Leveraging Machine Learning To Advance Genome-Wide Association Studies
INTERNATIONAL JOURNAL OF DATA MINING AND BIOINFORMATICS(2021)
摘要
Genome-Wide Association Studies (GWAS) has demonstrated its power in discovering genetic variations to particular traits related to agronomically important features in crops. The typical output of a GWAS program includes a series of Single Nucleotide Polymorphisms (SNPs) and their significance. Currently, there is no standard way to compare results across different programs or to select the most 'significant' results uniformly and consistently. To obtain a comprehensive and accurate set of SNPs associated with a trait of interest, we present a novel automated pipeline that leverages machine learning for GWAS discoveries. The pipeline first performs population structure analysis, then executes multiple GWAS software and combines their results into a single SNP set. After that, it selects SNPs from the set with high individual and/or joint effects with the Least Absolute Shrinkage and Selection Operator analysis. Finally, the predictivity of the model is assessed using cross-validation.
更多查看译文
关键词
genome-wide association studies, machine learning, population structure analysis, cross-validation, LASSO, fusarium head blight
AI 理解论文
溯源树
样例
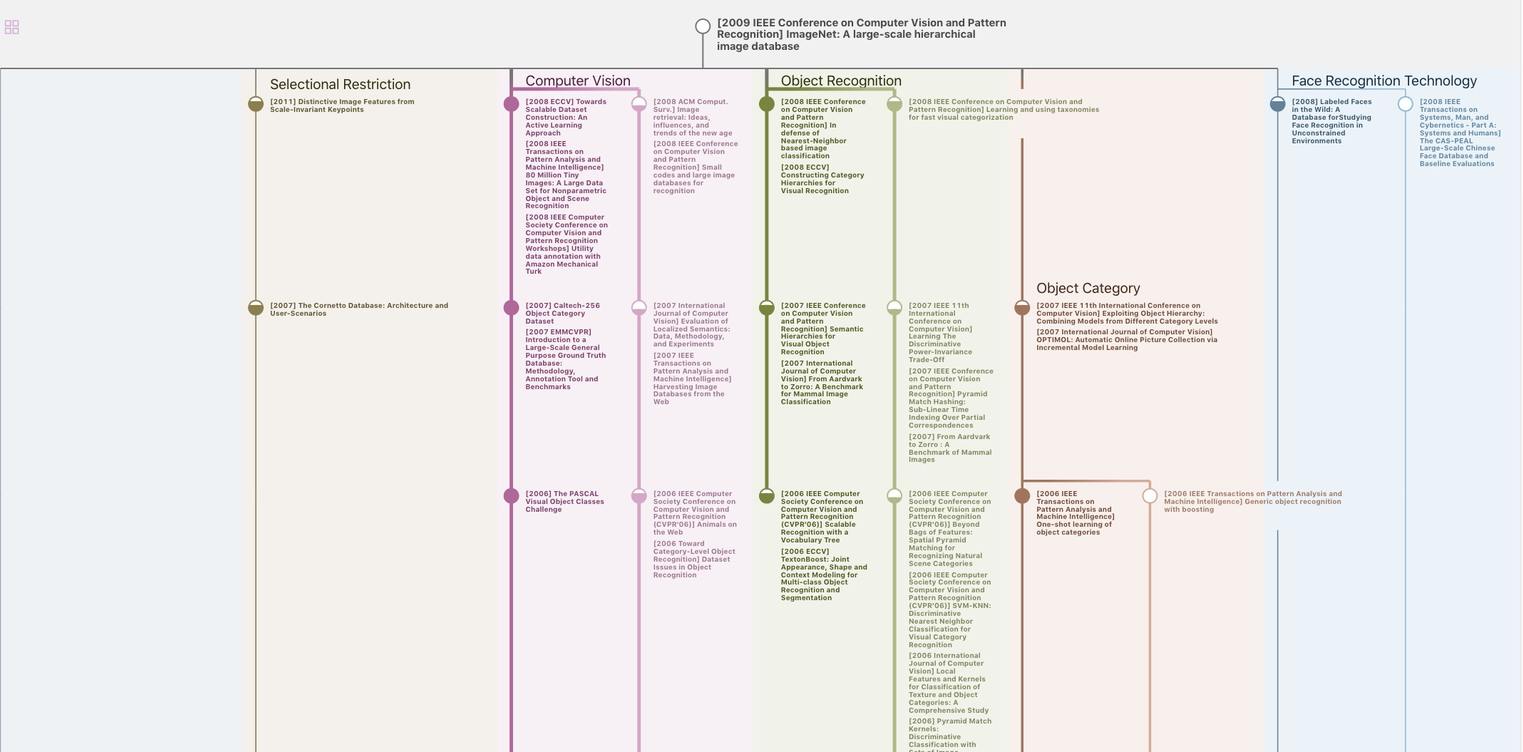
生成溯源树,研究论文发展脉络
Chat Paper
正在生成论文摘要