The Impact of Reinitialization on Generalization in Convolutional Neural Networks
arxiv(2021)
摘要
Recent results suggest that reinitializing a subset of the parameters of a neural network during training can improve generalization, particularly for small training sets. We study the impact of different reinitialization methods in several convolutional architectures across 12 benchmark image classification datasets, analyzing their potential gains and highlighting limitations. We also introduce a new layerwise reinitialization algorithm that outperforms previous methods and suggest explanations of the observed improved generalization. First, we show that layerwise reinitialization increases the margin on the training examples without increasing the norm of the weights, hence leading to an improvement in margin-based generalization bounds for neural networks. Second, we demonstrate that it settles in flatter local minima of the loss surface. Third, it encourages learning general rules and discourages memorization by placing emphasis on the lower layers of the neural network. Our takeaway message is that the accuracy of convolutional neural networks can be improved for small datasets using bottom-up layerwise reinitialization, where the number of reinitialized layers may vary depending on the available compute budget.
更多查看译文
关键词
convolutional neural networks,generalization,reinitialization,neural networks
AI 理解论文
溯源树
样例
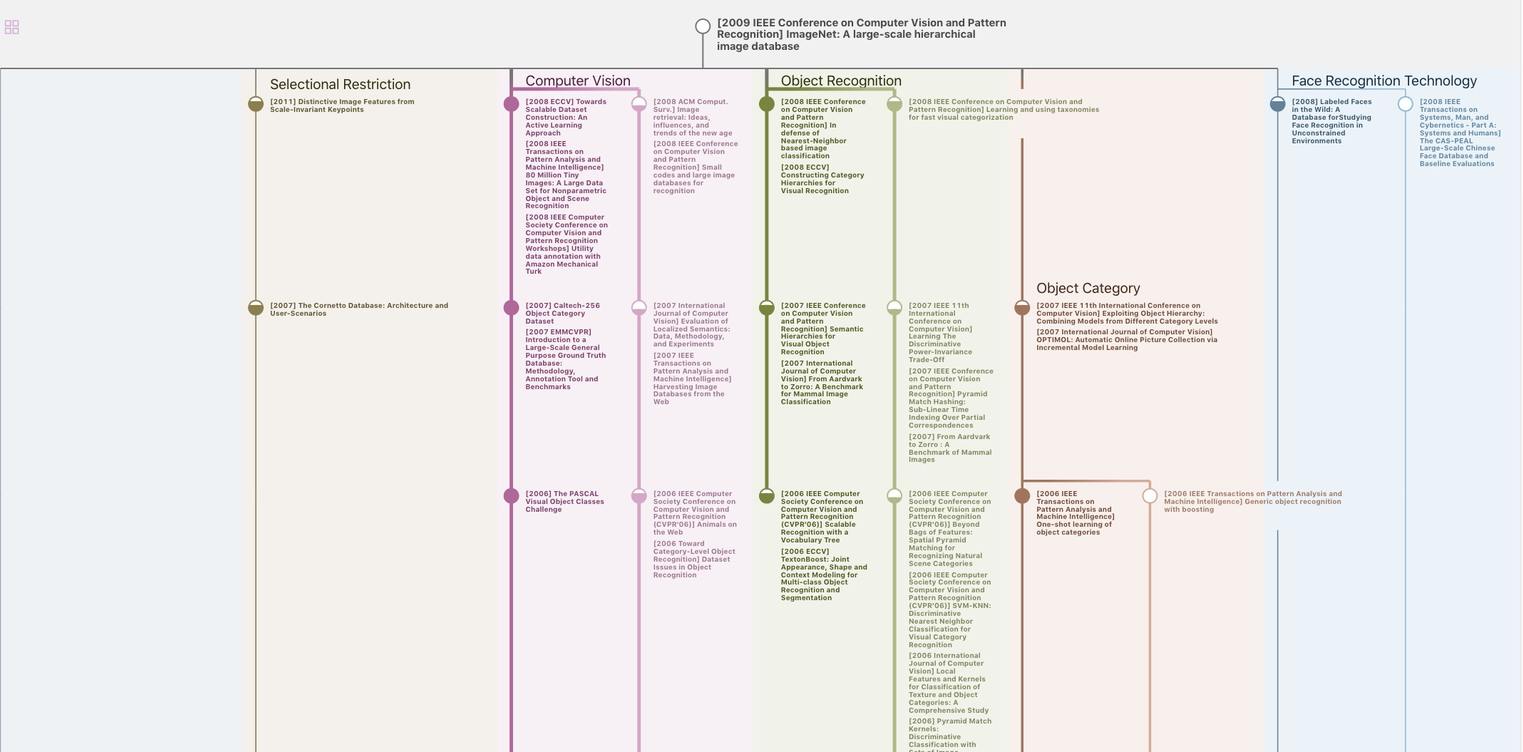
生成溯源树,研究论文发展脉络
Chat Paper
正在生成论文摘要