Explainable Noisy Label Flipping for Multi-Label Fashion Image Classification
2021 IEEE/CVF CONFERENCE ON COMPUTER VISION AND PATTERN RECOGITION WORKSHOPS (CVPRW 2021)(2021)
摘要
In online shopping applications, the daily insertion of new products requires an overwhelming annotation effort. Usually done by humans, it comes at a huge cost and yet generates high rates of noisy/missing labels that seriously hinder the effectiveness of CNNs in multi-label classification. We propose SELF-ML, a classification framework that exploits the relation between visual attributes and appearance together with the "low-rank" nature of the feature space. It learns a sparse reconstruction of image features as a convex combination of very few images - a basis - that are correctly annotated. Building on this representation, SELF-ML has a module that relabels noisy annotations from the derived combination of the clean data. Due to such structured reconstruction, SELF-ML gives an explanation of its label-flipping decisions. Experiments on a real-world shopping dataset show that SELF-ML significantly increases the number of correct labels even with few clean annotations.
更多查看译文
关键词
multilabel classification,SELF-ML,classification framework,visual attributes,low-rank nature,feature space,sparse reconstruction,image features,convex combination,noisy annotations,label-flipping decisions,real-world shopping dataset show,correct labels,clean annotations,explainable noisy label flipping,multilabel fashion image classification,online shopping applications,daily insertion,overwhelming annotation effort,huge cost
AI 理解论文
溯源树
样例
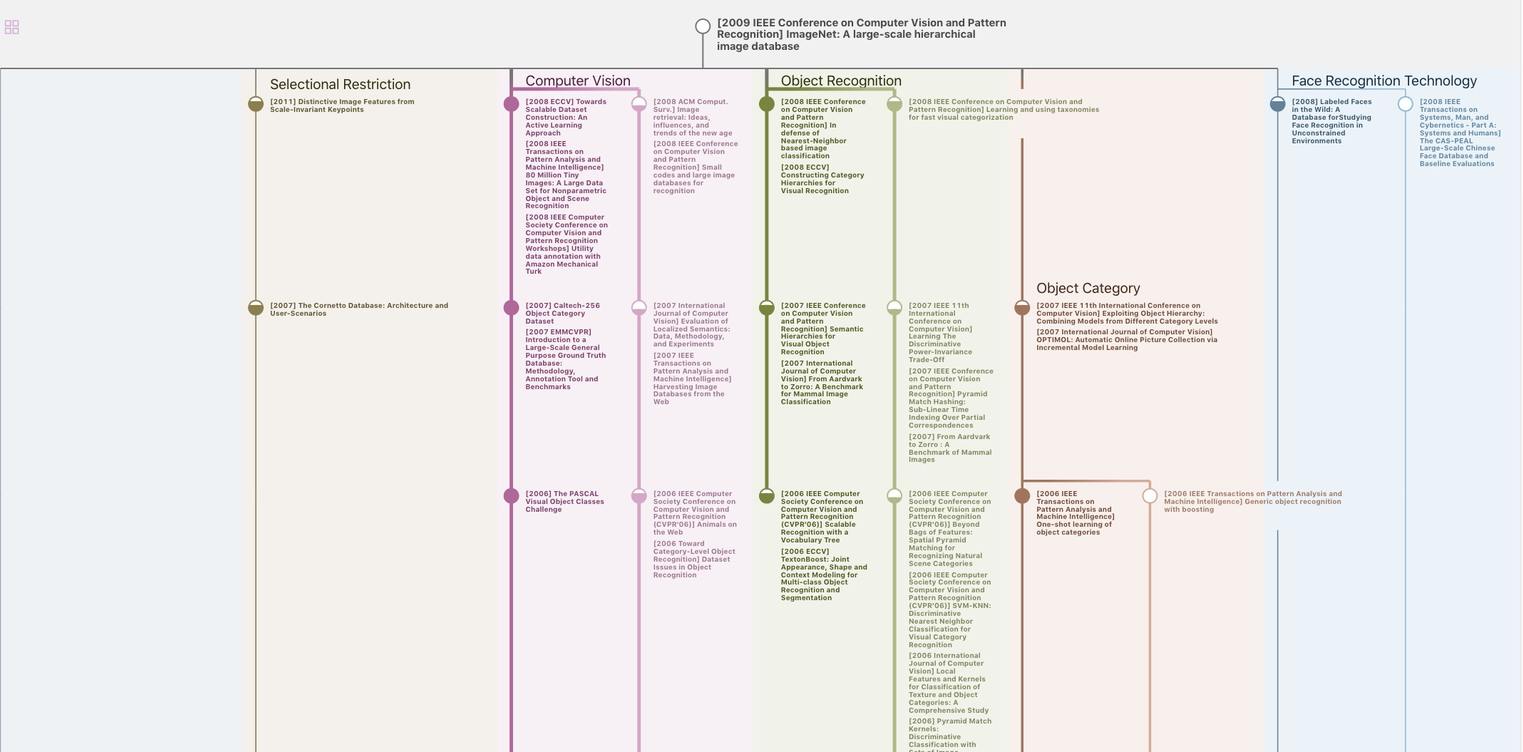
生成溯源树,研究论文发展脉络
Chat Paper
正在生成论文摘要