Differentiable Prompt Makes Pre-trained Language Models Better Few-shot Learners.
International Conference on Learning Representations (ICLR)(2022)
摘要
Large-scale pre-trained language models have contributed significantly to natural language processing by demonstrating remarkable abilities as few-shot learners. However, their effectiveness depends mainly on scaling the model parameters and prompt design, hindering their implementation in most real-world applications. This study proposes a novel pluggable, extensible, and efficient approach named DifferentiAble pRompT (DART), which can convert small language models into better few-shot learners without any prompt engineering. The main principle behind this approach involves reformulating potential natural language processing tasks into the task of a pre-trained language model and differentially optimizing the prompt template as well as the target label with backpropagation. Furthermore, the proposed approach can be: (i) Plugged to any pre-trained language models; (ii) Extended to widespread classification tasks. A comprehensive evaluation of standard NLP tasks demonstrates that the proposed approach achieves a better few-shot performance.
更多查看译文
关键词
prompt-tuning,pre-trained language model,few-shot learning
AI 理解论文
溯源树
样例
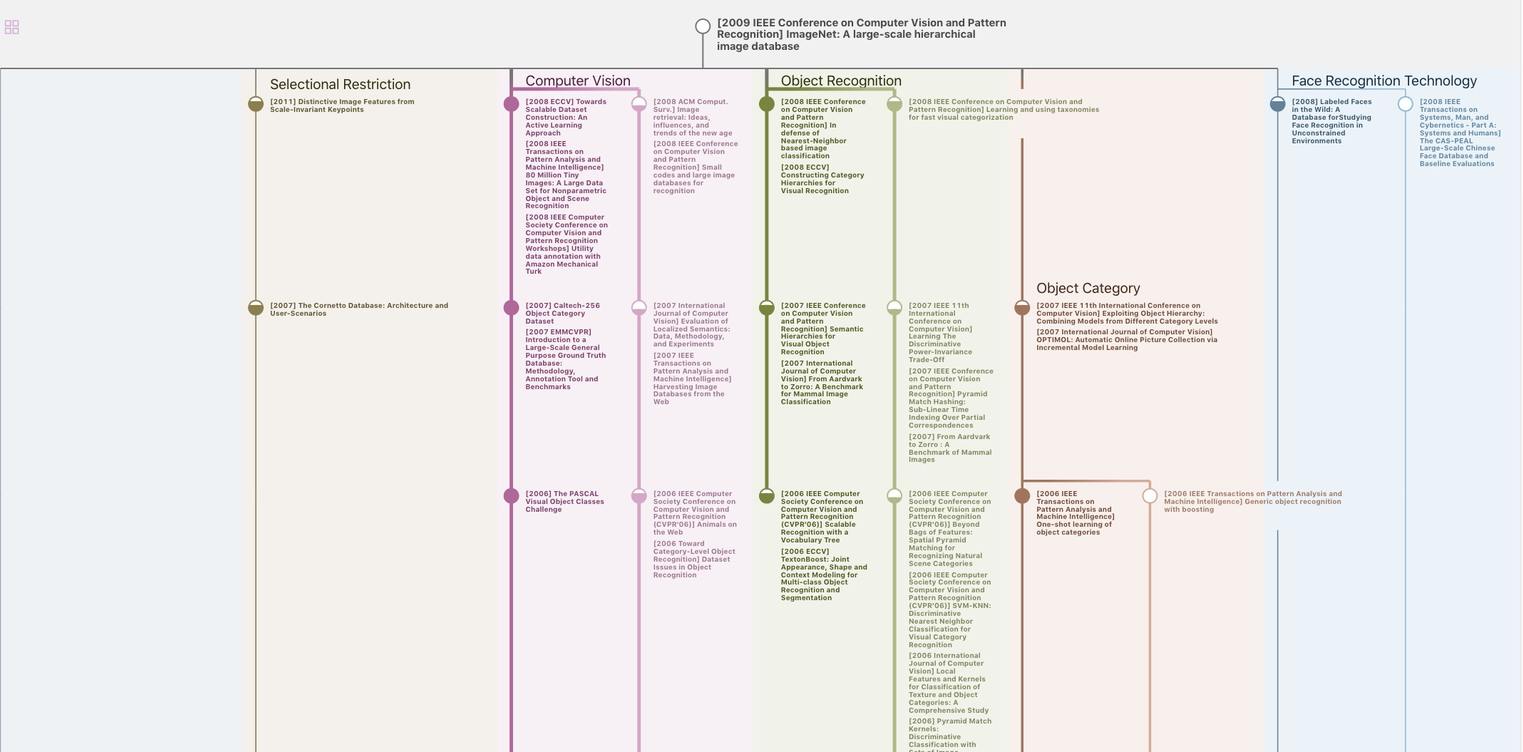
生成溯源树,研究论文发展脉络
Chat Paper
正在生成论文摘要