Could We Relieve AI/ML Models of the Responsibility of Providing Dependable Uncertainty Estimates? A Study on Outside-Model Uncertainty Estimates
COMPUTER SAFETY, RELIABILITY, AND SECURITY (SAFECOMP 2021)(2021)
摘要
Improvements in Artificial Intelligence (AI), especially in the area of neural networks, have led to calls to use them also in the context of safety-critical systems. However, current AI-based models are data-driven, so we cannot assure that they will provide the intended outcome for any input. To obtain information about the uncertainty remaining in their outcome, uncertainty estimation capabilities can be integrated during model building. However, the approach of providing accurate outcomes and dependable uncertainty estimates using the same model has limitations. Among others, estimates of such ‘in-model’ approaches are provided without statistical confidence, tend to be overconfident if not calibrated, and are hard to interpret and review by domain experts. An alternative ‘outside-model’ approach is the use of model-agnostic uncertainty wrappers (UWs). To investigate how well they perform in comparison to in-model approaches, we benchmarked them against deep ensembles, which can be considered the gold standard for in-model uncertainty estimation, as well as to the softmax outputs of a deep neural network as a baseline. Despite a slightly higher Brier score, the UW provides other benefits that are important in a safety-critical context, like considering a statistical confidence level and providing explainable uncertainty estimates through a decision tree considering human-interpretable semantic factors. Furthermore, in-model uncertainty estimates can be forwarded into an UW, combining advantages of both approaches.
更多查看译文
关键词
Uncertainty wrapper,Data-driven model,Machine learning,Benchmarking study,Traffic sign recognition,Automated driving,Deep ensemble,Uncertainty calibration,Uncertainty quantification
AI 理解论文
溯源树
样例
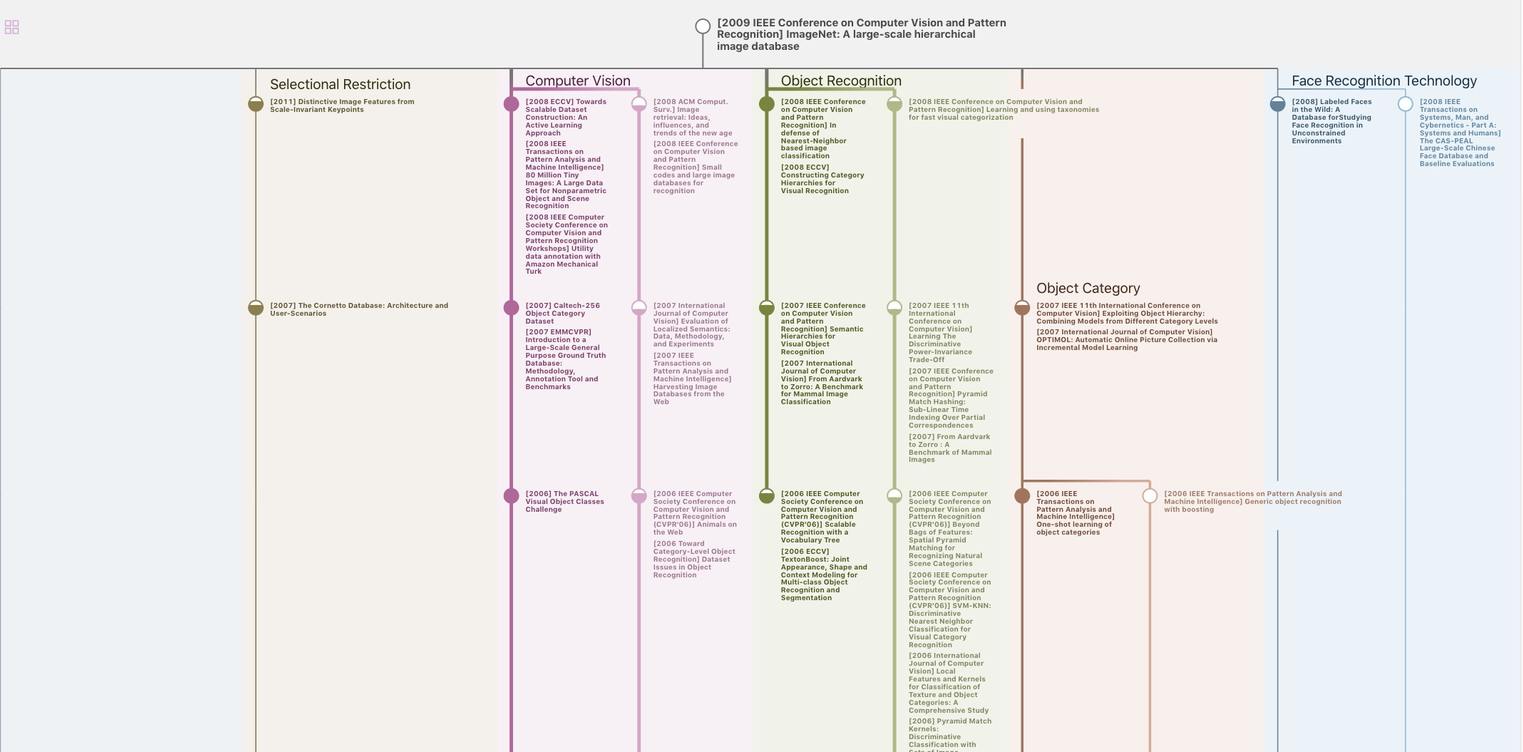
生成溯源树,研究论文发展脉络
Chat Paper
正在生成论文摘要