Learning to Detect Phone-related Pedestrian Distracted Behaviors with Synthetic Data
2021 IEEE/CVF CONFERENCE ON COMPUTER VISION AND PATTERN RECOGITION WORKSHOPS (CVPRW 2021)(2021)
摘要
Due to the popularity and mobility of smart phones, phone-related pedestrian distracted behaviors, e.g., Texting, Game Playing, and Phone calls, have caused many traffic fatalities and accidents. As an advanced driver-assistance or autonomous-driving system, computer vision could be used to automatically detect distractions from cameras installed on the vehicle for useful safety intervention. The state-of-the-art method models this problem as a standard supervised learning method with a two-branch Convolutional Neural Network (CNN) followed by a voting on all image frames. In contrast, this paper proposes a new synthetic dataset named SYN-PPDB (448 synchronized video pairs of 53,760 computer game images) for this research problem and models it as a transfer learning problem from synthetic data to real data. A new deep learning model embedded with spatial-temporal feature learning and pose-aware transfer learning is proposed. Experimental results show that we could improve the state-of-the-art overall recognition accuracy from 84.27% to 96.67%.
更多查看译文
关键词
smart phones,advanced driver-assistance,computer vision,standard supervised learning method,two-branch convolutional neural network,synthetic dataset,transfer learning problem,deep learning model,spatial-temporal feature learning,pose-aware transfer learning,phone-related pedestrian distracted behaviors,autonomous-driving system,computer game images,synchronized video pairs
AI 理解论文
溯源树
样例
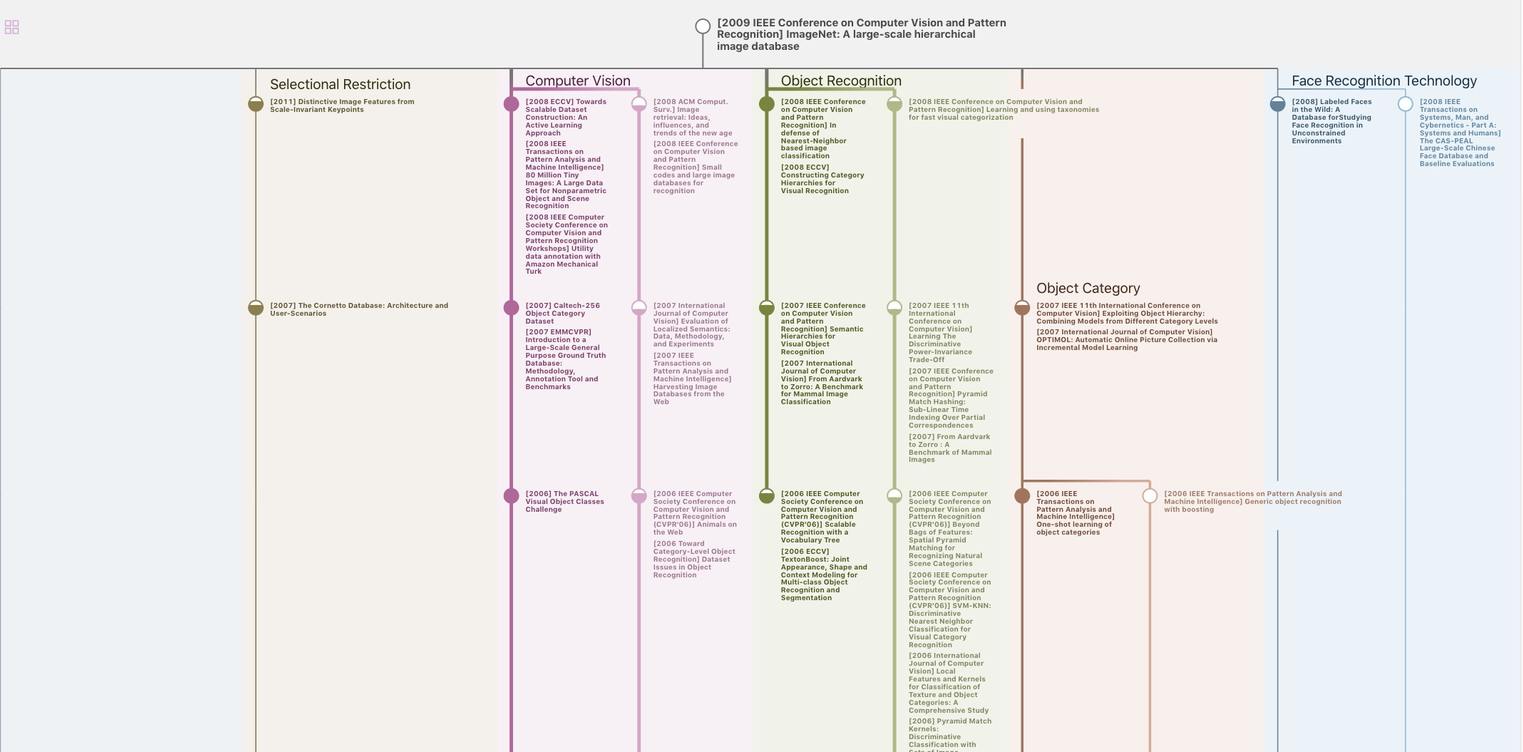
生成溯源树,研究论文发展脉络
Chat Paper
正在生成论文摘要