximation must use $\Omega((kz/\varepsilon)\log \sigma)$ storage.
更多查看译文
关键词
outliers,sliding-window
AI 理解论文
溯源树
样例
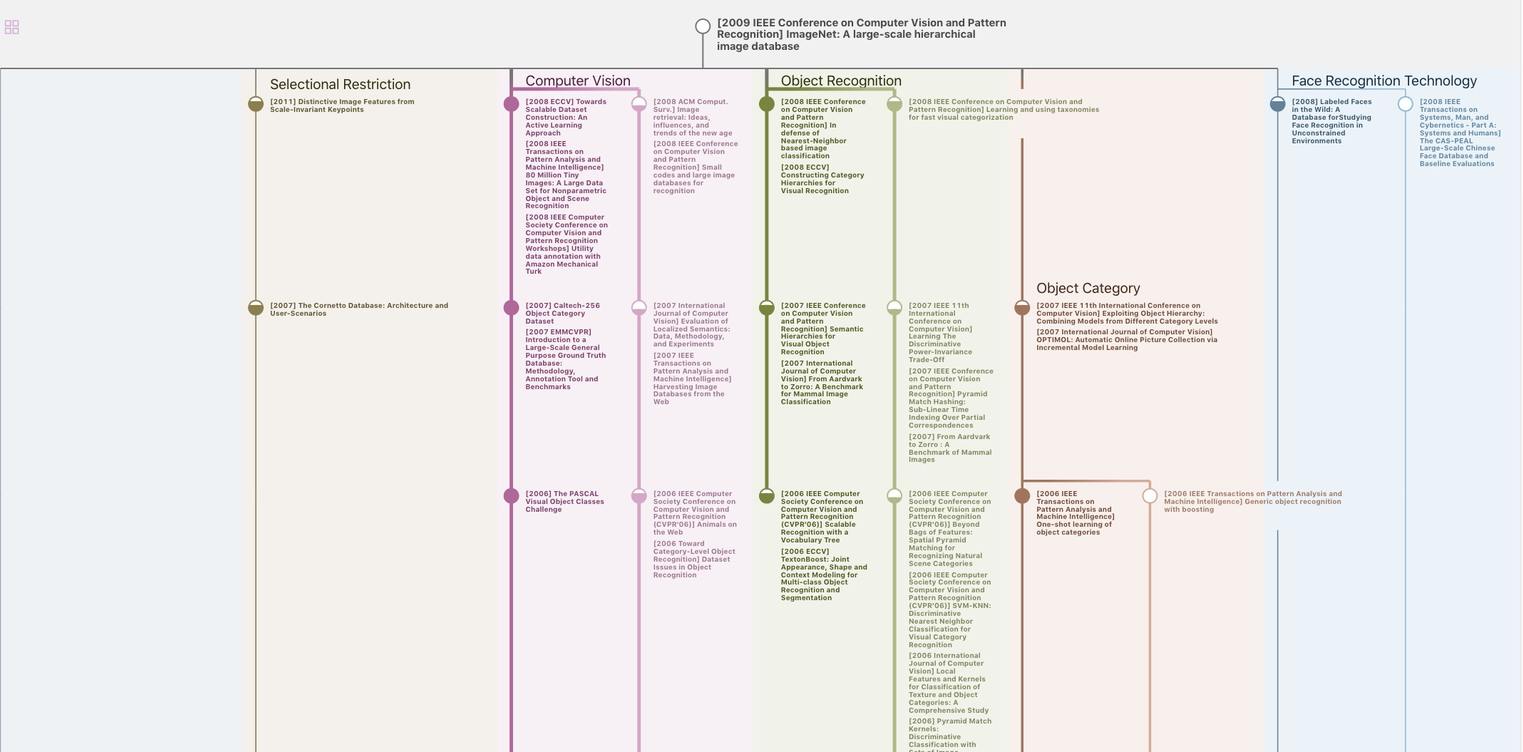
生成溯源树,研究论文发展脉络
Chat Paper
正在生成论文摘要