Exploring deep learning methods for recognizing rare diseases and their clinical manifestations from texts
BMC Bioinformatics(2022)
摘要
Background and objective Although rare diseases are characterized by low prevalence, approximately 400 million people are affected by a rare disease. The early and accurate diagnosis of these conditions is a major challenge for general practitioners, who do not have enough knowledge to identify them. In addition to this, rare diseases usually show a wide variety of manifestations, which might make the diagnosis even more difficult. A delayed diagnosis can negatively affect the patient’s life. Therefore, there is an urgent need to increase the scientific and medical knowledge about rare diseases. Natural Language Processing (NLP) and Deep Learning can help to extract relevant information about rare diseases to facilitate their diagnosis and treatments. Methods The paper explores several deep learning techniques such as Bidirectional Long Short Term Memory (BiLSTM) networks or deep contextualized word representations based on Bidirectional Encoder Representations from Transformers (BERT) to recognize rare diseases and their clinical manifestations (signs and symptoms). Results BioBERT, a domain-specific language representation based on BERT and trained on biomedical corpora, obtains the best results with an F1 of 85.2% for rare diseases. Since many signs are usually described by complex noun phrases that involve the use of use of overlapped, nested and discontinuous entities, the model provides lower results with an F1 of 57.2%. Conclusions While our results are promising, there is still much room for improvement, especially with respect to the identification of clinical manifestations (signs and symptoms).
更多查看译文
关键词
Rare diseases,Named entity recognition,Deep learning
AI 理解论文
溯源树
样例
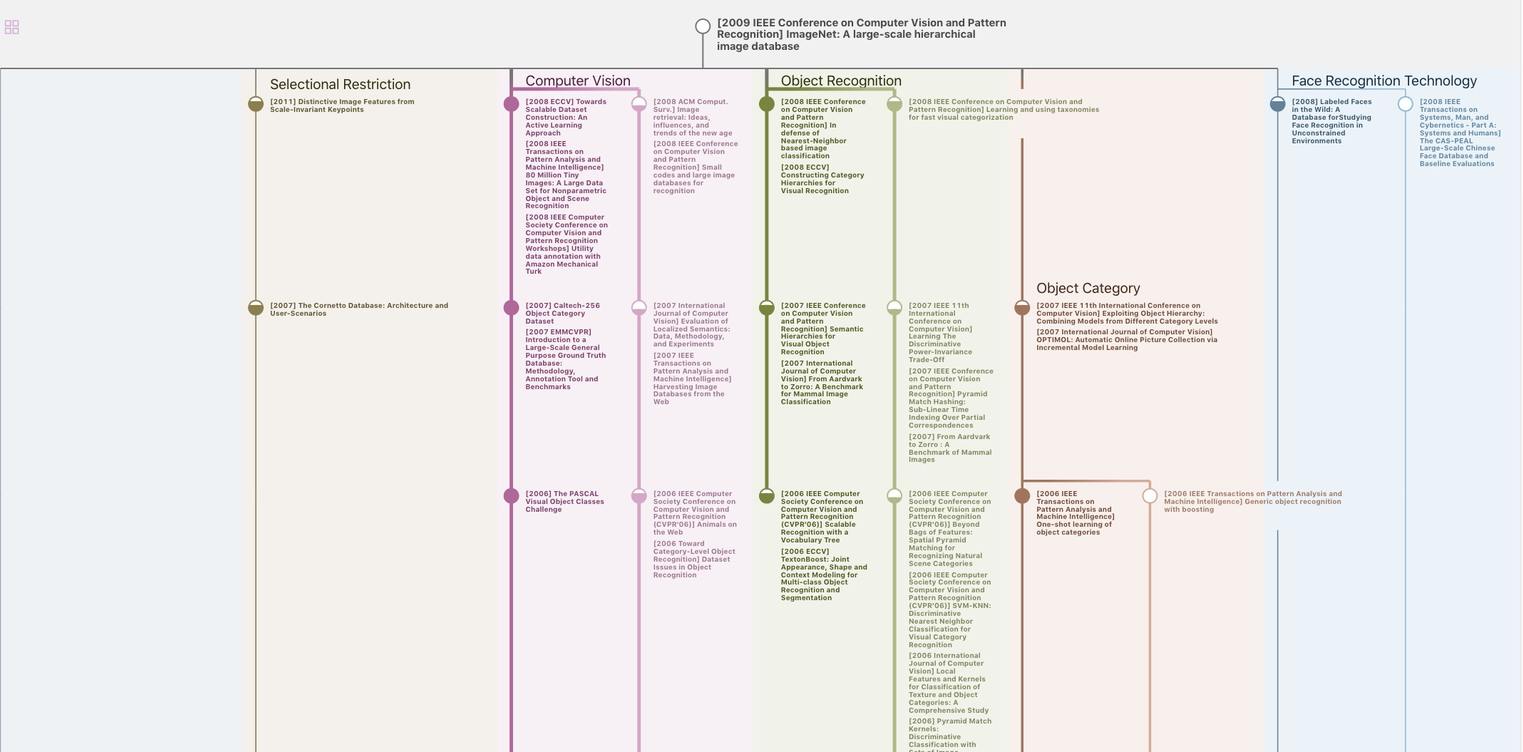
生成溯源树,研究论文发展脉络
Chat Paper
正在生成论文摘要