Combining Magnification and Measurement for Non-Contact Cardiac Monitoring
2021 IEEE/CVF CONFERENCE ON COMPUTER VISION AND PATTERN RECOGITION WORKSHOPS (CVPRW 2021)(2021)
摘要
Deep learning approaches currently achieve the state-of-the-art results on camera-based vital signs measurement. One of the main challenges with using neural models for these applications is the lack of sufficiently large and diverse datasets. Limited data increases the chances of overfitting models to the available data which in turn can harm generalization. In this paper, we show that the generalizability of imaging photoplethysmography models can be improved by augmenting the training set with "magnified" videos. These augmentations are specifically designed to reveal useful features for recovering the photoplethysmogram. We show that using augmentations of this form is more effective at improving model robustness than other commonly used data augmentation approaches. We show better within-dataset and especially cross-dataset performance with our proposed data augmentation approach on three publicly available datasets.
更多查看译文
关键词
diverse datasets,overfitting models,generalizability,imaging photoplethysmography models,augmentations,model robustness,within-dataset,cross-dataset performance,data augmentation approach,noncontact cardiac,camera-based vital signs measurement,neural models,sufficiently large datasets
AI 理解论文
溯源树
样例
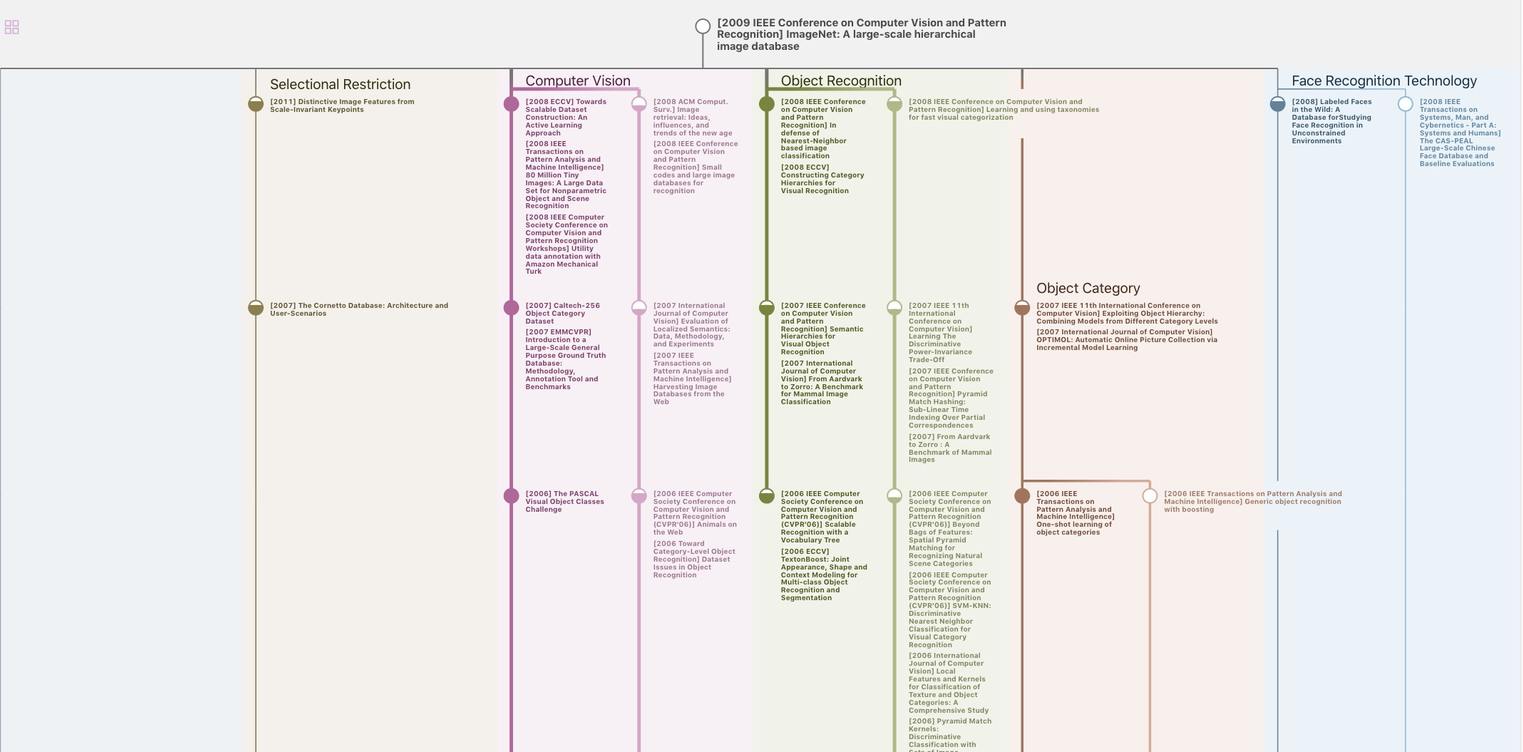
生成溯源树,研究论文发展脉络
Chat Paper
正在生成论文摘要