Neural Network Gaussian Processes by Increasing Depth
IEEE TRANSACTIONS ON NEURAL NETWORKS AND LEARNING SYSTEMS(2024)
摘要
Recent years have witnessed an increasing interest in the correspondence between infinitely wide networks and Gaussian processes. Despite the effectiveness and elegance of the current neural network Gaussian process theory, to the best of our knowledge, all the neural network Gaussian processes (NNGPs) are essentially induced by increasing width. However, in the era of deep learning, what concerns us more regarding a neural network is its depth as well as how depth impacts the behaviors of a network. Inspired by a width-depth symmetry consideration, we use a shortcut network to show that increasing the depth of a neural network can also give rise to a Gaussian process, which is a valuable addition to the existing theory and contributes to revealing the true picture of deep learning. Beyond the proposed Gaussian process by depth, we theoretically characterize its uniform tightness property and the smallest eigenvalue of the Gaussian process kernel. These characterizations can not only enhance our understanding of the proposed depth-induced Gaussian process but also pave the way for future applications. Lastly, we examine the performance of the proposed Gaussian process by regression experiments on two benchmark datasets.
更多查看译文
关键词
Artificial neural networks,Gaussian processes,Deep learning,Neurons,Kernel,Topology,Network topology,Deep neural networks,generalized central limit theorem,neural network Gaussian processes (NNGPs),smallest eigenvalue,uniform tightness,weak dependence
AI 理解论文
溯源树
样例
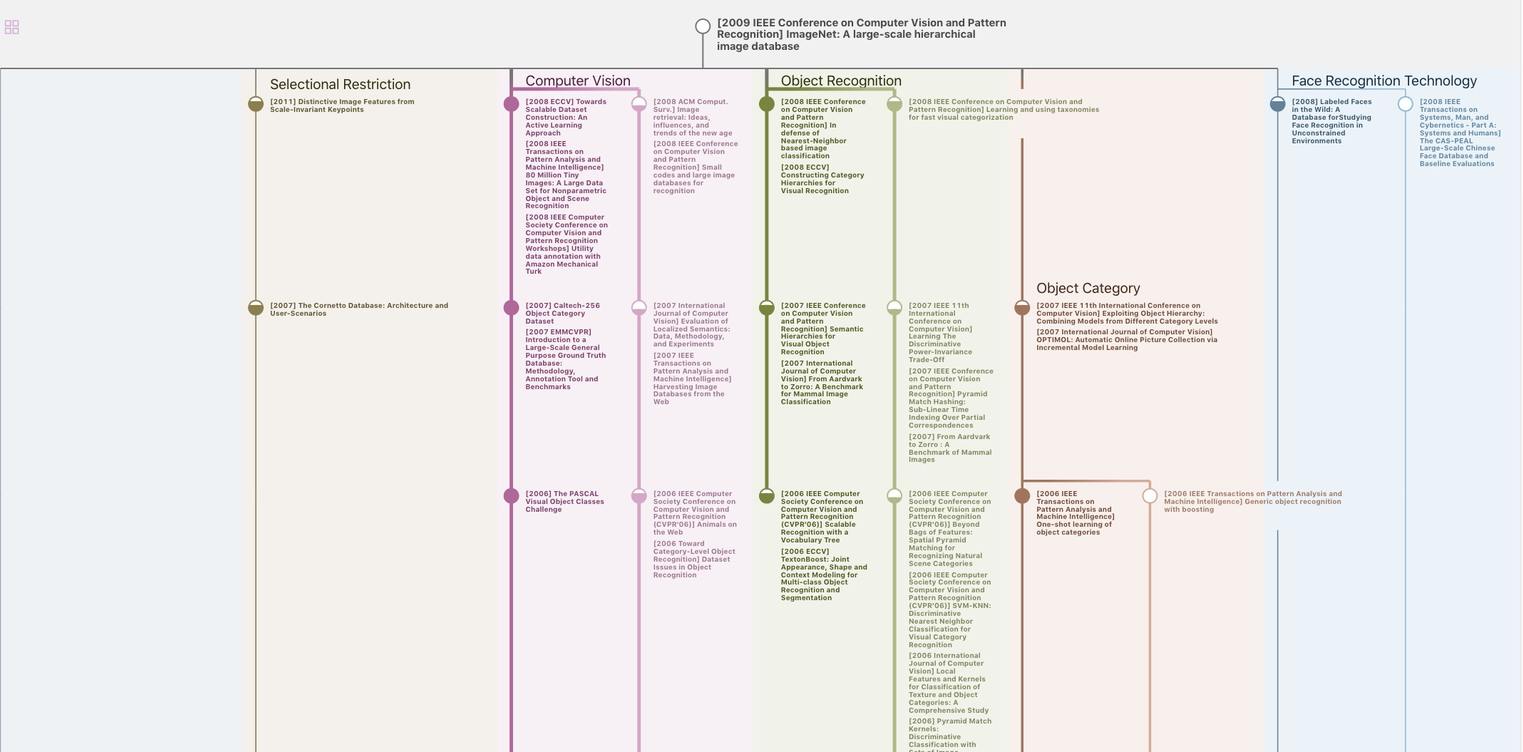
生成溯源树,研究论文发展脉络
Chat Paper
正在生成论文摘要