End-to-End Learned Image Compression with Augmented Normalizing Flows
2021 IEEE/CVF CONFERENCE ON COMPUTER VISION AND PATTERN RECOGITION WORKSHOPS (CVPRW 2021)(2021)
摘要
This paper presents a new attempt at using augmented normalizing flows (ANF) for lossy image compression. ANF is a specific type of normalizing flow models that augment the input with an independent noise, allowing a smoother transformation from the augmented input space to the latent space. Inspired by the fact that ANF can offer greater expressivity by stacking multiple variational autoencoders (VAE), we generalize the popular VAE-based compression framework by the autoencoding transforms of ANF. When evaluated on Kodak dataset, our ANF-based model provides 3.4% higher BD-rate saving as compared with a VAE-based baseline that implements hyper-prior with mean prediction. Interestingly, it benefits even more from the incorporation of a post-processing network, showing 11.8% rate saving as compared to 6.0% with the baseline plus post-processing.
更多查看译文
关键词
augmented normalizing flows,lossy image compression,normalizing flow models,smoother transformation,augmented input space,latent space,multiple variational autoencoders,popular VAE-based compression framework,autoencoding transforms,ANF-based model,VAE-based baseline
AI 理解论文
溯源树
样例
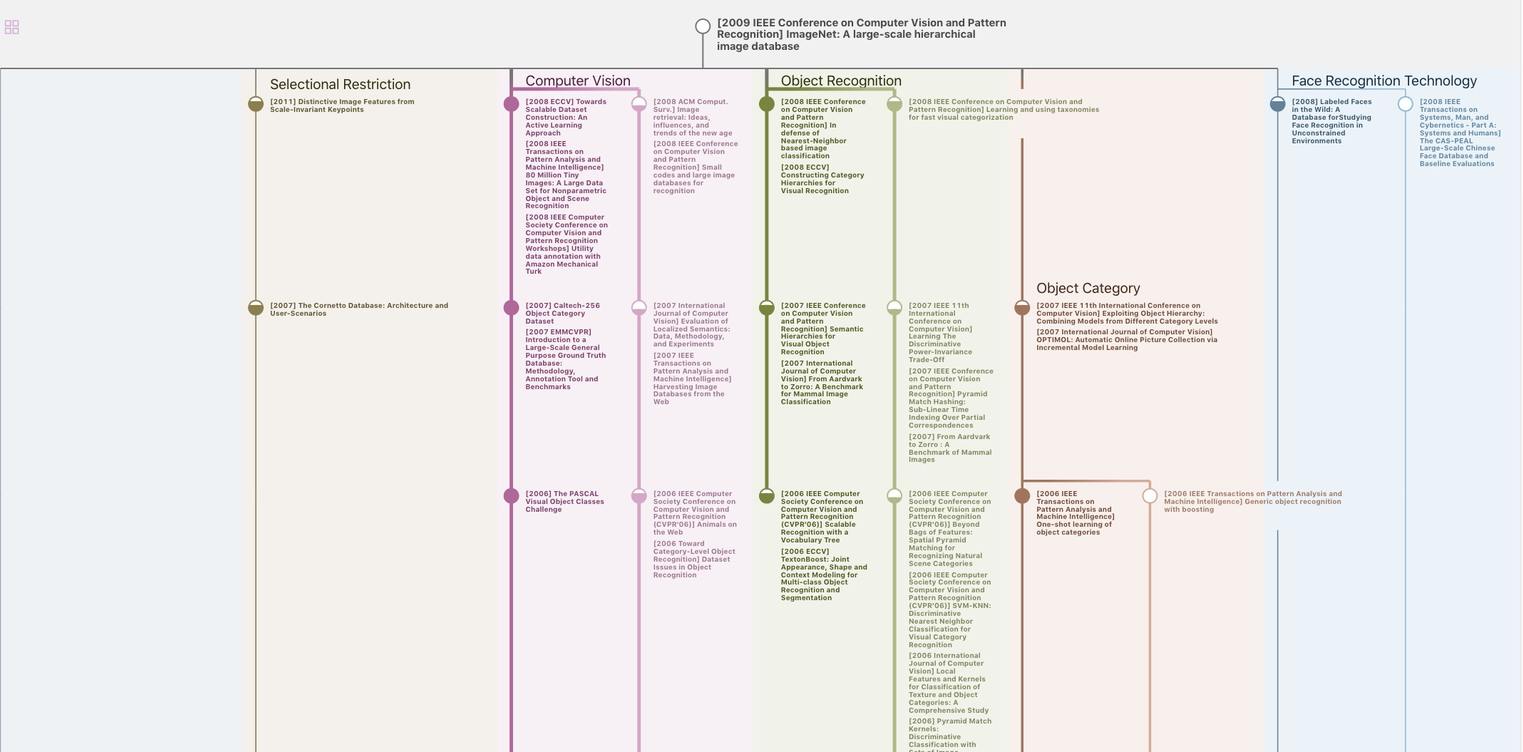
生成溯源树,研究论文发展脉络
Chat Paper
正在生成论文摘要