Maximum F1-Score Training for End-to-End Mispronunciation Detection and Diagnosis of L2 English Speech
IEEE International Conference on Multimedia and Expo (ICME)(2022)
摘要
End-to-end (E2E) neural models are increasingly attracting attention as a promising modeling approach for mispronunciation detection and diagnosis (MDD). Typically, these models are trained by optimizing a cross-entropy criterion, which corresponds to improving the log-likelihood of the training data. However, there is a discrepancy between the objectives of model training and the MDD evaluation, since the performance of an MDD model is commonly evaluated in terms of F1-score instead of phone or word error rate (PER/WER). In view of this, we in this paper explore the use of a discriminative objective function for training E2E MDD models, which aims to maximize the expected F1-score directly. A series of experiments conducted on the L2-ARCTIC dataset show that our proposed method can yield considerable performance improvements in relation to some state-of-the-art E2E MDD approaches and the celebrated GOP method.
更多查看译文
关键词
mispronunciation detection and diagnosis (MDD),computer-assisted pronunciation training (CAPT),maximum F1-score training,end-to-end model
AI 理解论文
溯源树
样例
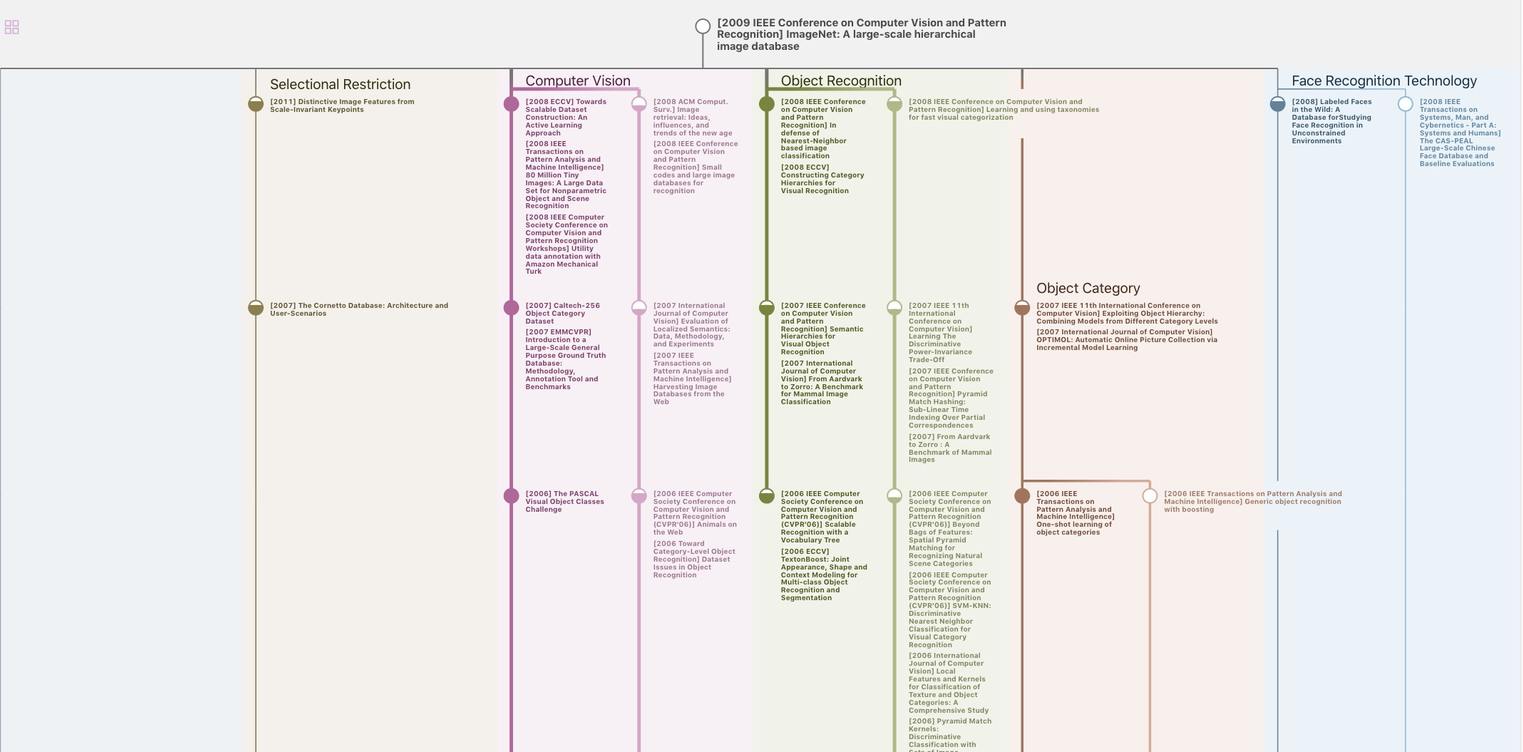
生成溯源树,研究论文发展脉络
Chat Paper
正在生成论文摘要