CLIP-Art: Contrastive Pre-training for Fine-Grained Art Classification
2021 IEEE/CVF CONFERENCE ON COMPUTER VISION AND PATTERN RECOGITION WORKSHOPS (CVPRW 2021)(2021)
摘要
Existing computer vision research in artwork struggles with artwork's fine-grained attributes recognition and lack of curated annotated datasets due to their costly creation. In this work, we use CLIP (Contrastive Language-Image Pre-Training) [12] for training a neural network on a variety of art images and text pairs, being able to learn directly from raw descriptions about images, or if available, curated labels. Model's zero-shot capability allows predicting the most relevant natural language description for a given image, without directly optimizing for the task. Our approach aims to solve 2 challenges: instance retrieval and fine-grained artwork attribute recognition. We use the iMet Dataset [20], which we consider the largest annotated artwork dataset. Our code and models will be available at https://github.com/KeremTurgutlu/clip_art
更多查看译文
关键词
CLIP-art,fine-grained art classification,computer vision research,artwork struggles,curated annotated datasets,costly creation,neural network,art images,text pairs,raw descriptions,available labels,curated labels,zero-shot capability,relevant natural language description,fine-grained artwork attribute recognition,largest annotated artwork dataset,contrastive language-image pre-training,iMet Dataset
AI 理解论文
溯源树
样例
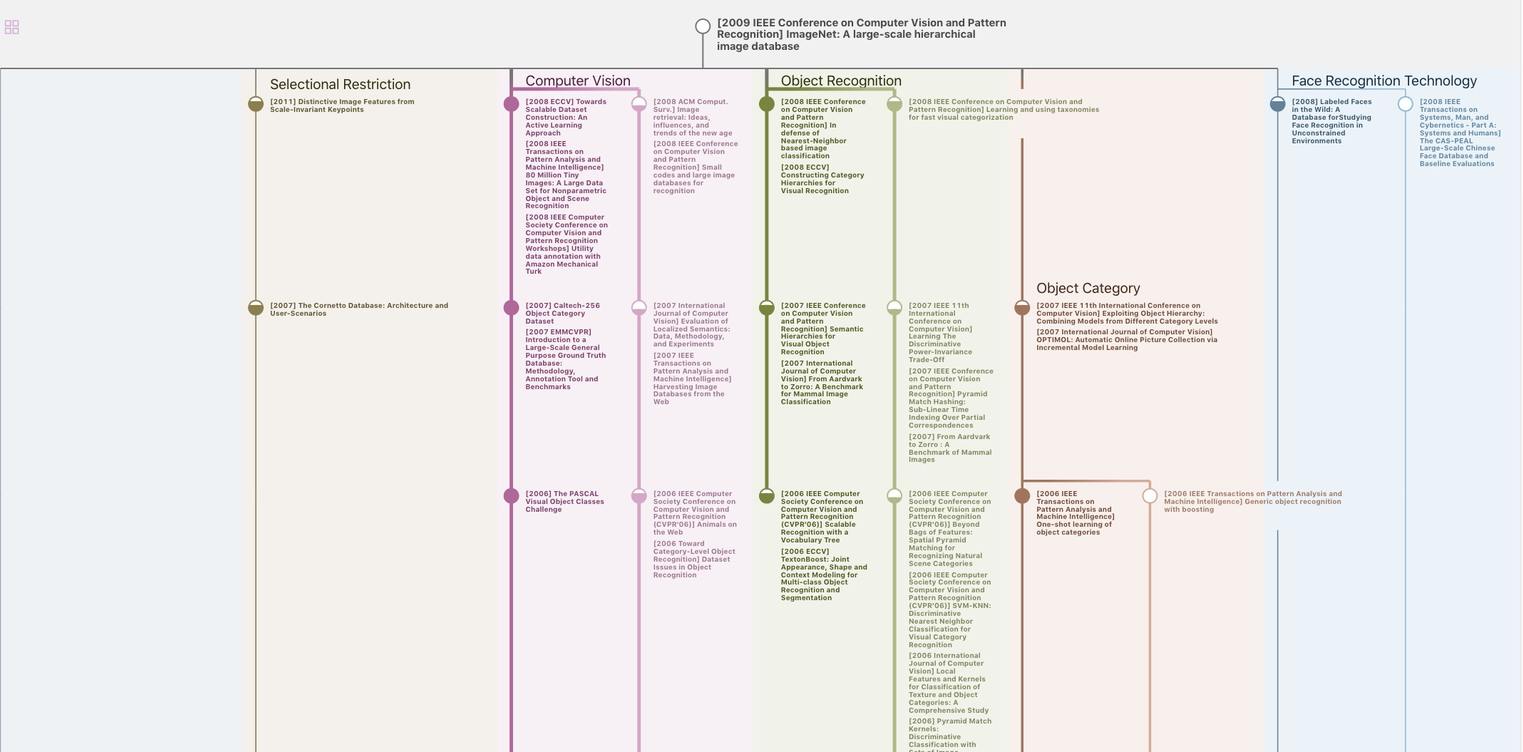
生成溯源树,研究论文发展脉络
Chat Paper
正在生成论文摘要