Multitask Learning for Visual Question Answering
IEEE Transactions on Neural Networks and Learning Systems(2023)
摘要
Visual question answering (VQA) is a task that machines should provide an accurate natural language answer given an image and a question about the image. Many studies have found that the current VQA methods are heavily driven by the surface correlation or statistical bias in the training data, and lack sufficient image grounding. To address this issue, we devise a novel end-to-end architecture that uses multitask learning to promote more sufficient image grounding and learn effective multimodality representations. The tasks consist of VQA and our proposed image cloze (IC) task requires machines to fill in the blanks accurately given an image and a textual description of the image. To ensure our model performs sufficient image grounding as much as possible, we propose a novel word-masking algorithm to develop the multimodal IC task based on the part-of-speech of words. Our model predicts the VQA answer and fills in the blanks after the multimodality representation learning that is shared by the two tasks. Experimental results show that our model achieves almost the equivalent, state-of-the-art, second-best performance on the VQA v2.0, VQA-changing priors (CP) v2, and grounded question answering (GQA) datasets, respectively, with fewer parameters and without additional data compared with baselines.
更多查看译文
关键词
Information fusion,multimodality fusion,multitask learning,visual question answering (VQA)
AI 理解论文
溯源树
样例
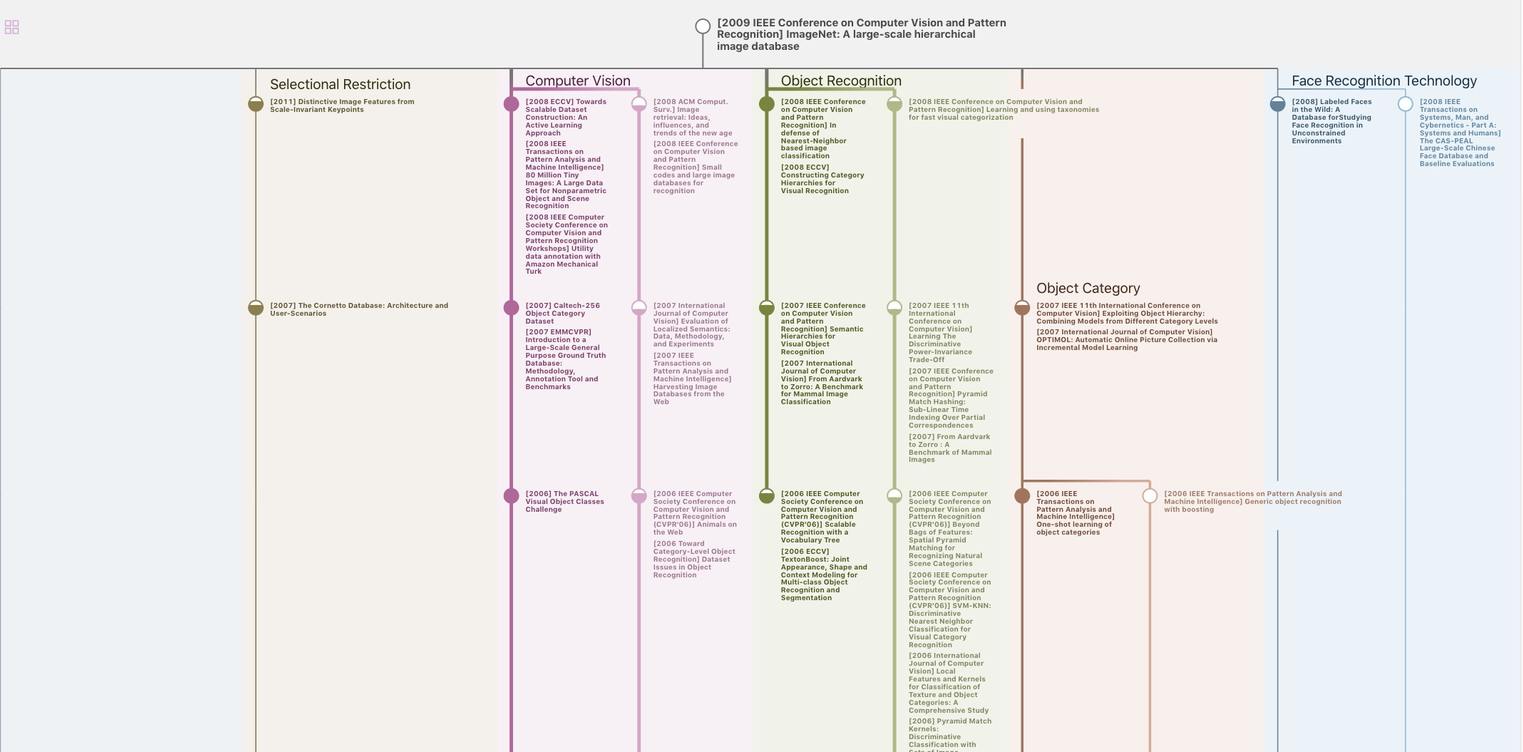
生成溯源树,研究论文发展脉络
Chat Paper
正在生成论文摘要