OptAGAN: Entropy-based finetuning on text VAE-GAN
arxiv(2021)
摘要
Transfer learning through large pre-trained models has changed the landscape of current applications in natural language processing (NLP). Recently Optimus, a variational autoencoder (VAE) which combines two pre-trained models, BERT and GPT-2, has been released, and its combination with generative adversial networks (GANs) has been shown to produce novel, yet very human-looking text. The Optimus and GANs combination avoids the troublesome application of GANs to the discrete domain of text, and prevents the exposure bias of standard maximum likelihood methods. We combine the training of GANs in the latent space, with the finetuning of the decoder of Optimus for single word generation. This approach lets us model both the high-level features of the sentences, and the low-level word-by-word generation. We finetune using reinforcement learning (RL) by exploiting the structure of GPT-2 and by adding entropy-based intrinsically motivated rewards to balance between quality and diversity. We benchmark the results of the VAE-GAN model, and show the improvements brought by our RL finetuning on three widely used datasets for text generation, with results that greatly surpass the current state-of-the-art for the quality of the generated texts.
更多查看译文
关键词
text,finetuning,entropy-based,vae-gan
AI 理解论文
溯源树
样例
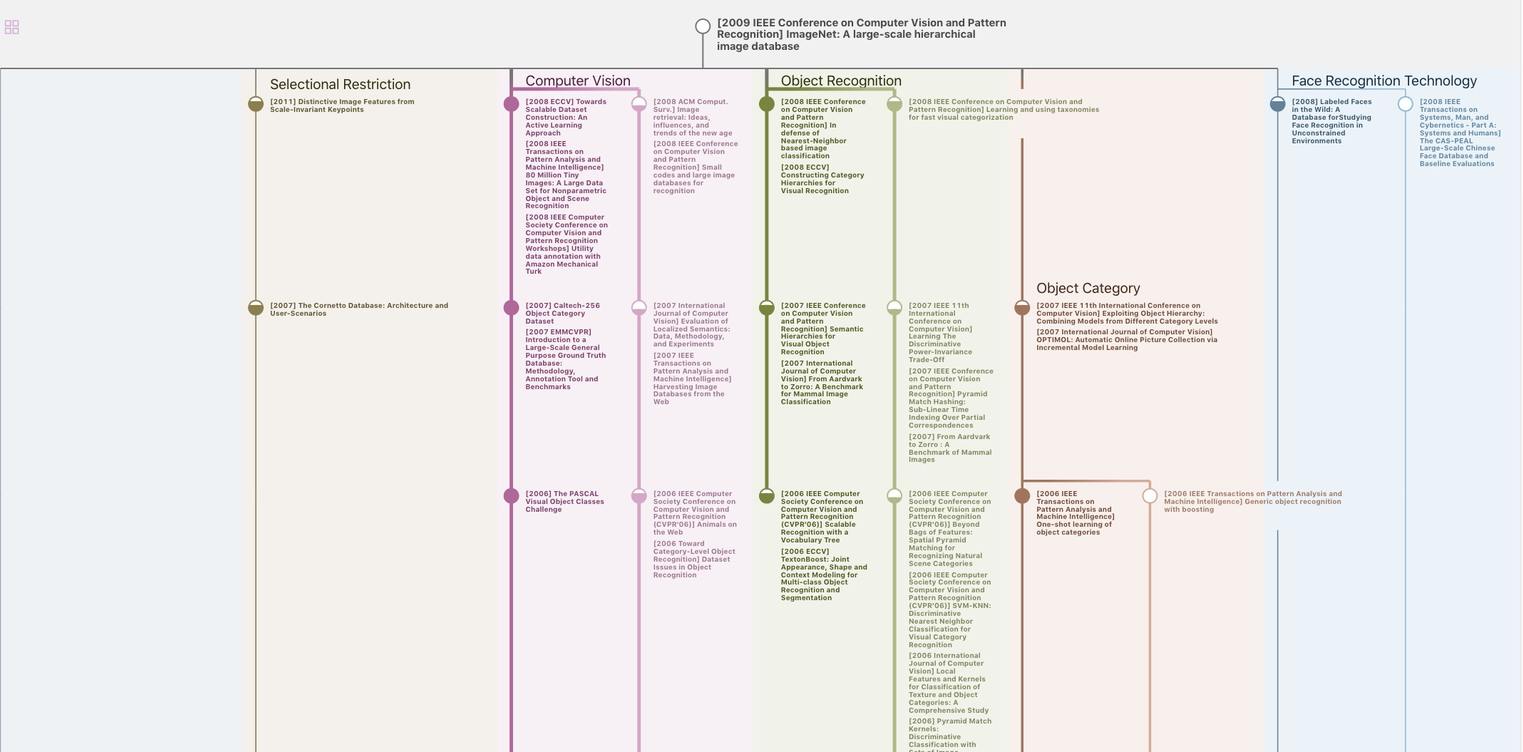
生成溯源树,研究论文发展脉络
Chat Paper
正在生成论文摘要